Leveraging Multi-view Learning for Human Anomaly Detection in Industrial Internet of Things
2020 International Conferences on Internet of Things (iThings) and IEEE Green Computing and Communications (GreenCom) and IEEE Cyber, Physical and Social Computing (CPSCom) and IEEE Smart Data (SmartData) and IEEE Congress on Cybermatics (Cybermatics)(2020)
Abstract
One of the most prominent human anomalous behaviour is fall. Fall detection for elderly care is important in the smart home care system. Fall detection is one of the smart home applications. In home-based assisted living, the detection of human anomalous behaviour is crucial. Home-based assisted living is becoming prevalent in elderly care. Aging brings several health issues and prevents the elderly to live an independent life. Therefore, it is important to detect human anomalous behaviour to ensure the safety of older adults. In this paper, we propose a multi-view learning approach to detect human anomalous behaviour. Our multi-view algorithms are running on edge devices. Multi-view learning is basically a method of learning from multiple views. It involves data fusion or integration from learned multiple feature sets. We evaluate our approaches for fall detection on the publicly available dataset “MobiFall_Dataset_v2.0”. We construct 2 distinct views of accelerometer and gyroscope data and apply co-training methods to train the classifiers. We perform experiments on the extracted feature sets of two different views and combined them using 3 different methods. We introduce 3 feature fusion methods to leverage the inadequacy of single-view learning. Multi-view approach used in this work has an improvement over single view learning and produces better accuracy in classification of falls.
MoreTranslated text
Key words
Fall Detection,Activity Recognition,Multi-view Learning,Sensor Fusion
AI Read Science
Must-Reading Tree
Example
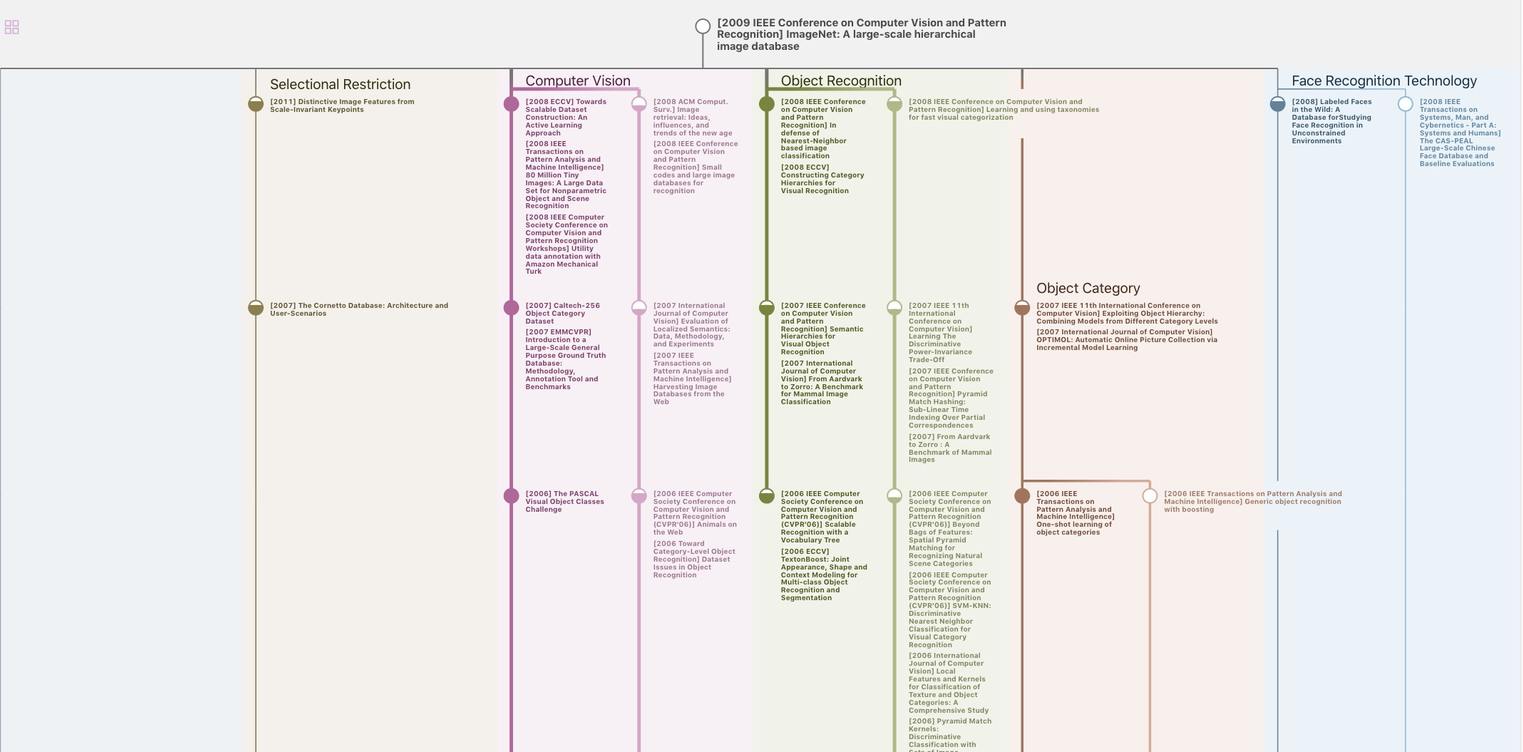
Generate MRT to find the research sequence of this paper
Chat Paper
Summary is being generated by the instructions you defined