A Graph Fusion Approach for Cross-Lingual Machine Reading Comprehension
AAAI 2023(2023)
摘要
Although great progress has been made for Machine Reading Comprehension (MRC) in English, scaling out to a large number of languages remains a huge challenge due to the lack of large amounts of annotated training data in non-English languages. To address this challenge, some recent efforts of cross-lingual MRC employ machine translation to transfer knowledge from English to other languages, through either explicit alignment or implicit attention. For effective knowledge transition, it is beneficial to leverage both semantic and syntactic information. However, the existing methods fail to explicitly incorporate syntax information in model learning. Consequently, the models are not robust to errors in alignment and noises in attention. In this work, we propose a novel approach, which jointly models the cross-lingual alignment information and the mono-lingual syntax information using a graph. We develop a series of algorithms, including graph construction, learning, and pre-training. The experiments on two benchmark datasets for cross-lingual MRC show that our approach outperforms all strong baselines, which verifies the effectiveness of syntax information for cross-lingual MRC.
更多查看译文
关键词
SNLP: Machine Translation & Multilinguality
AI 理解论文
溯源树
样例
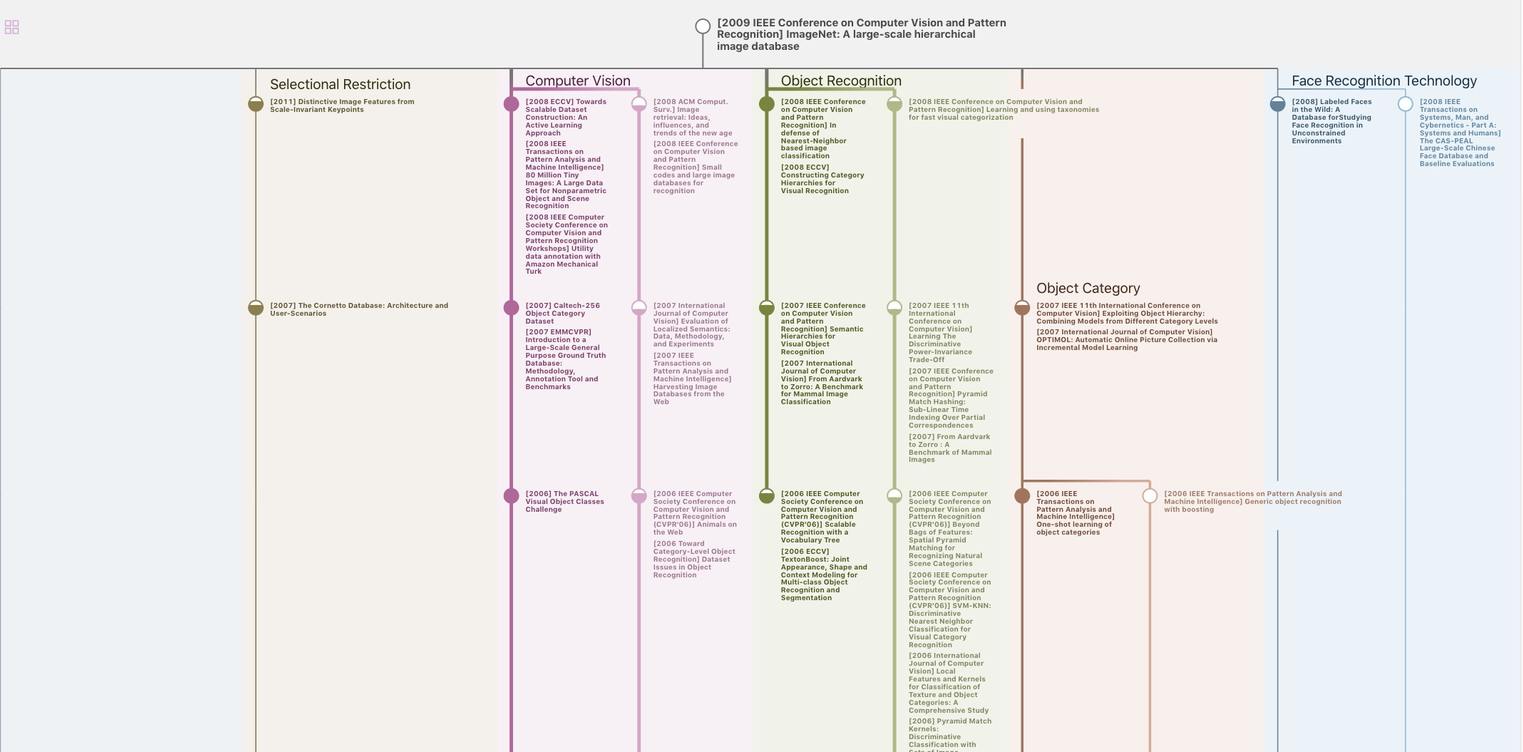
生成溯源树,研究论文发展脉络
Chat Paper
正在生成论文摘要