Conditional Distributional Treatment Effect with Kernel Conditional Mean Embeddings and U-Statistic Regression
INTERNATIONAL CONFERENCE ON MACHINE LEARNING, VOL 139(2021)
摘要
We propose to analyse the conditional distributional treatment effect (CoDiTE), which, in contrast to the more common conditional average treatment effect (CATE), is designed to encode a treatment's distributional aspects beyond the mean. We first introduce a formal definition of the CoDiTE associated with a distance function between probability measures. Then we discuss the CoDiTE associated with the maximum mean discrepancy via kernel conditional mean embeddings, which, coupled with a hypothesis test, tells us whether there is any conditional distributional effect of the treatment. Finally, we investigate what kind of conditional distributional effect the treatment has, both in an exploratory manner via the conditional witness function, and in a quantitative manner via U-statistic regression, generalising the CATE to higher-order moments. Experiments on synthetic, semi-synthetic and real datasets demonstrate the merits of our approach.
更多查看译文
关键词
Treatment Effects
AI 理解论文
溯源树
样例
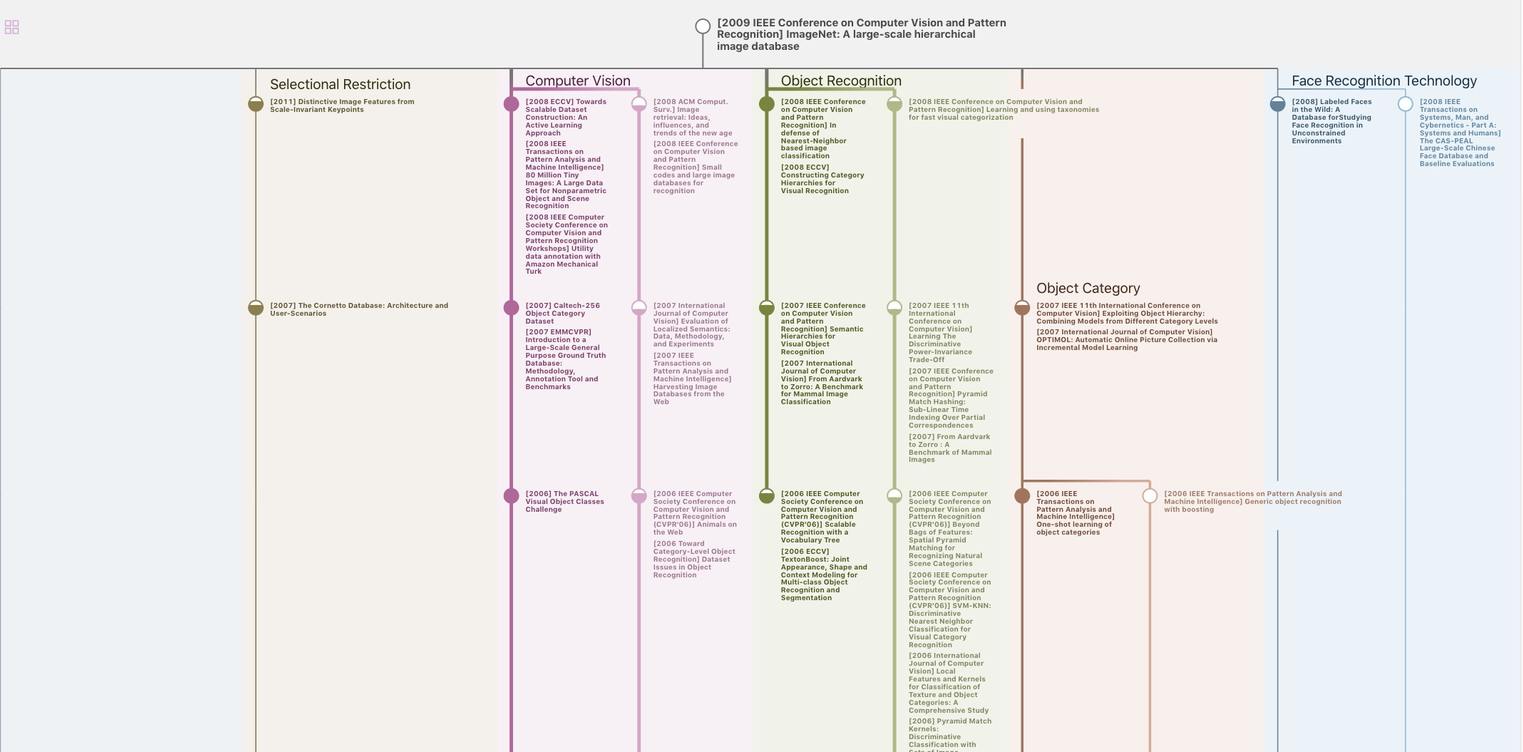
生成溯源树,研究论文发展脉络
Chat Paper
正在生成论文摘要