Revealing The State Space Of Turbulence Using Machine Learning
PHYSICAL REVIEW FLUIDS(2021)
摘要
Despite the apparent complexity of turbulent flow, identifying a simpler description of the underlying dynamical system remains a fundamental challenge. Capturing how the turbulent flow meanders among unstable states (simple invariant solutions) in phase space, as envisaged by Hopf [Commun. Pure Appl. Math. 1, 303 (1948)], using some efficient representation offers the best hope of doing this, despite the inherent difficulty in identifying these states. Here, we make a significant step toward this goal by demonstrating that deep convolutional autoencoders can identify low-dimensional representations of two-dimensional turbulence which are closely associated with the simple invariant solutions characterizing the turbulent attractor. To establish this, we develop latent Fourier analysis that decomposes the flow embedding into a set of orthogonal latent Fourier modes which decode into physically meaningful patterns resembling simple invariant solutions. The utility of this approach is highlighted by analyzing turbulent Kolmogorov flow (monochromatically forced flow on a 2D torus) at Re = 40, where, in between intermittent bursts, the flow resides in the neighborhood of an unstable state and is very low dimensional. Projections onto individual latent Fourier wavenumbers reveal the simple invariant solutions organizing both the quiescent and bursting dynamics in a systematic way inaccessible to previous approaches.
更多查看译文
关键词
Coherent Structures,Deep Learning,Turbulent Flows,Model Reduction,Hydrodynamic Turbulence
AI 理解论文
溯源树
样例
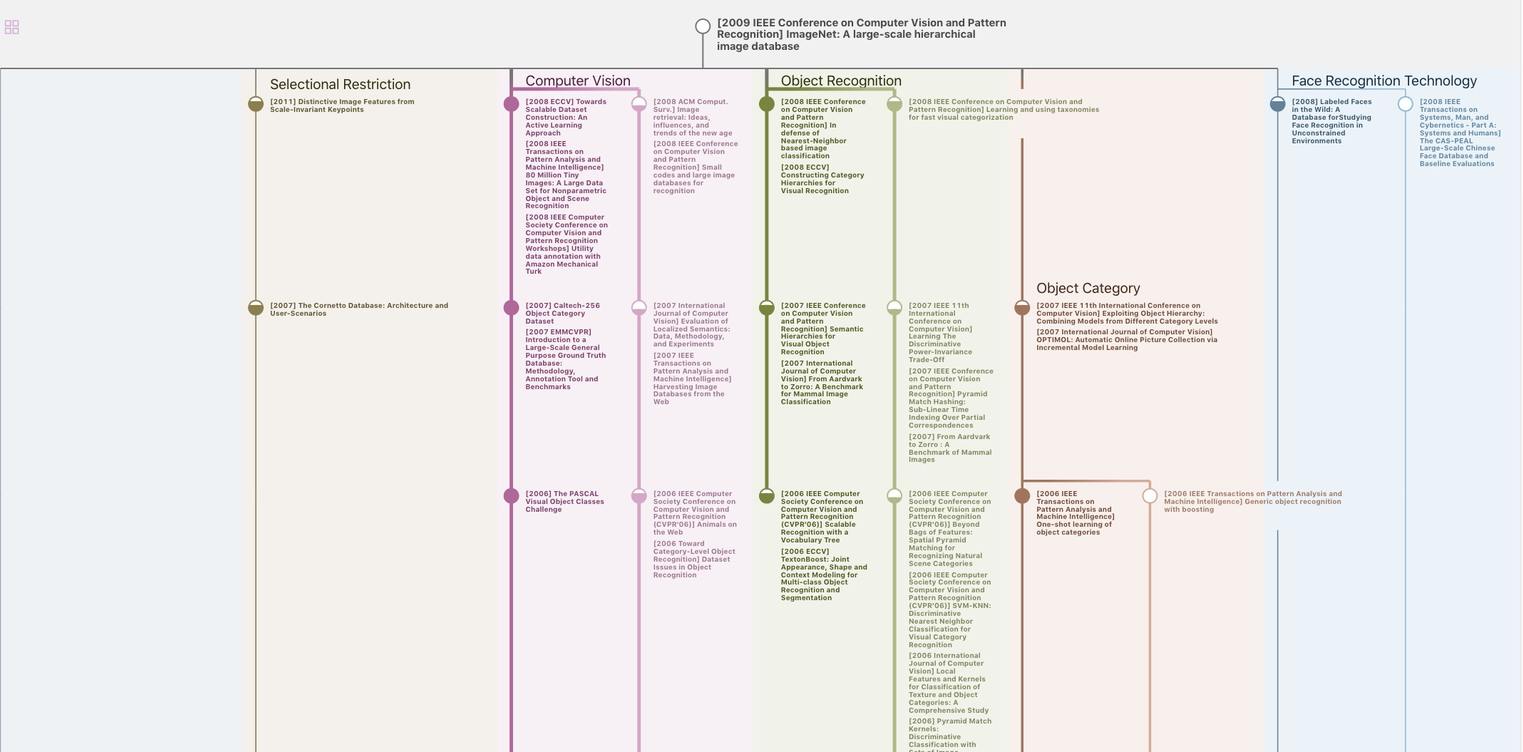
生成溯源树,研究论文发展脉络
Chat Paper
正在生成论文摘要