Evaluation Of Backscattering Models And Support Vector Machine For The Retrieval Of Bare Soil Moisture From Sentinel-1 Data
REMOTE SENSING(2020)
摘要
The main objective of this work was to retrieve surface soil moisture (SSM) by using scattering models and a support vector machine (SVM) technique driven by backscattering coefficients obtained from Sentinel-1 satellite images acquired over bare agricultural soil in the Tensfit basin of Morocco. Two backscattering models were selected in this study due to their wide use in inversion procedures: the theoretical integral equation model (IEM) and the semi-empirical model (Oh). To this end, the sensitivity of the SAR backscattering coefficients at VV (sigma vv degrees) and VH (sigma vh degrees) polarizations to in situ soil moisture data were analyzed first. As expected, the results showed that over bare soil the sigma vv degrees was well correlated with SSM compared to the sigma vh degrees, which showed more dispersion with correlation coefficients values (r) of about 0.84 and 0.61 for the VV and VH polarizations, respectively. Afterwards, these values of sigma vv degrees were compared to those simulated by the backscatter models. It was found that IEM driven by the measured length correlation L slightly underestimated SAR backscatter coefficients compared to the Oh model with a bias of about -0.7 dB and -1.2 dB and a root mean square (RMSE) of about 1.1 dB and 1.5 dB for Oh and IEM models, respectively. However, the use of an optimal value of L significantly improved the bias of IEM, which became near to zero, and the RMSE decreased to 0.9 dB. Then, a classical inversion approach of sigma vv degrees observations based on backscattering model is compared to a data driven retrieval technic (SVM). By comparing the retrieved soil moisture against ground truth measurements, it was found that results of SVM were very encouraging and were close to those obtained by IEM model. The bias and RMSE were about 0.28 vol.% and 2.77 vol.% and -0.13 vol.% and 2.71 vol.% for SVM and IEM, respectively. However, by taking into account the difficultly of obtaining roughness parameter at large scale, it was concluded that SVM is still a useful tool to retrieve soil moisture, and therefore, can be fairly used to generate maps at such scales.
更多查看译文
关键词
soil moisture,synthetic aperture radar (SAR),Sentinel-1,semi-empirical and theoretical backscatter models,support vector machine,bare soil
AI 理解论文
溯源树
样例
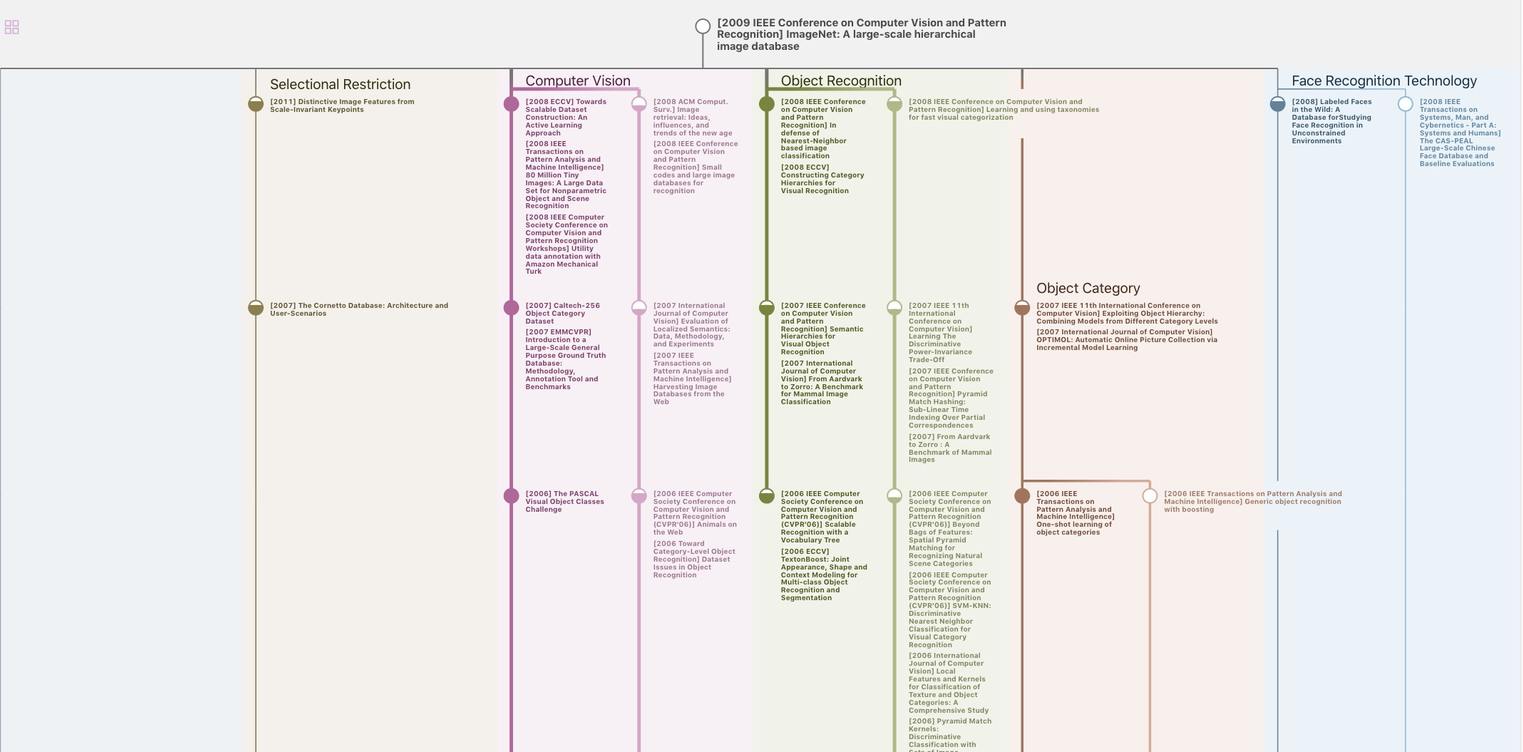
生成溯源树,研究论文发展脉络
Chat Paper
正在生成论文摘要