Predictive analytics approaches for software effort estimation: A review
Indian journal of science and technology(2020)
Abstract
Background/Objective: In Software Effort Estimation (SEE), predicting the amount of time taken in human hours or months for software development is considered as a cumbersome process. SEE consists of both Software Development Effort Estimation (SDEE) and Software Maintenance Effort Estimation (SMEE). Over estimation or under estimation of software effort results in project cancellation or project failure. The objective of this study is to identify the best performing model for software Effort Estimation through experimental comparison with various Machine learning algorithms. Methods: Software Effort Estimation was addressed by using various machine learning techniques such as Multilinear Regression, Ridge Regression, Lasso Regression, ElasticNet Regression, Random Forest, Support Vector Machine, Decision Tree and NeuralNet to recognize best performing model. Datasets used are Desharnais, Maxwell, China and Albrecht datasets. Evaluation metrics considered are Mean Absolute Error (MAE), Mean Squared Error (MSE), Root Mean Square Error (RMSE) and R-Squared. Findings: Experiments on various machine learning algorithms for software Effort Estimation determines that Support Vector Machine produced the best performance comparatively with other algorithms. Keywords: Machine learning; software effort estimation; regression models; classification models
MoreTranslated text
Key words
software effort estimation,predictive analytics approaches
AI Read Science
Must-Reading Tree
Example
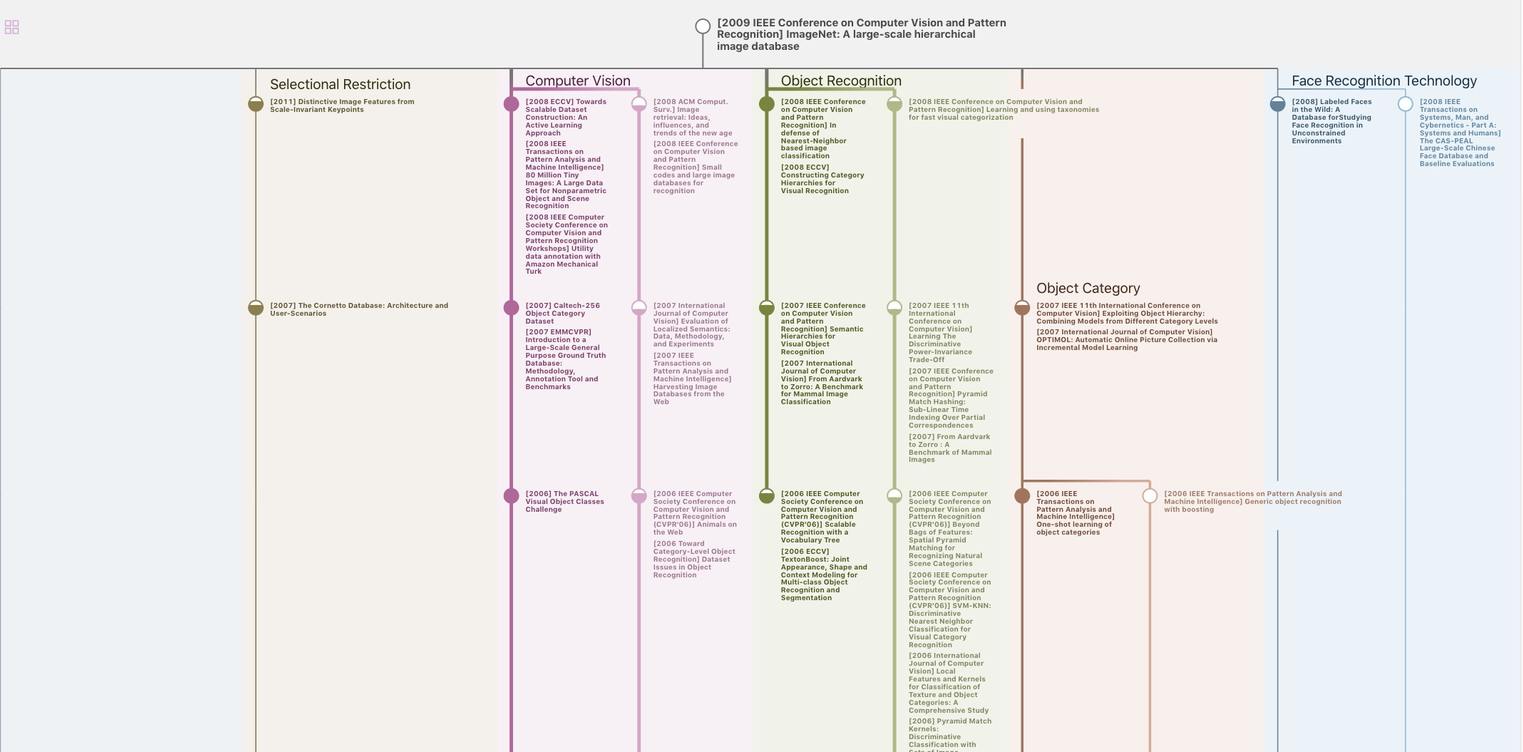
Generate MRT to find the research sequence of this paper
Chat Paper
Summary is being generated by the instructions you defined