Restoration of Multiple Image Distortions using a Semi-dynamic Deep Neural Network
MM '23: Proceedings of the 31st ACM International Conference on Multimedia(2023)
摘要
Restoring multiple image distortions with a single model is difficult because different distortions require fundamentally different processing mechanisms, e.g., deblurring requires high-pass filtering, while denoising requires low-pass filtering operations. This paper presents a dynamic universal image restoration (DUIR) system capable of simultaneously processing multiple distortions. The new model features several innovative designs: (i) a distortion embedding module (DEM) to automatically encode the distortion information of an input, (ii) a distortion attention module (DAM) that uses a bi-directional long short-term memory (LSTM) to encode the distortion into a sequence of forward and backward interdependent modulating signals, and (iii) a dynamically adaptive image restoration deep convolutional neural network (DAIR-DCNN) featuring unique semi-dynamic layers (SDLs) in which part of their parameters are dynamically modulated by the distortion signals. DEM, DAM, and SDLs together make DAIR-DCNN adaptive to the distortions of the current input, which in turn equips the DUIR system with the capability of simultaneously processing multiple image distortions with a single trained model. We present extensive experimental results to show that the new technique achieves superior performance to state-of-the-art models on both synthetic and real data. We further demonstrate that a trained DUIR system can simultaneously handle different distortions, including those with conflicting demands, such as denoising, deblurring, and compression artifact removal.
更多查看译文
AI 理解论文
溯源树
样例
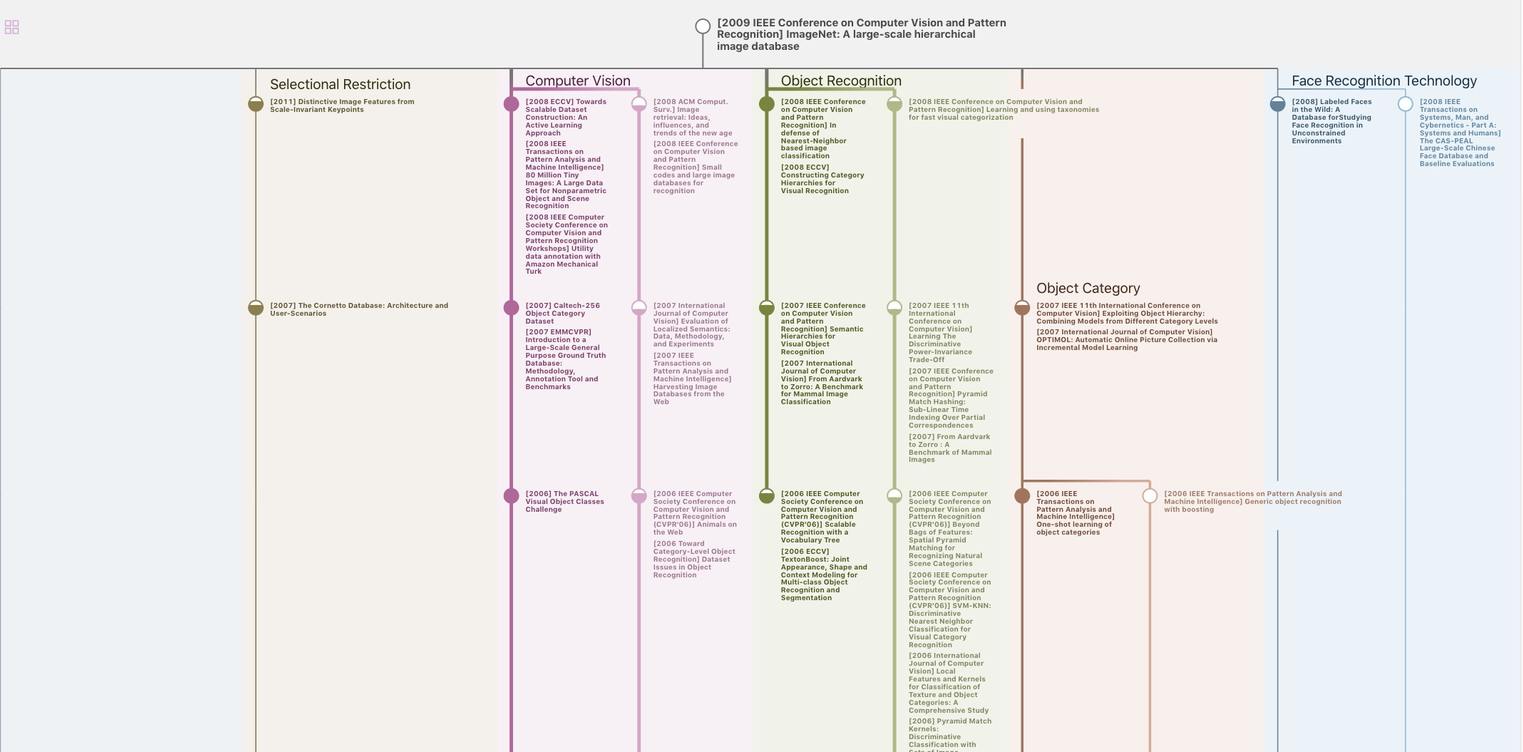
生成溯源树,研究论文发展脉络
Chat Paper
正在生成论文摘要