Classification Of Cardiac Abnormalities From Ecg Signals Using Se-Resnet
2020 COMPUTING IN CARDIOLOGY(2020)
Abstract
In PhysioNet/Computing in Cardiology Challenge 2020, we developed an ensembled model based on SE-ResNet to classify cardiac abnormalities from 12-lead electrocardiogram (ECG) signals. We employed two residual neural network modules with squeeze-and-excitation blocks to learn from the first 10-second and 30-second segments of the signals. We used external open-source data for validation and fine-tuning during the model development phase. We designed a multi-label loss to emphasize the impact of wrong predictions during training. We built a rule-based bradycardia model based on clinical knowledge to correct the output. All these efforts helped us to achieve a robust classification performance. Our final model achieved a challenge validation score of 0.682 and a full test score of 0.514, placing our team HeartBeats 3rd out of 41 in the official ranking. We believed that our model has a great potential to be applied in the actual clinical practice, and planned to further extend the research after the challenge.
MoreTranslated text
Key words
rule-based bradycardia model,cardiac abnormalities,ECG signals,SE-ResNet,12-lead electrocardiogram signals,residual neural network modules
AI Read Science
Must-Reading Tree
Example
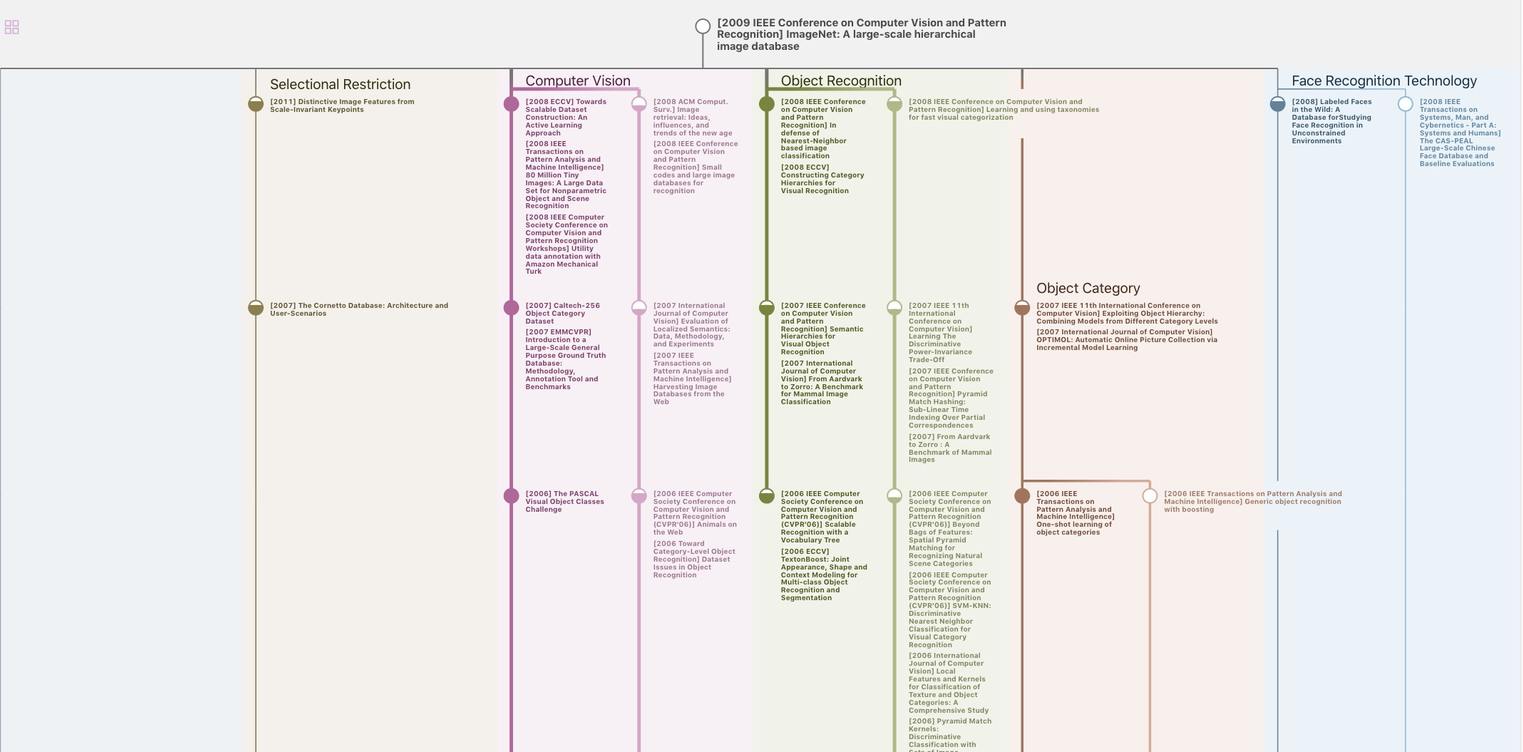
Generate MRT to find the research sequence of this paper
Chat Paper
Summary is being generated by the instructions you defined