Toward Unsupervised Graph Neural Network: Interactive Clustering And Embedding Via Optimal Transport
20TH IEEE INTERNATIONAL CONFERENCE ON DATA MINING (ICDM 2020)(2020)
摘要
Most of the existing Graph Neural Networks (GNNs) are deliberately designed for semi-supervised learning tasks, where supervision information (labelled node) is utilized to mitigate the oversmoothing problem of message passing. Unfortunately, the oversmoothing problem tends to be more severe in unsupervised tasks, since supervision information is not available. Since community structure/cluster is an essential characteristic of network, a natural approach to reduce the oversmoothing problem is to also constrain the node embeddings to maintain their own characteristics to prevent all the node embeddings from becoming too similar to be distinguished. In this paper, a novel Optimal Transport based Graph Neural Network (OT-GNN) is proposed to overcome the oversmoothing problem in unsupervised GNNs by imposing the equal-sized clustering constraints to the obtained node embeddings. To solve the combinatorial optimization problem, the constrained objective function of unsupervised GNN is relaxed to an Optimal Transport problem, and a fast version of the Sinkhorm-Knopp algorithm is adopted to handle large networks. Extensive experiments on node clustering and classification demonstrate the superior performance of our proposed OT-GNN.
更多查看译文
关键词
graph neural network,network embedding,unsupervised learning,node clustering
AI 理解论文
溯源树
样例
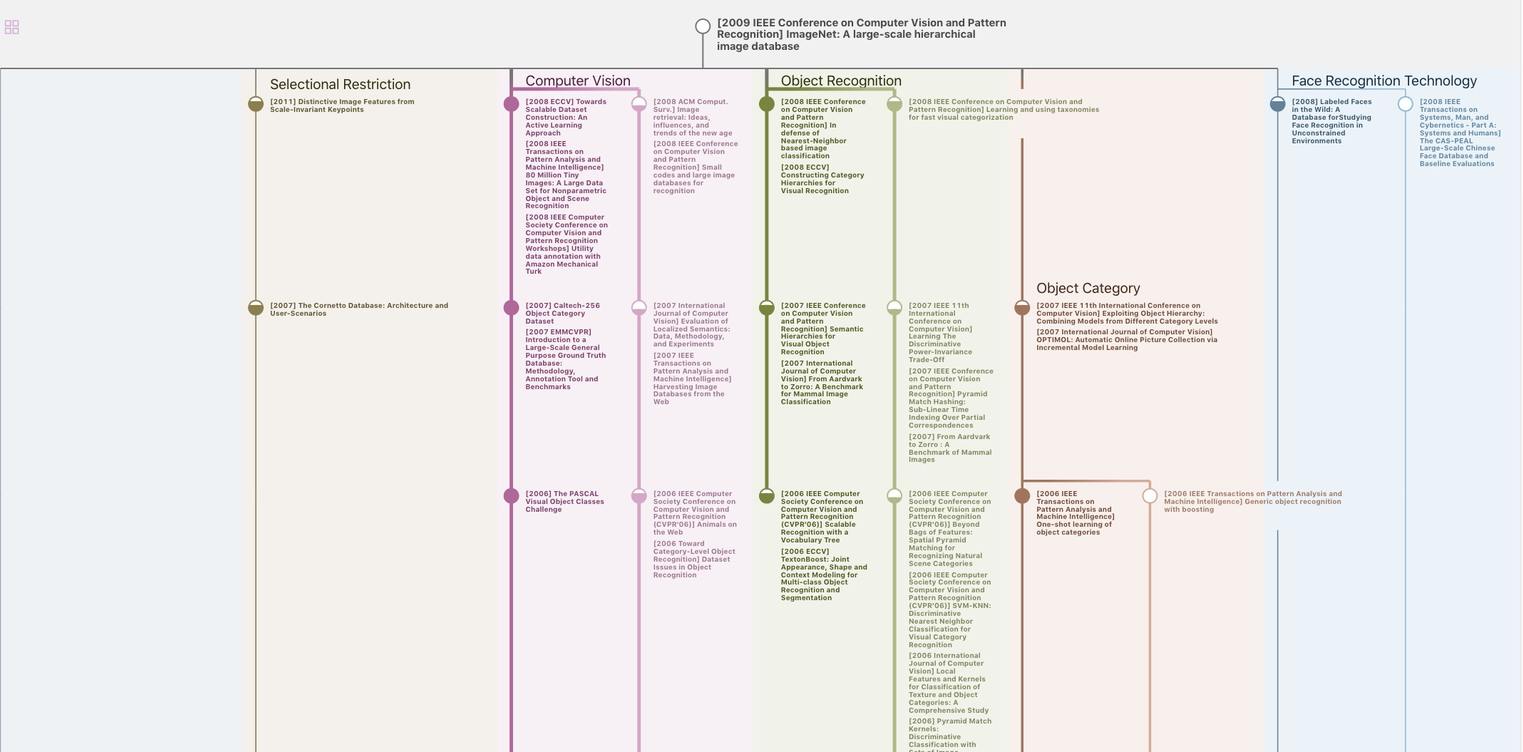
生成溯源树,研究论文发展脉络
Chat Paper
正在生成论文摘要