Wireless control using reinforcement learning for practical web QoE
Computer Communications(2020)
摘要
Wireless networks show several challenges not found in wired networks, due to the dynamics of data transmission. Besides, home wireless networks are managed by non-technical people, and providers do not implement full management services because of the difficulties of manually managing thousands of devices. Thus, automatic management mechanisms are desirable. However, such control mechanisms are hard to achieve in practice because we do not always have a model of the process to be controlled, or the behavior of the environment is dynamic. Thus, the control must adapt to changing conditions, and it is necessary to identify the quality of the control executed from the perspective of the user of the network service. This article proposes a control loop for transmission power and channel selection, based on Software Defined Networking and Reinforcement Learning (RL), and capable of improving Web Quality of Experience metrics, thus benefiting the user. We evaluate a prototype in which some Access Points are controlled by a single controller or by independent controllers. The control loop uses the predicted Mean Opinion Score (MOS) as a reward, thus the system needs to classify the web traffic. We proposed a semi-supervised learning method to classify the web sites into three classes (light, average and heavy) that groups pages by their complexity, i.e. number and size of page elements. These classes define the MOS predictor used by the control loop. The proposed web site classifier achieves an average score of 87%±1%, classifying 500 unlabeled examples with only fifteen known examples, with a sub-second runtime. Further, the RL control loop achieves higher Mean Opinion Score (up to 167% in our best result) than the baselines. The page load time of clients browsing heavy web sites is improved by up to 6.6x.
更多查看译文
关键词
Wireless network,Software defined network,Reinforcement learning,Q-Learning,Multi-armed bandit,Quality of Experience
AI 理解论文
溯源树
样例
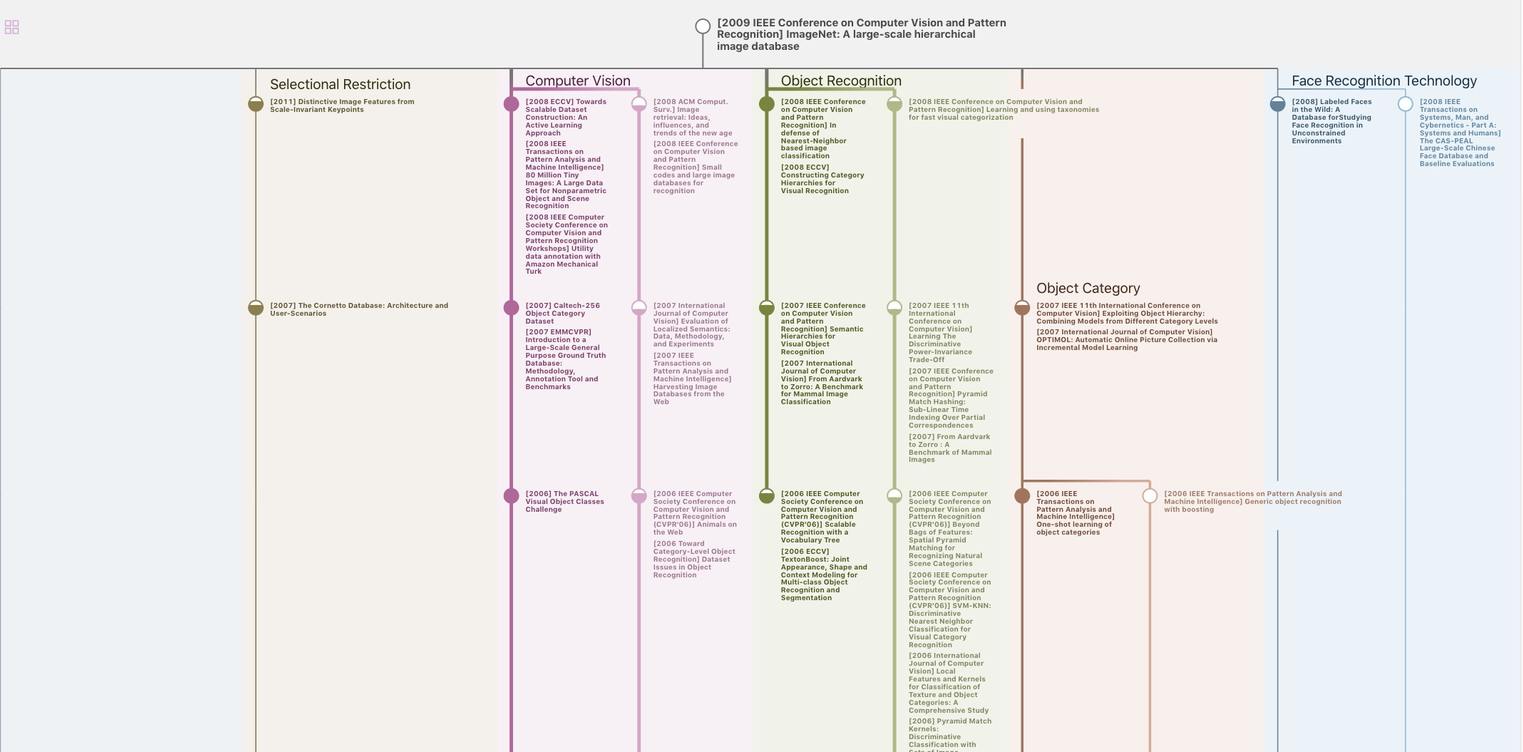
生成溯源树,研究论文发展脉络
Chat Paper
正在生成论文摘要