Surprise Sampling: Improving And Extending The Local Case-Control Sampling
ELECTRONIC JOURNAL OF STATISTICS(2021)
Abstract
Fithian & Hastie [7] proposed a sampling scheme called local case-control (LCC) sampling that achieves stability and efficiency by utilizing a clever adjustment pertained to the logistic model. It is particularly useful for classification with large and imbalanced data. This paper proposes a more general sampling scheme based on a working principle that data points deserve higher sampling probability if they contain more information or appear "surprising" in the sense of, for example, a large error of pilot prediction or a large absolute score. Compared with the relevant existing sampling schemes, as reported in [7] and [1], the proposed one has several advantages. It adaptively gives out the optimal forms to a variety of objectives, including the LCC and [1] as special cases. Under same model specifications, the proposed estimator also performs no worse than those in the literature. The estimation procedure is valid even if the model is misspecified and/or the pilot estimator is inconsistent or dependent on full data. We present theoretical justifications of the claimed advantages and optimality of the estimation and the sampling design. Different from [1], our large sample theory are population-wise rather than data-wise. Moreover, the proposed approach can be applied to unsupervised learning studies, since it essentially only requires a specific loss function and no response-covariate structure of data is needed. Numerical studies are carried out and the evidence in support of the theory is shown.
MoreTranslated text
Key words
Generalized linear models, Horvitz-Thompson estimator, local case-control sampling, model mis-specification, subsampling
AI Read Science
Must-Reading Tree
Example
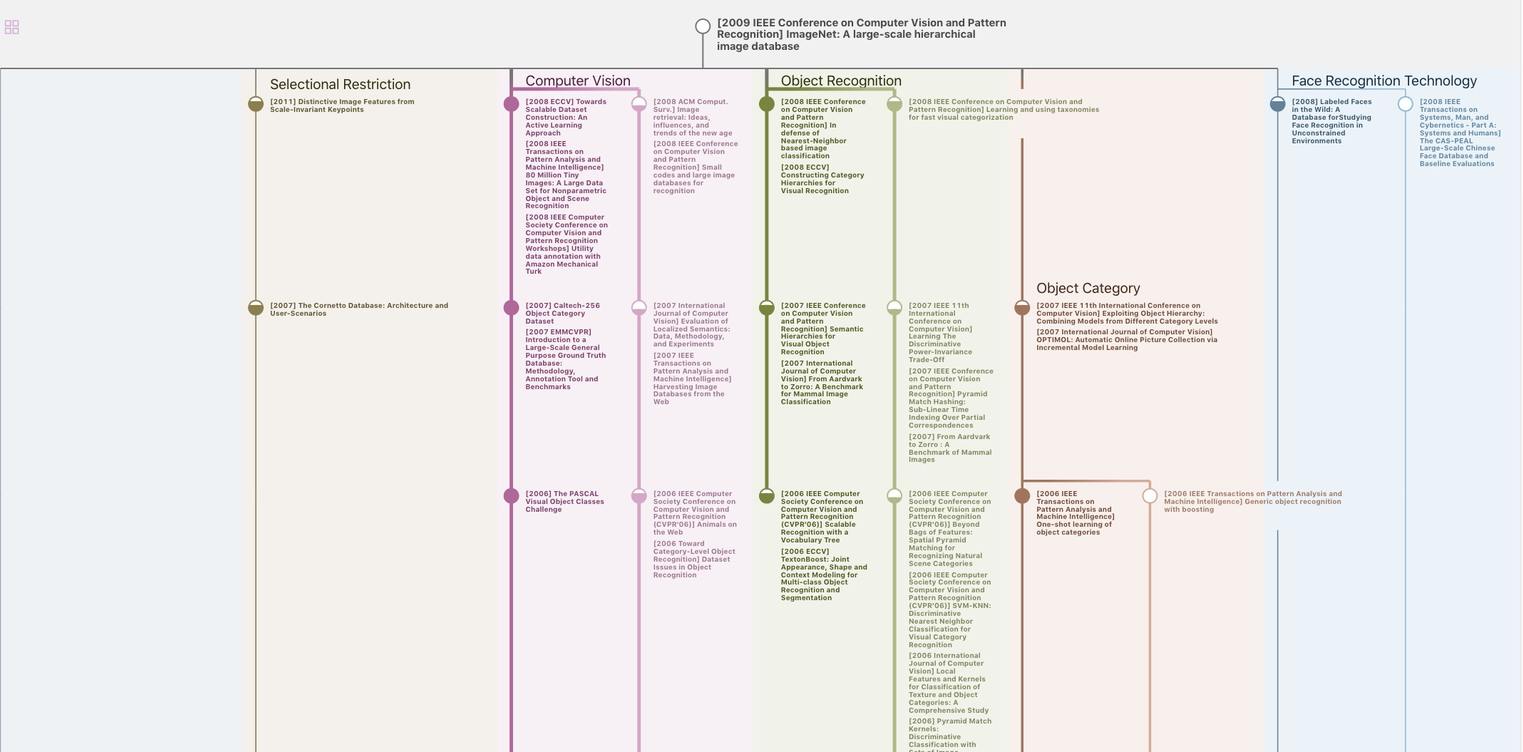
Generate MRT to find the research sequence of this paper
Chat Paper
Summary is being generated by the instructions you defined