Adversarial Mutual Information Learning for Network Embedding
PROCEEDINGS OF THE TWENTY-NINTH INTERNATIONAL JOINT CONFERENCE ON ARTIFICIAL INTELLIGENCE(2020)
摘要
Network embedding which is to learn a low dimensional representation of nodes in a network has been used in many network analysis tasks. Some network embedding methods, including those based on generative adversarial networks (GAN) (a promising deep learning technique), have been proposed recently. Existing GAN-based methods typically use GAN to learn a Gaussian distribution as a priori for network embedding. However, this strategy makes it difficult to distinguish the node representation from Gaussian distribution. Moreover, it does not make full use of the essential advantage of GAN (that is to adversarially learn the representation mechanism rather than the representation itself), leading to compromised performance of the method. To address this problem, we propose to use the adversarial idea on the representation mechanism, i.e. on the encoding mechanism under the framework of autoencoder. Specifically, we use the mutual information between node attributes and embedding as a reasonable alternative of this encoding mechanism (which is much easier to track). Additionally, we introduce another mapping mechanism (which is based on GAN) as a competitor into the adversarial learning system. A range of empirical results demonstrate the effectiveness of the proposed approach.
更多查看译文
关键词
Representation Learning,Network Embedding,Knowledge Graph Embedding,Adversarial Examples,Relational Data Modeling
AI 理解论文
溯源树
样例
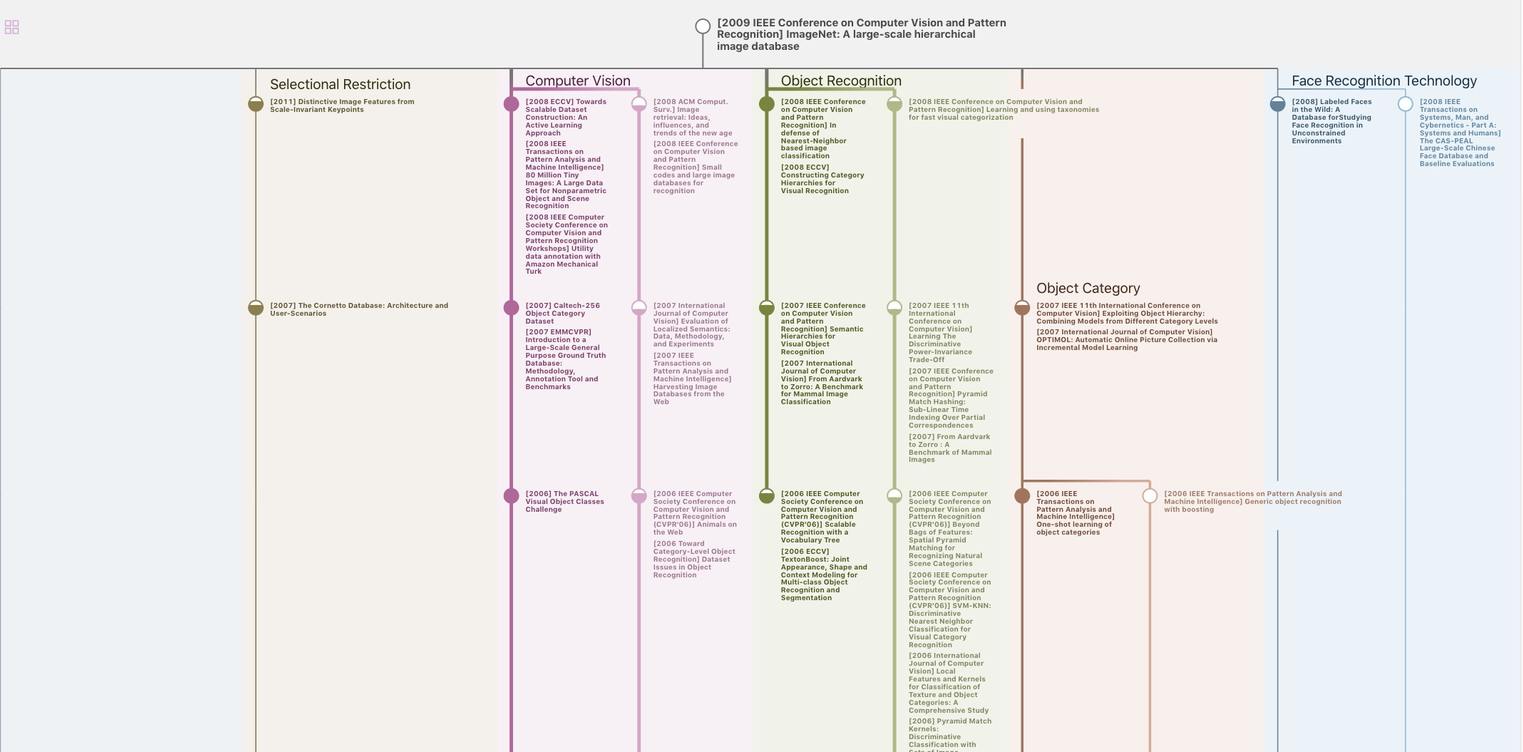
生成溯源树,研究论文发展脉络
Chat Paper
正在生成论文摘要