Developing a new mesh quality evaluation method based on convolutional neural network
ENGINEERING APPLICATIONS OF COMPUTATIONAL FLUID MECHANICS(2020)
Abstract
One of the difficult requirements imposed on high-quality CFD mesh generation has been the ability to evaluate the mesh quality efficiently. Due to the lack of a general and effective evaluating criterion, the current mesh quality evaluation task mainly relies on various quality metrics for the shape of mesh elements, such as angle, radius, edge and contextual information collected by pre-processing software. However, this line of methods greatly increases the pre-processing cost and may not guarantee a precise quality result. In this paper, we provide a solution to solve the mentioned issues, resulting in a CNN model GridNet and the first mesh dataset NACA-Market. GridNet takes the mesh file as input and then automatically evaluates the mesh quality. Experiment results show that GridNet is capable of performing automatic mesh quality evaluation and outperforms the widely used classifiers. We hope that the proposed large benchmark collection and network could fill in the gaps in the fields of CNN-based mesh quality evaluation and provide potential future research directions in this field.
MoreTranslated text
Key words
Computational fluid dynamics (CFD),mesh quality evaluation,convolutional neural network (CNN),mesh dataset
AI Read Science
Must-Reading Tree
Example
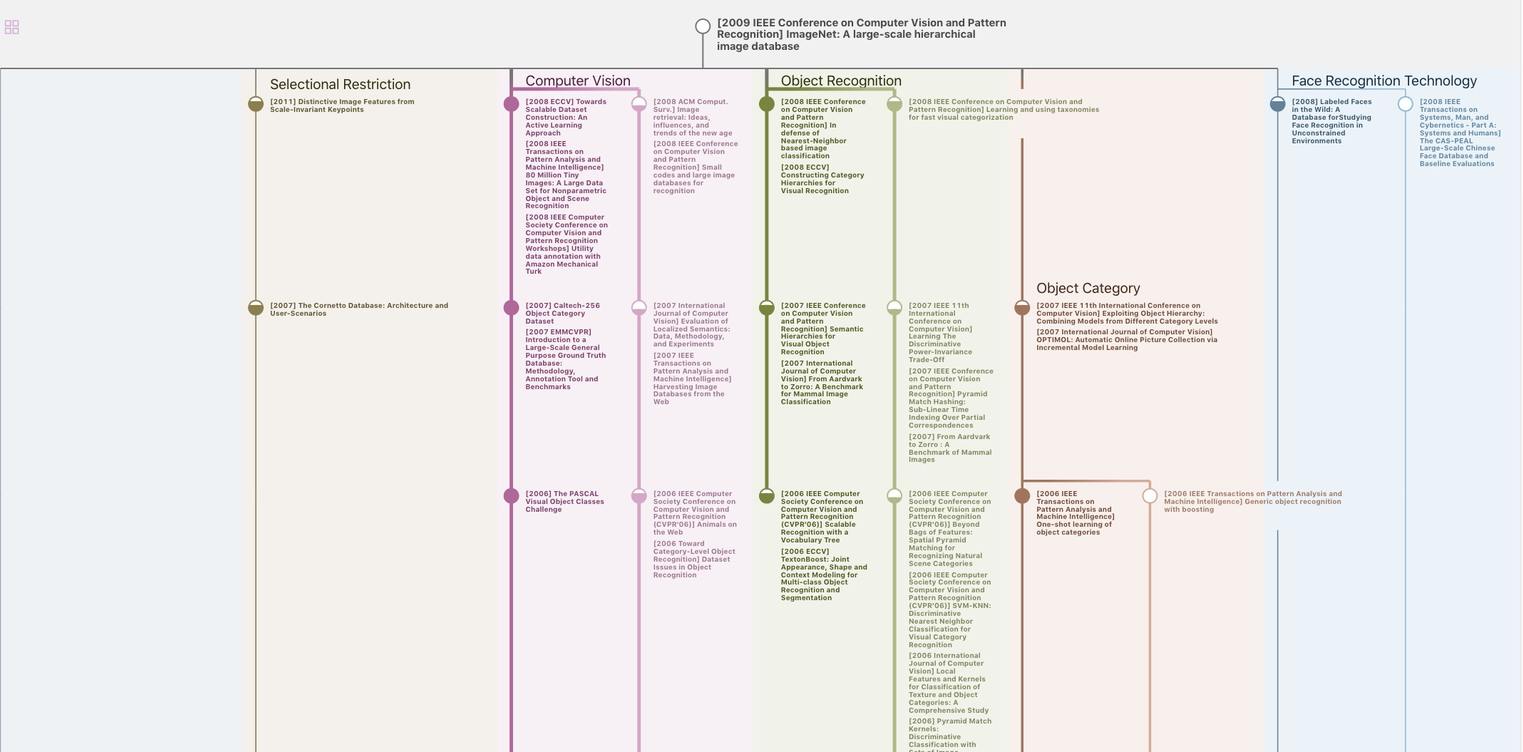
Generate MRT to find the research sequence of this paper
Chat Paper
Summary is being generated by the instructions you defined