Gradient-Based Adversarial Training on Transformer Networks for Detecting Check-Worthy Factual Claims.
CoRR(2020)
摘要
This paper presents the latest developments to ClaimBuster's claim-spotting model, which tackles the critical task of identifying check-worthy claims from large streams of information. We introduce the first adversarially-regularized, transformer-based claim-spotting model, which achieves state-of-the-art results on several benchmark datasets. In addition to analyzing model performance metrics, we also quantitatively and qualitatively analyze the impact of ClaimBuster's real-world deployment. Moreover, to help facilitate reproducibility and community engagement, we publicly release our codebase, dataset, data curation platform, API, Google Colab notebooks, and various ClaimBuster-based demo systems, at claimbuster.org.
更多查看译文
关键词
fact checking,computational journalism,misinformation,transformer,adversarial training,natural language processing,machine learning,deployed systems,emerging applications and technology
AI 理解论文
溯源树
样例
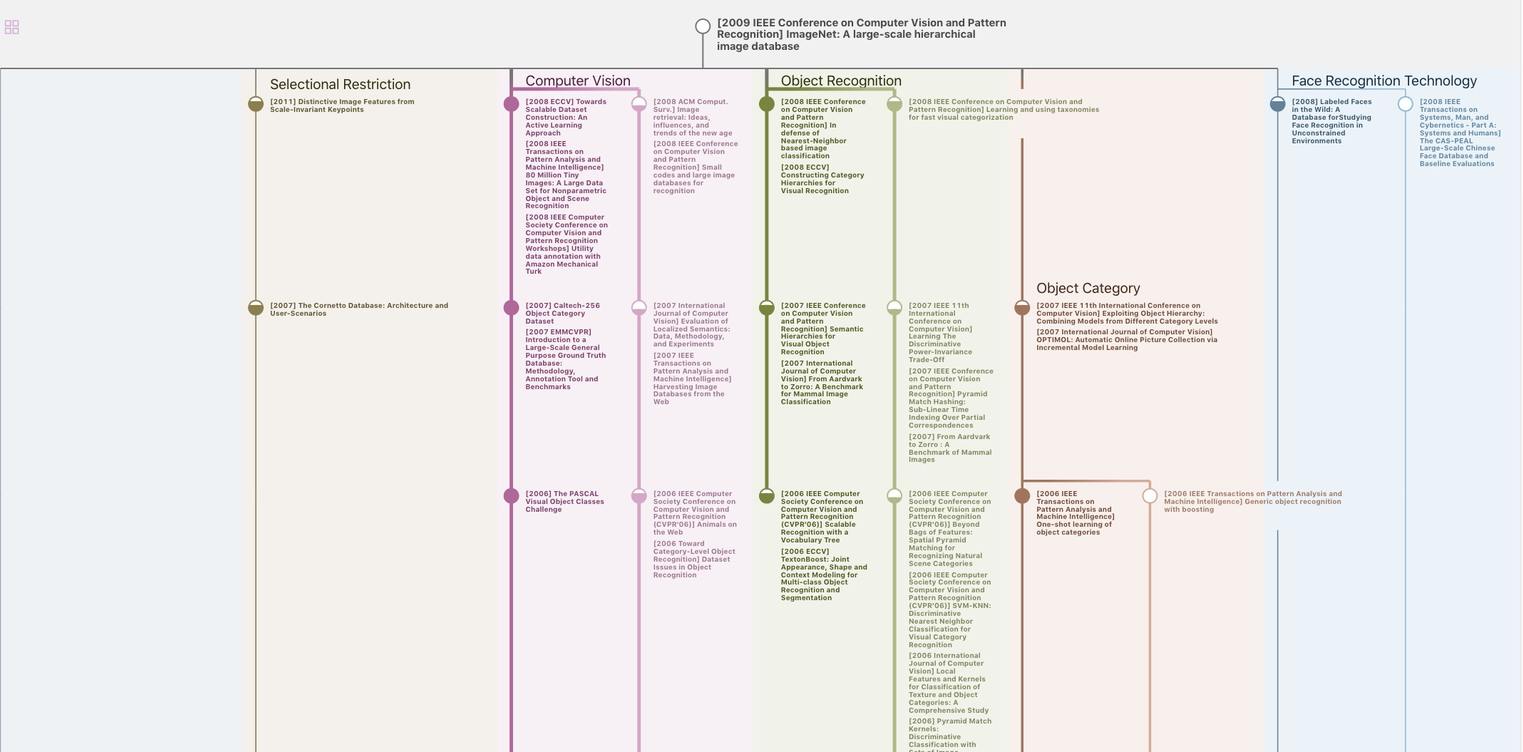
生成溯源树,研究论文发展脉络
Chat Paper
正在生成论文摘要