Using Wearable Sensors for Human Activity Recognition in Logistics: A Comparison of Different Feature Sets and Machine Learning Algorithms
INTERNATIONAL JOURNAL OF ADVANCED COMPUTER SCIENCE AND APPLICATIONS(2020)
摘要
The topic of human activity recognition has gained a lot of attention due to its usage for exercise monitoring, smart health and assisted living.Even though the aforementioned domains have received significant interest by researchers, activity recognition for industrial settings has received little attention in comparison.Industry 4.0 involves the assimilation of industrial workers with robots and other equipment used in the industry and necessitates the development of recognition methodologies for activities being performed in industries.In this regard, this paper presents a comparison in performance of various time/frequency domain features and popular machine learning algorithms for use in activity recognition in a logistics scenario.Experiments were conducted on inertial measurement sensor data from the recently released LARa dataset which involved three feature sets being used with four machine learning algorithms; Support Vector Machines, Decision Trees, Random Forests and Extreme Gradient Boost (XGBoost).The best result achieved in the experiments was an average accuracy of 78.61% using the XGBoost classifier while using both time and frequency domain features.This work serves as a baseline for activity recognition in logistics using IMU sensors and enables the development of solutions to support fulfillment of Industry 4.0 goals.
更多查看译文
关键词
Human Activity Recognition (HAR),inertial sensors,LARa dataset,smart industry
AI 理解论文
溯源树
样例
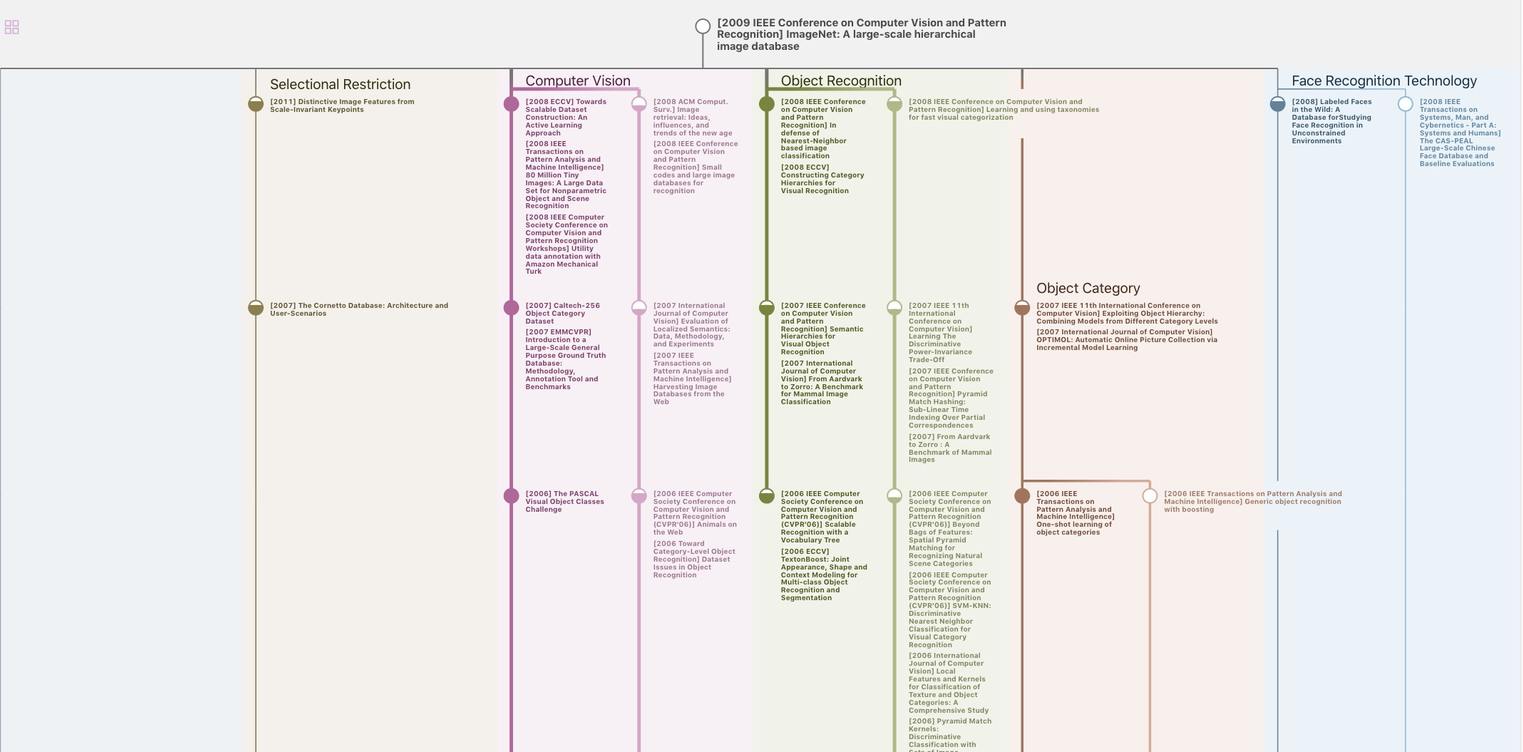
生成溯源树,研究论文发展脉络
Chat Paper
正在生成论文摘要