CAN A DEEP CONVOLUTIONAL NEURAL NETWORK EXTRACT DIAGNOSTIC INFORMATION ON OBSTRUCTIVE SLEEP APNEA FROM IMAGES?
Sleep(2020)
摘要
Abstract Introduction Lateral cephalometric radiography is a simple way to provide craniofacial soft/hard tissue profiles specific for patients with obstructive sleep apnea (OSA) and may thus offer diagnostic information on the disease. We hypothesized that a machine learning technology, a deep convolutional neural network (DCNN), could make it possible to detect OSA based solely on lateral cephalometric radiographs without the need for either large amounts of subjective/laboratory data or skilled analyses. Methods In this diagnostic study, a DCNN was developed (n=1,258) and tested (n=131) using data from 1,389 lateral cephalometric radiographs obtained from individuals diagnosed with severe OSA (n=867; apnea hypopnea index >30/hour) or non-OSA (n=522; apnea hypopnea index < 5) at a single center for sleep disorders from March, 2006 to February, 2017. Three kinds of data sets were prepared by changing the area of interest using a single image; original image without any modification (Full Image), image containing a facial profile, upper airway, craniofacial soft/hard tissues, and image containing part of the occipital region (upper left corner of the image; Head Only). A radiologist and an orthodontist also performed a manual cephalometric analysis of the Full Image for comparison. Observers were blinded to the patient groupings. Data analysis was performed from April, 2018 to August, 2019. When the predictive score obtained from the DCNN analysis exceeded the threshold (0.50), the patient was judged to have OSA. The primary outcome was diagnostic accuracy in terms of area under the receiver-operating characteristic curve. Results The sensitivity/specificity was 0.87/0.82 for Full Image, 0.88/0.75 for Main Region, 0.71/0.63 for Head Only, and 0.54/0.80 for the manual analysis. The area under the curve was the highest for Main Region (0.92): 0.89 for Full Image, 0.70 for Head Only, and 0.75 for the manual analysis. Conclusion A DCNN identified individuals with OSA with high accuracy. This is a useful approach that does not require any laborious analyses in a primary care setting or in remote areas where an initial specialized OSA diagnosis is not feasible. Support This study was supported in part by the Japan Society for the Promotion of Science (grant numbers 17K11793, 19K10236).
更多查看译文
关键词
obstructive sleep apnea,neural network
AI 理解论文
溯源树
样例
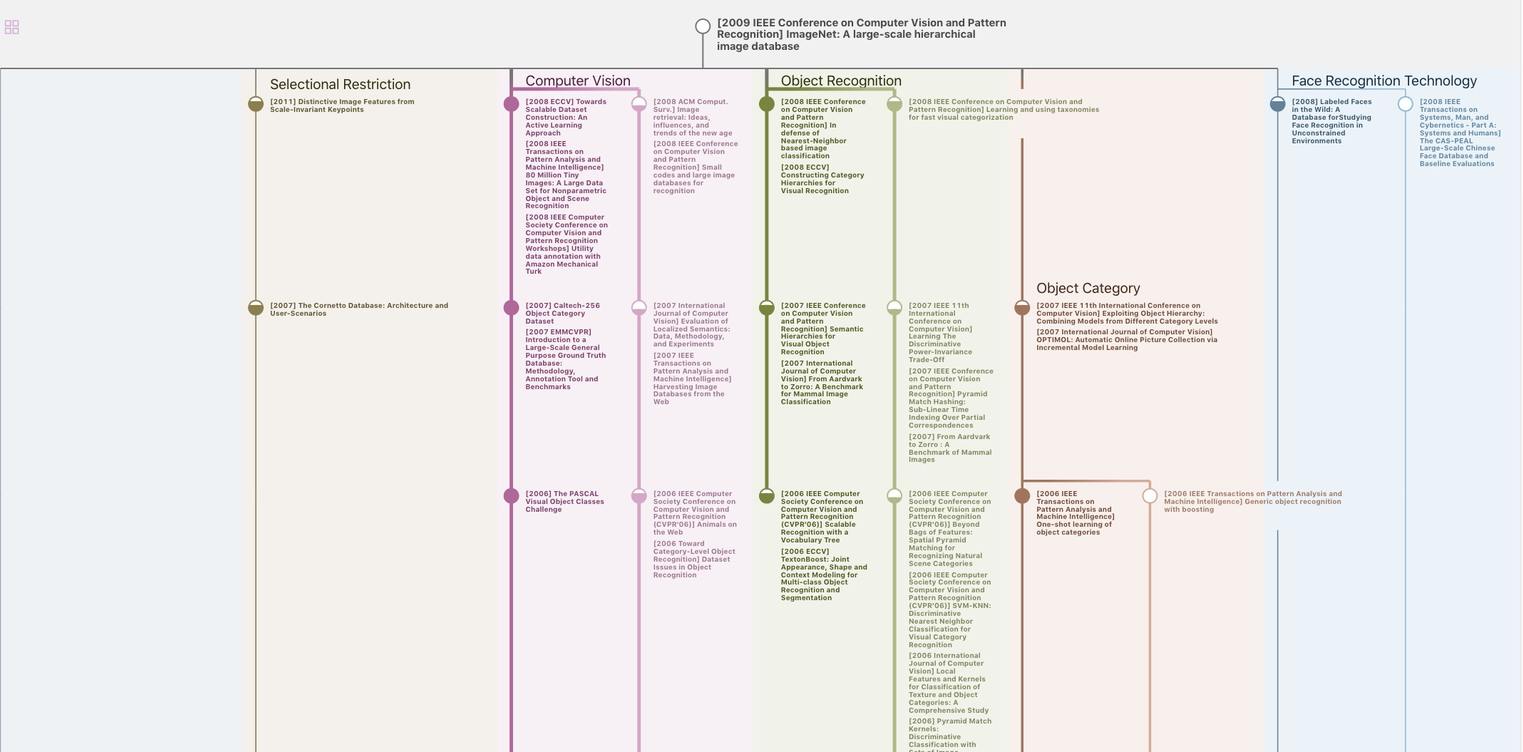
生成溯源树,研究论文发展脉络
Chat Paper
正在生成论文摘要