Efficient Hindsight Reinforcement Learning Using Demonstrations For Robotic Tasks With Sparse Rewards
INTERNATIONAL JOURNAL OF ADVANCED ROBOTIC SYSTEMS(2020)
Abstract
The goal of reinforcement learning is to enable an agent to learn by using rewards. However, some robotic tasks naturally specify with sparse rewards, and manually shaping reward functions is a difficult project. In this article, we propose a general and model-free approach for reinforcement learning to learn robotic tasks with sparse rewards. First, a variant of Hindsight Experience Replay, Curious and Aggressive Hindsight Experience Replay, is proposed to improve the sample efficiency of reinforcement learning methods and avoid the need for complicated reward engineering. Second, based on Twin Delayed Deep Deterministic policy gradient algorithm, demonstrations are leveraged to overcome the exploration problem and speed up the policy training process. Finally, the action loss is added into the loss function in order to minimize the vibration of output action while maximizing the value of the action. The experiments on simulated robotic tasks are performed with different hyperparameters to verify the effectiveness of our method. Results show that our method can effectively solve the sparse reward problem and obtain a high learning speed.
MoreTranslated text
Key words
Robot learning, reinforcement learning, sparse reward, CAHER, demonstrations
AI Read Science
Must-Reading Tree
Example
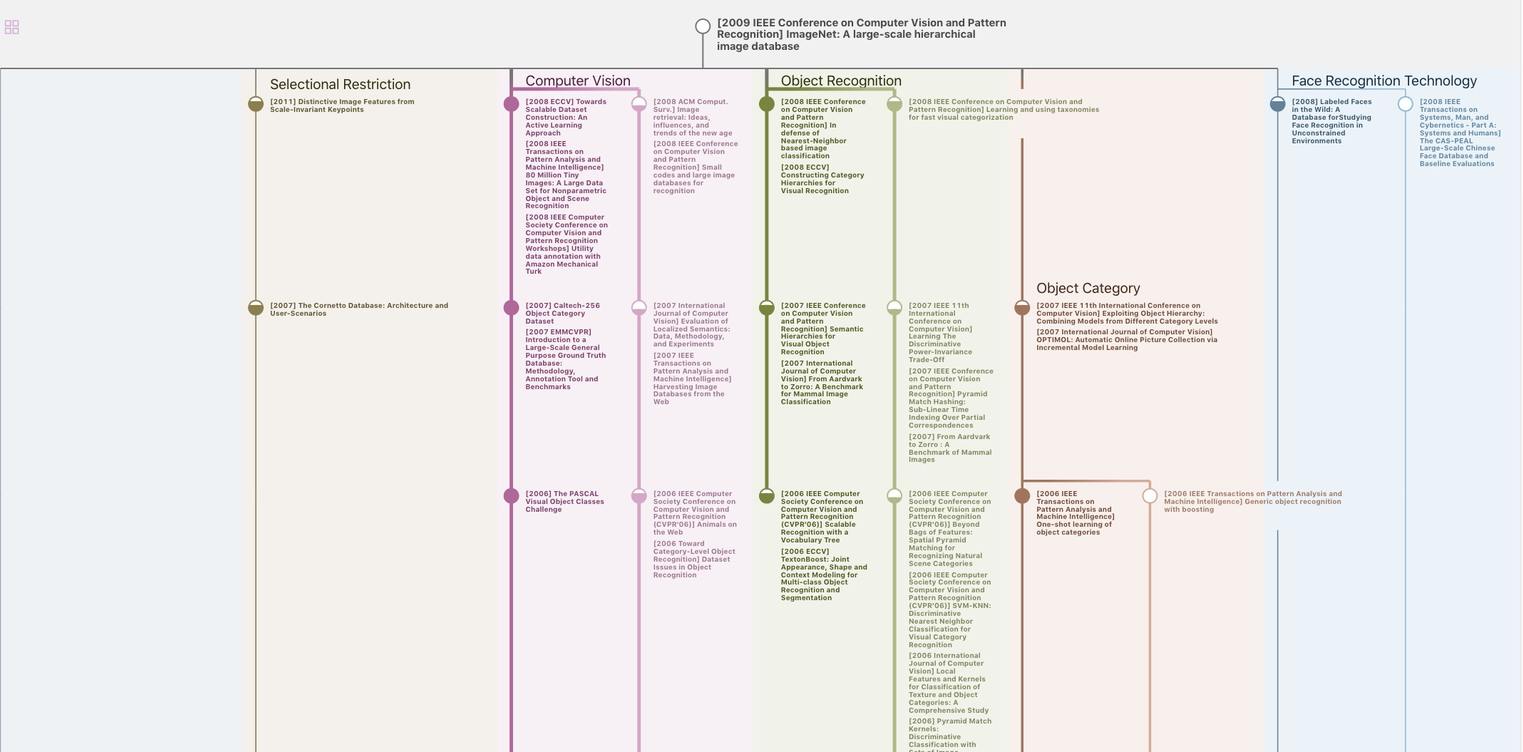
Generate MRT to find the research sequence of this paper
Chat Paper
Summary is being generated by the instructions you defined