Interactive Few-Shot Learning: Limited Supervision, Better Medical Image Segmentation
IEEE TRANSACTIONS ON MEDICAL IMAGING(2021)
摘要
Many known supervised deep learning methods for medical image segmentation suffer an expensive burden of data annotation for model training. Recently, few-shot segmentation methods were proposed to alleviate this burden, but such methods often showed poor adaptability to the target tasks. By prudently introducing interactive learning into the few-shot learning strategy, we develop a novel few-shot segmentation approach called Interactive Few-shot Learning (IFSL), which not only addresses the annotation burden of medical image segmentation models but also tackles the common issues of the known few-shot segmentation methods. First, we design a new few-shot segmentation structure, called Medical Prior-based Few-shot Learning Network (MPrNet), which uses only a few annotated samples (e.g., 10 samples) as support images to guide the segmentation of query images without any pretraining. Then, we propose an Interactive Learning-based Test Time Optimization Algorithm (IL-TTOA) to strengthen our MPrNet on the fly for the target task in an interactive fashion. To our best knowledge, our IFSL approach is the first to allow few-shot segmentation models to be optimized and strengthened on the target tasks in an interactive and controllable manner. Experiments on four few-shot segmentation tasks show that our IFSL approach outperforms the state-of-the-art methods by more than 20% in the DSC metric. Specifically, the interactive optimization algorithm (IL-TTOA) further contributes similar to 10% DSC improvement for the few-shot segmentation models.
更多查看译文
关键词
Medical image segmentation,few-shot learning,interactive learning,limited supervision
AI 理解论文
溯源树
样例
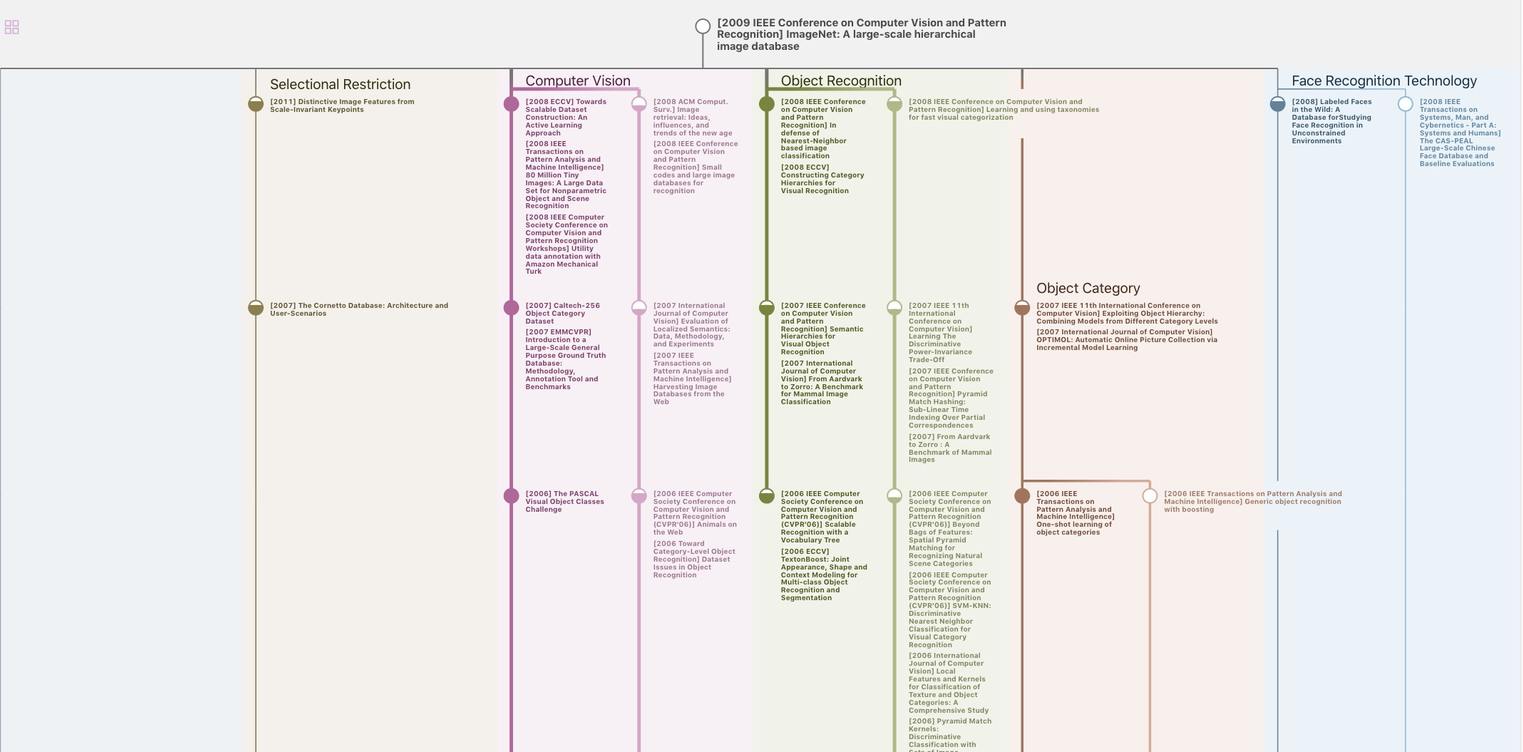
生成溯源树,研究论文发展脉络
Chat Paper
正在生成论文摘要