Self-supervised Contrastive Few-Shot Learning for Motor Imagery Brain-Computer Interfaces.
ICCPR '23 Proceedings of the 2023 12th International Conference on Computing and Pattern Recognition(2024)
摘要
Motor imagery brain-computer interfaces (BCIs) are integral to the field of intelligent rehabilitation. However, the collection of motor imagery data is time-consuming and expensive, while traditional deep learning models often face the risk of overfitting when handling limited data samples. In this paper, we propose a motor imagery recognition network that merges a self-supervised contrastive framework with few-shot learning. The proposed model is first trained on the dataset without labels using self-supervised contrastive to obtain the optimal model. The model parameters obtained are then used as initialization parameters for the prototypical network, which improves in few-shot learning. The results show that on the BCICIV2A and BCICIV2B datasets, the proposed approach achieves accuracies of 69.12% and 80.27%, respectively, representing improvements of 6.79% and 4.14% compared to the baseline models. This research achievement holds significant implications for advancing the application of motor imagery BCIs in the domain of intelligent rehabilitation.
更多查看译文
AI 理解论文
溯源树
样例
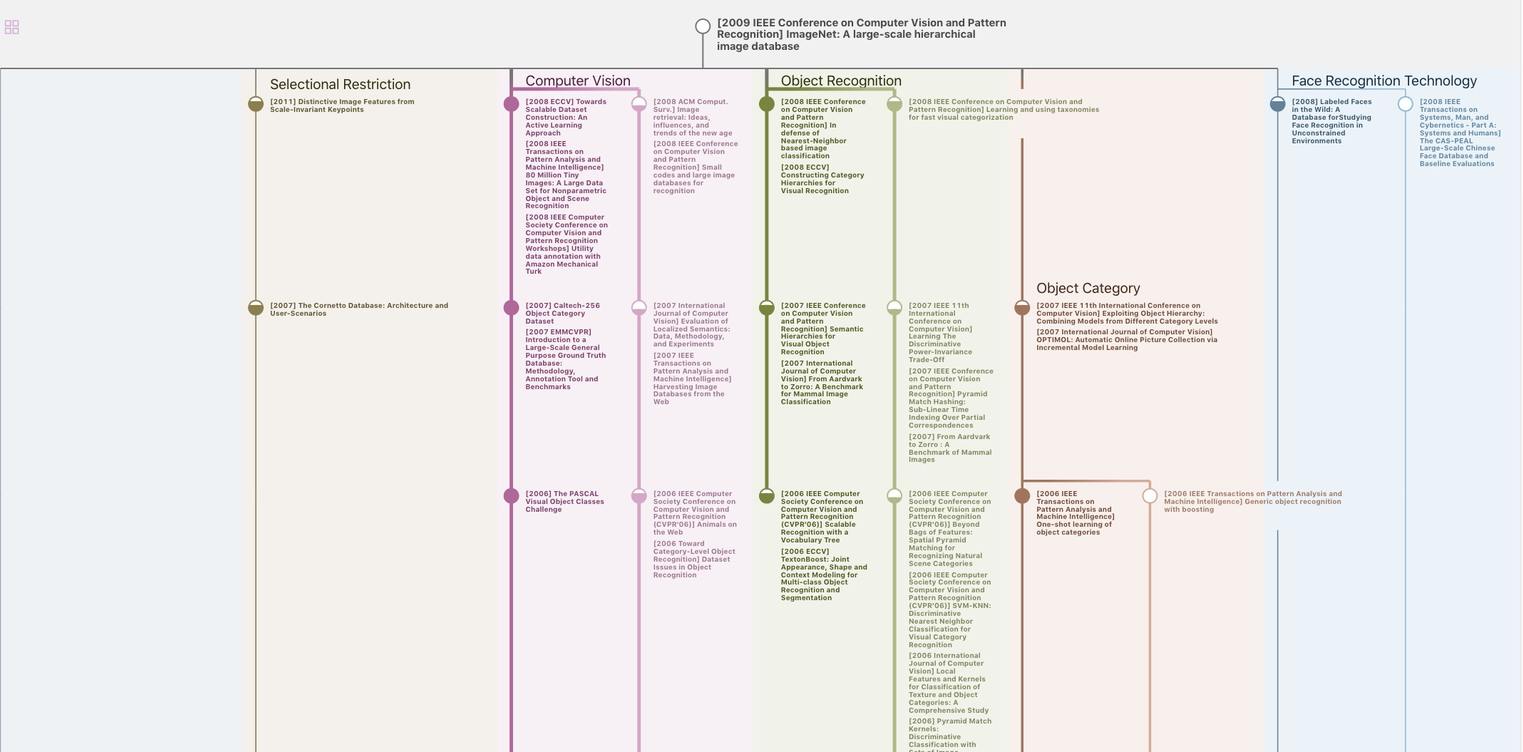
生成溯源树,研究论文发展脉络
Chat Paper
正在生成论文摘要