Video Question Answering: a Survey of Models and Datasets
MOBILE NETWORKS & APPLICATIONS(2021)
摘要
Video question answering (VideoQA) automatically answers natural language question according to the content of videos. It promotes the development of online education, scenario analysis, video content retrieving, etc. VideoQA is a challenging task because it requires a model to understand semantic information of the video and the question to generate the answer. Firstly, we propose a general framework of VideoQA which consists of a video feature extraction module, a text feature extraction module, an integration module, and an answer generation module. The integration module is the core module, including core processing model, recurrent neural networks (RNNs) encoder and feature fusion. These three sub-modules cooperate to generate the contextual representation, and the answer generation module generates the answer based on it. Then, we summarize the methods in core processing model, and introduce the ideas and applications of the methods in detail, such as encoder-decoder, attention model, and memory network and other methods. Additionally, we introduce the widely used datasets and evaluation criteria, as well as the analysis of experimental results on benchmark datasets. Finally, we discuss challenges in the field of VideoQA and provide some possible directions for future work.
更多查看译文
关键词
Video question answering, Feature extraction, Encoder-decoder, Attention model, Memory network, Recurrent neural networks, Feature fusion
AI 理解论文
溯源树
样例
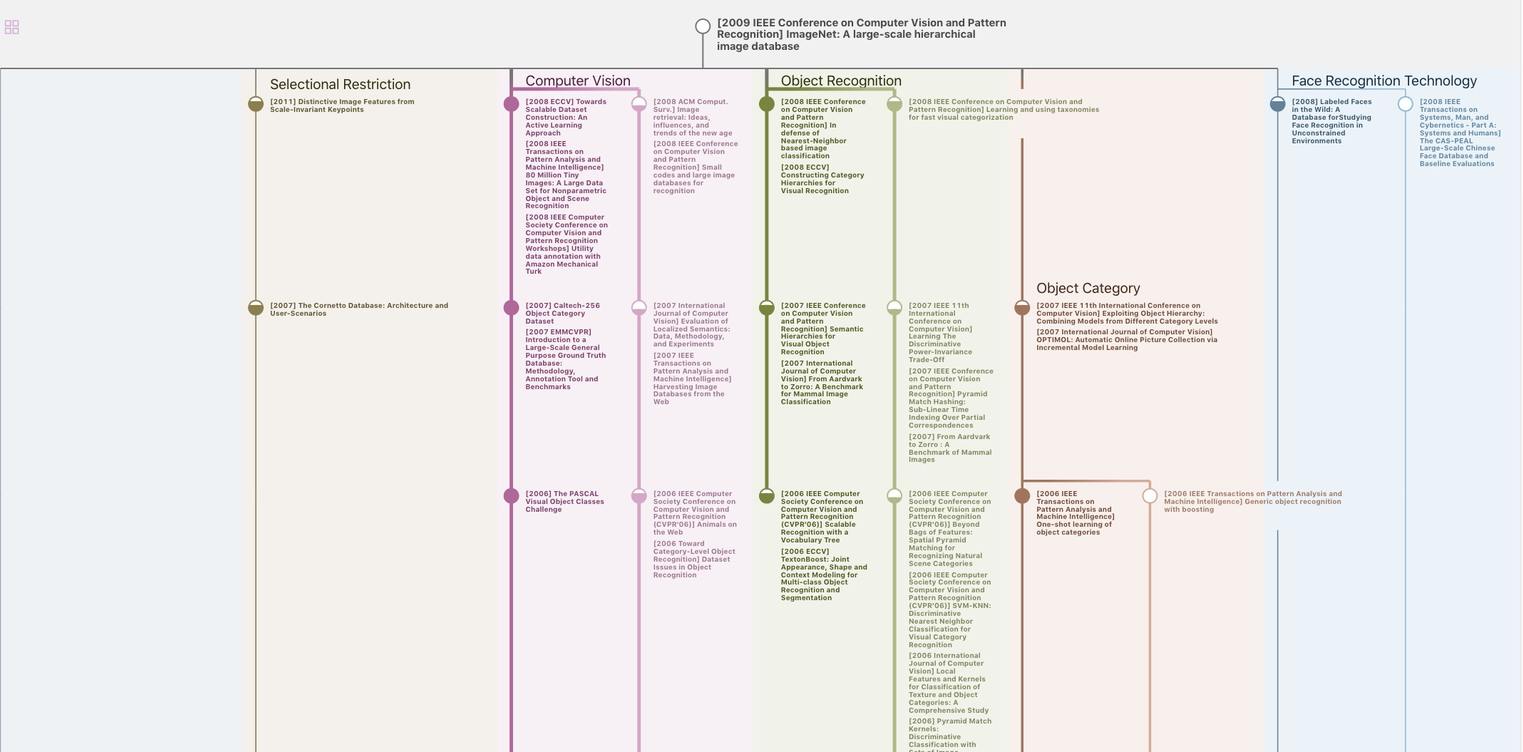
生成溯源树,研究论文发展脉络
Chat Paper
正在生成论文摘要