Enhanced clustering methodology
semanticscholar(2012)
摘要
Clustering in data analysis means data with similar features are grouped together within a particular valid cluster. Each cluster consists of data that are more similar among themselves and dissimilar to data of other clusters. Clustering can be viewed as an unsupervised learning concept from machine learning perspective. In this paper, we have proposed an Enhanced Clustering Methodology to obtain better clustering quality with much reduced complexity. We have evaluated the performances of the classical K-Means approach of data clustering, it’s modified Global K-Means, an Efficient K-Means and the proposed Enhanced K-Means method. The accuracy of all these algorithms were examined taking several data sets from UCI [21] repository of machine learning databases. Their clustering efficiency has been compared in conjunction with two typical cluster validity indices, namely the Davies-Bouldin Index and the Dunn’s Index for different number of clusters, and our experimental results demonstrated that the quality of clustering by proposed method is much proficient than the other mentioned K-Means based algorithms when larger data sets with more number of attributes are taken into consideration. Apart from this it has been found that, the computational time for clustering determined by the proposed algorithm is much lower than the other discussed methods.
更多查看译文
AI 理解论文
溯源树
样例
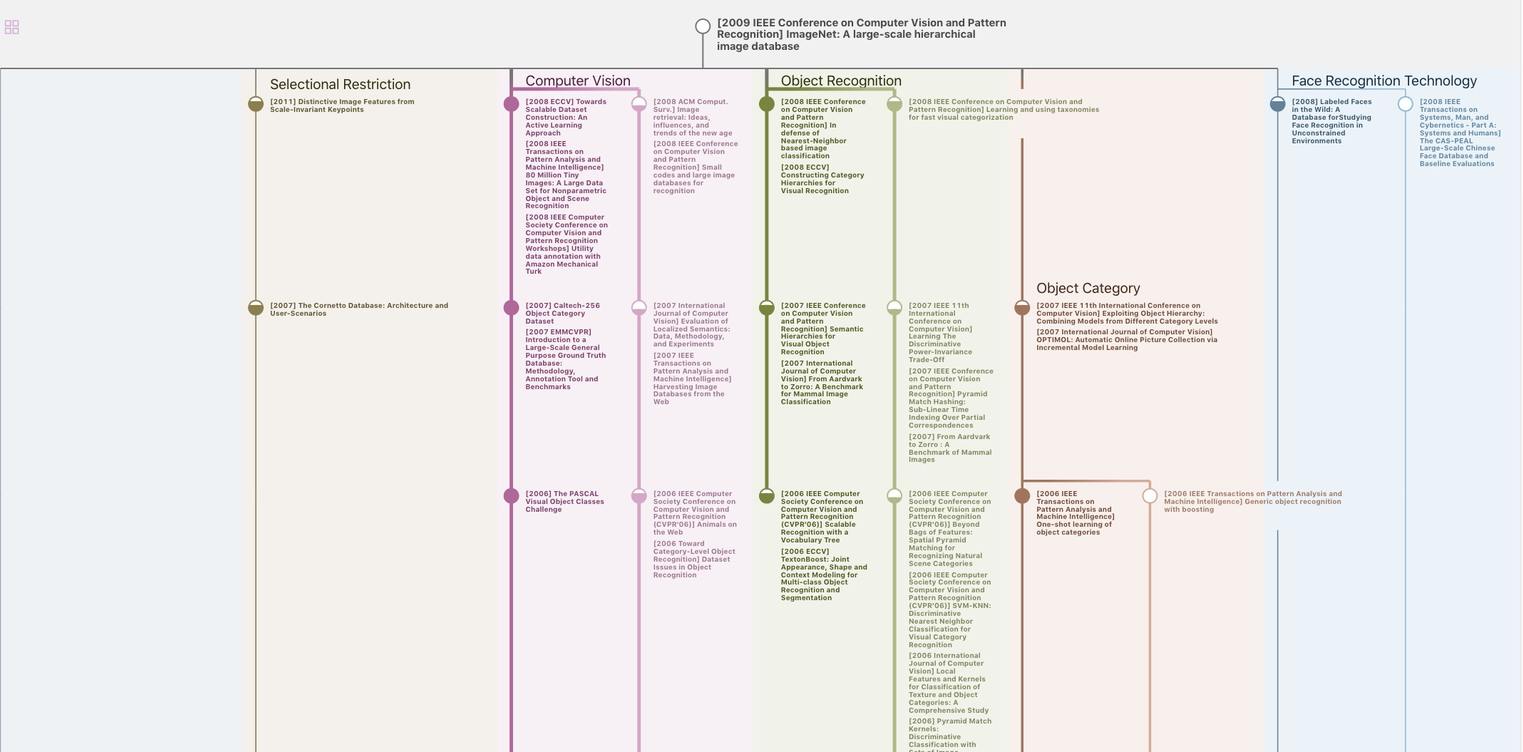
生成溯源树,研究论文发展脉络
Chat Paper
正在生成论文摘要