Reinforcement Learning For Optimizing Driving Policies On Cruising Taxis Services
SUSTAINABILITY(2020)
摘要
As the key element of urban transportation, taxis services significantly provide convenience and comfort for residents' travel. However, the reality has not shown much efficiency. Previous researchers mainly aimed to optimize policies by order dispatch on ride-hailing services, which cannot be applied in cruising taxis services. This paper developed the reinforcement learning (RL) framework to optimize driving policies on cruising taxis services. Firstly, we formulated the drivers' behaviours as the Markov decision process (MDP) progress, considering the influences after taking action in the long run. The RL framework using dynamic programming and data expansion was employed to calculate the state-action value function. Following the value function, drivers can determine the best choice and then quantify the expected future reward at a particular state. By utilizing historic orders data in Chengdu, we analysed the function value's spatial distribution and demonstrated how the model could optimize the driving policies. Finally, the realistic simulation of the on-demand platform was built. Compared with other benchmark methods, the results verified that the new model performs better in increasing total revenue, answer rate and decreasing waiting time, with the relative percentages of 4.8%, 6.2% and -27.27% at most.
更多查看译文
关键词
Markov decision process,reinforcement learning,optimizing driving policies,cruising taxis services
AI 理解论文
溯源树
样例
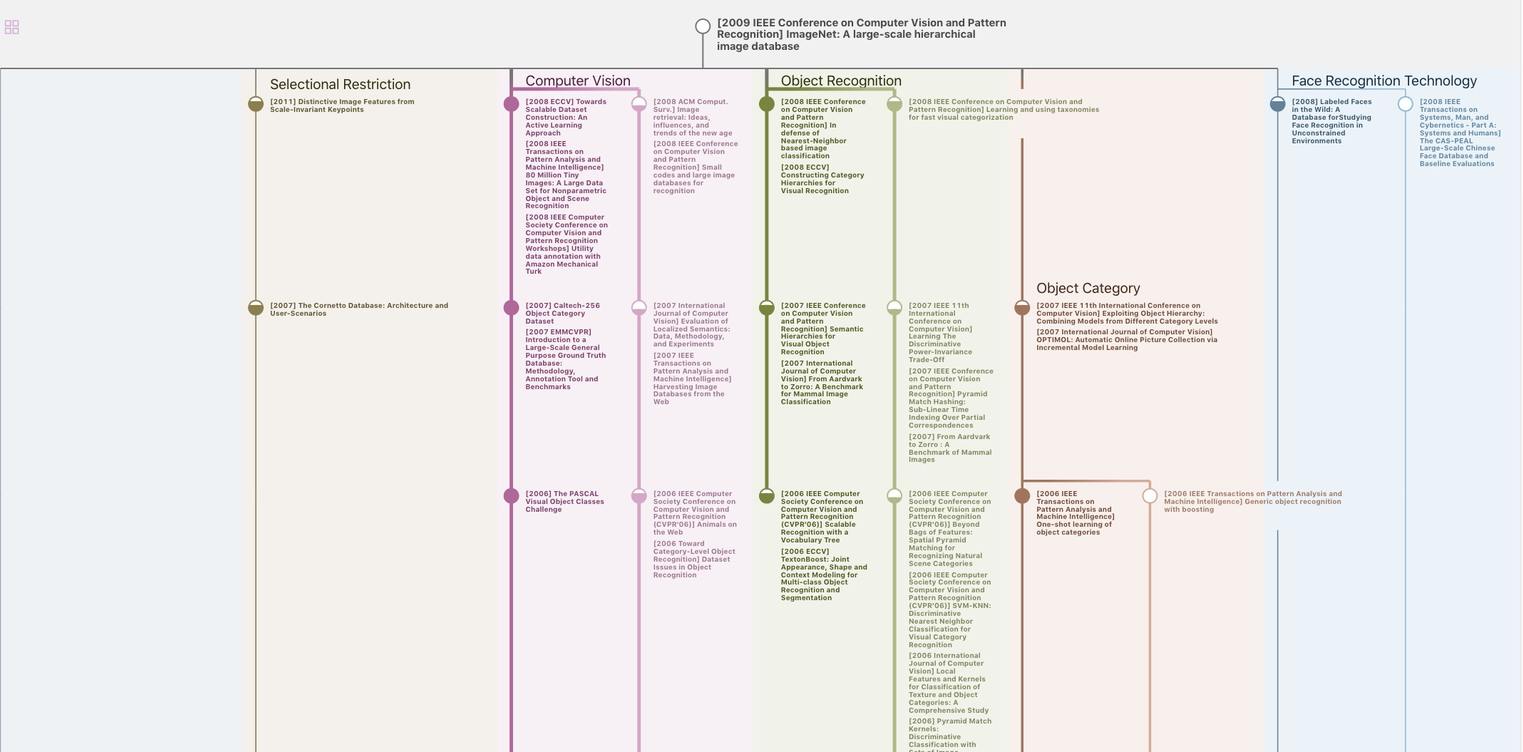
生成溯源树,研究论文发展脉络
Chat Paper
正在生成论文摘要