Tensor Decomposition for Salient Object Detection in Images.
Big Data Cogn. Comput.(2019)
摘要
The fundamental challenge of salient object detection is to find the decision boundary that separates the salient object from the background. Low-rank recovery models address this challenge by decomposing an image or image feature-based matrix into a low-rank matrix representing the image background and a sparse matrix representing salient objects. This method is simple and efficient in finding salient objects. However, it needs to convert high-dimensional feature space into a two-dimensional matrix. Therefore, it does not take full advantage of image features in discovering the salient object. In this article, we propose a tensor decomposition method which considers spatial consistency and tries to make full use of image feature information in detecting salient objects. First, we use high-dimensional image features in tensor to preserve spatial information about image features. Following this, we use a tensor low-rank and sparse model to decompose the image feature tensor into a low-rank tensor and a sparse tensor, where the low-rank tensor represents the background and the sparse tensor is used to identify the salient object. To solve the tensor low-rank and sparse model, we employed a heuristic strategy by relaxing the definition of tensor trace norm and tensor l1-norm. Experimental results on three saliency benchmarks demonstrate the effectiveness of the proposed tensor decomposition method.
更多查看译文
关键词
salient object, saliency detection, low-rank matrix recovery, tensor decomposition
AI 理解论文
溯源树
样例
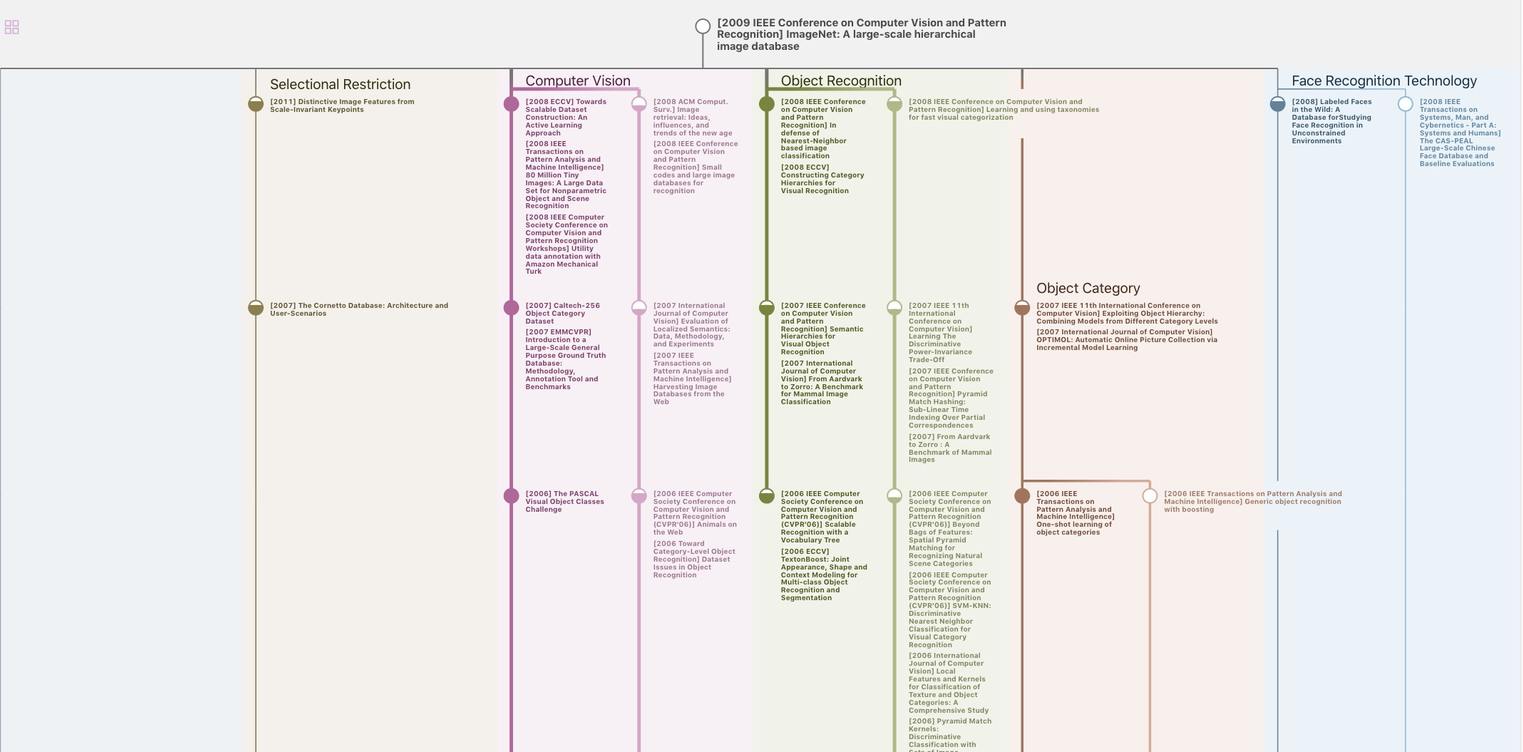
生成溯源树,研究论文发展脉络
Chat Paper
正在生成论文摘要