Restoration of Out-of-Focus Fluorescence Microscopy Images Using Learning-Based Depth-Variant Deconvolution
IEEE Photonics Journal(2020)
摘要
Image quality is degraded in the out-of-focus region because of the depth-variant (DV) point spread function (DV-PSF) of a fluorescence microscope. Either non-blind or blind deconvolution for restoration results in limited improvement. In this work, we propose a two-step learning-based DV deconvolution (LB-DVD) to restore the out-of-focus image. In the first step, DV-PSF is predicted by a defocus level prediction convolutional neural network (DelpNet). In the second step, the extracted DV-PSF is used for DV deconvolution. To our knowledge, LB-DVD is proposed and demonstrated for the first time. DelpNet achieves an accuracy of 98.2% for predicting defocus levels of image patches (84 x 84 pixels). The subsequent DV deconvolution gives rise to good performance in peak signal-to-noise ratios and structural similarity index, which are improved by up to 6.6 dB and 11%, respectively, before and after the deconvolution. As for a wide-field image, there exist different DV-PSFs within the two-dimensional fluorescence image due to the surface undulation. An overlapping weighting patch-wise LB-DVD is used in image montage to eliminate patch boundary artifacts. As a result, our LB-DVD shows the feasibility and promise to be applied to typical fluorescence microscopy in practical applications.
更多查看译文
关键词
Fluorescence microscopy,convolutional neural network,deconvolution
AI 理解论文
溯源树
样例
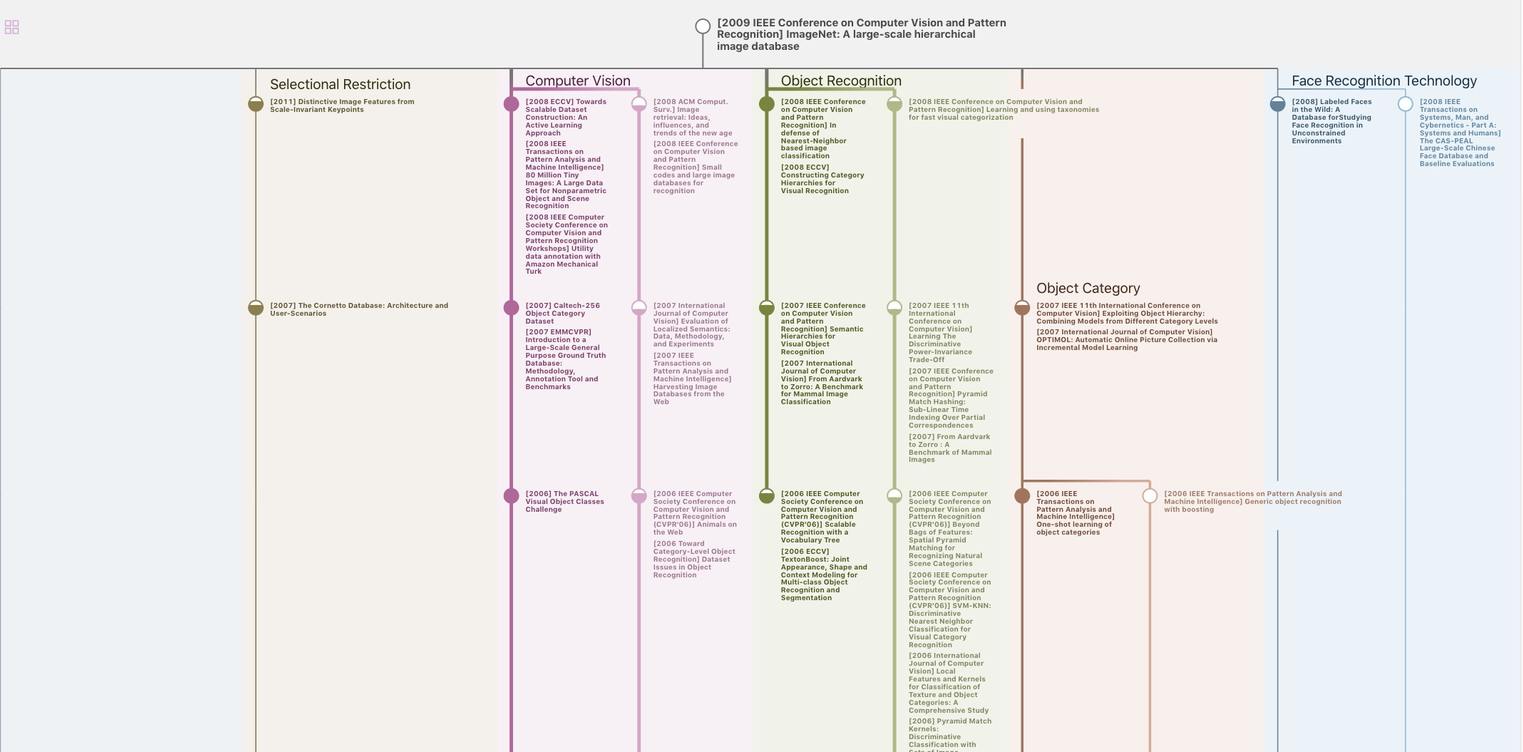
生成溯源树,研究论文发展脉络
Chat Paper
正在生成论文摘要