Geodesic distances in the intrinsic dimensionality estimation using packing numbers
NONLINEAR ANALYSIS-MODELLING AND CONTROL(2014)
Abstract
Dimensionality reduction is a very important tool in data mining. An intrinsic dimensionality of a data set is a key parameter in many dimensionality reduction algorithms. When the intrinsic dimensionality of a data set is known, it is possible to reduce the dimensionality of the data without losing much information. To this end, it is reasonable to find out the intrinsic dimensionality of the data. In this paper, one of the global estimators of intrinsic dimensionality, the packing numbers estimator (PNE), is explored experimentally. We propose the modification of the PNE method that uses geodesic distances in order to improve the estimates of the intrinsic dimensionality by the PNE method.
MoreTranslated text
Key words
multidimensional data,intrinsic dimensionality,packing numbers estimator,manifold,degrees of freedom,image understanding,motion
AI Read Science
Must-Reading Tree
Example
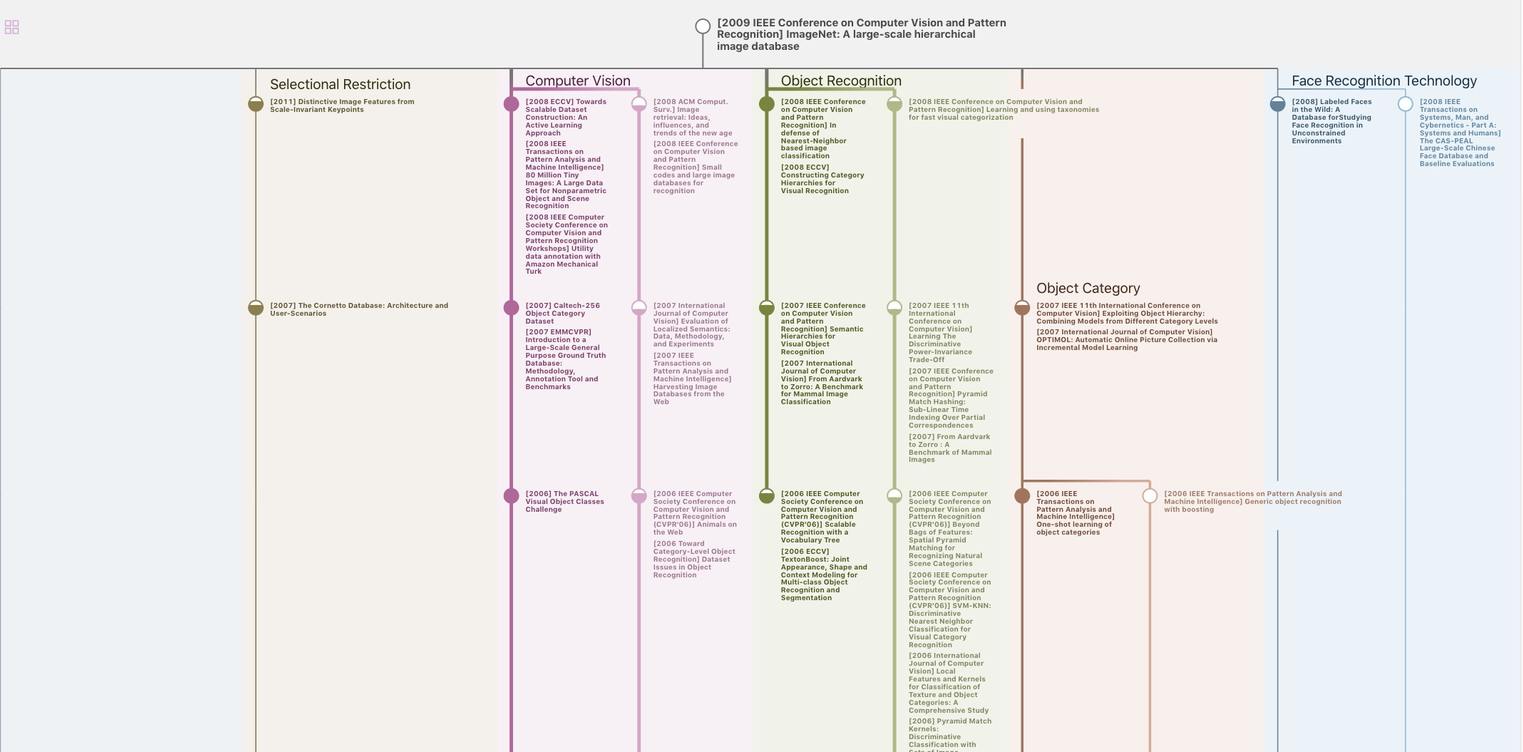
Generate MRT to find the research sequence of this paper
Chat Paper
Summary is being generated by the instructions you defined