Tighter continuous relaxations for MAP inference in discrete MRFs: A survey
Handbook of Numerical Analysis(2019)
摘要
Continuous relaxations are central to MAP inference in discrete Markov random fields (MRFs). In these methods, the intractable discrete optimization problem is approximated by a continuous relaxation. The relaxation could be based on different approaches, with the linear programming (LP) relaxation being the most well studied. For continuous relaxations, the important considerations are efficiently solving the optimization formulations and improving the approximation quality of the relaxations. In this chapter, we focus on the latter topic, which is referred to as tighter continuous relaxations. We present a comprehensive survey of techniques to achieve a tighter LP relaxation, followed by a discussion of semidefinite programming (SDP) relaxation techniques. We discuss these topics by covering both theoretical motivations and algorithmic considerations. We hope this will be a ready reference for researchers …
更多查看译文
关键词
Graphical models, Discrete Markov random fields, MAP inference, Discrete and continuous optimization, Continuous relaxation, Relaxation hierarchies
AI 理解论文
溯源树
样例
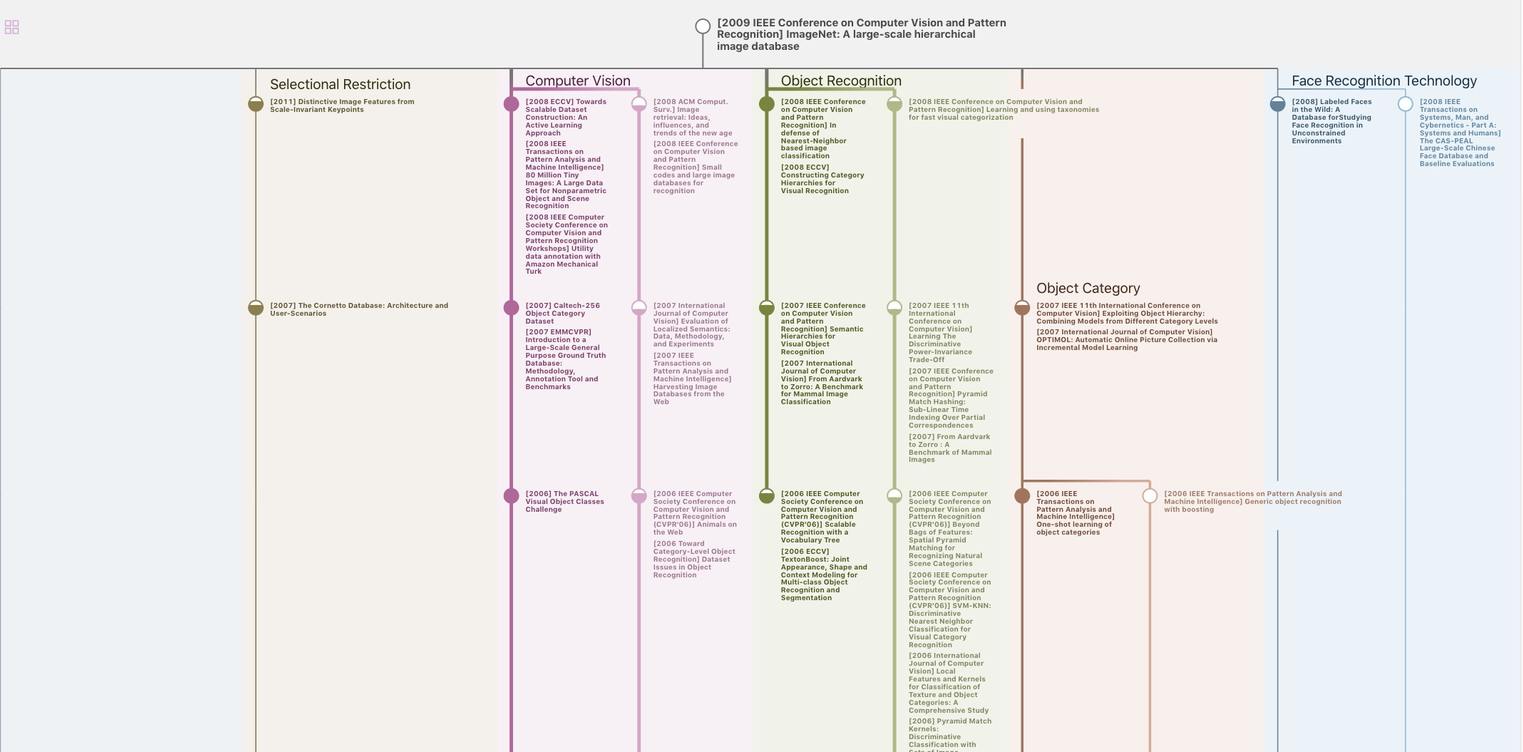
生成溯源树,研究论文发展脉络
Chat Paper
正在生成论文摘要