Time evolution of the characteristic and probability density function of diffusion processes via neural networks
arXiv (Cornell University)(2020)
Abstract
We investigate the use of physics-informed neural networks-based solution of the PDE satisfied by the probability density function (pdf) of the state of a dynamical system subject to random forcing. Two alternatives for the PDE are considered: the Fokker-Planck equation and a PDE for the characteristic function (chf) of the state, both of which provide the same probabilistic information. Solving these PDEs using the finite element method is unfeasible when the dimension of the state is larger than 3. We examine analytically and numerically the advantages and disadvantages of solving the corresponding PDE of one over the other. It is also demonstrated how prior information of the dynamical system can be exploited to design and simplify the neural network architecture. Numerical examples show that: 1) the neural network solution can approximate the target solution even for partial integro-differential equations and system of PDEs, 2) solving either PDE using neural networks yields similar pdfs of the state, and 3) the solution to the PDE can be used to study the behavior of the state for different types of random forcings.
MoreTranslated text
Key words
diffusion processes,neural networks,probability density function
AI Read Science
Must-Reading Tree
Example
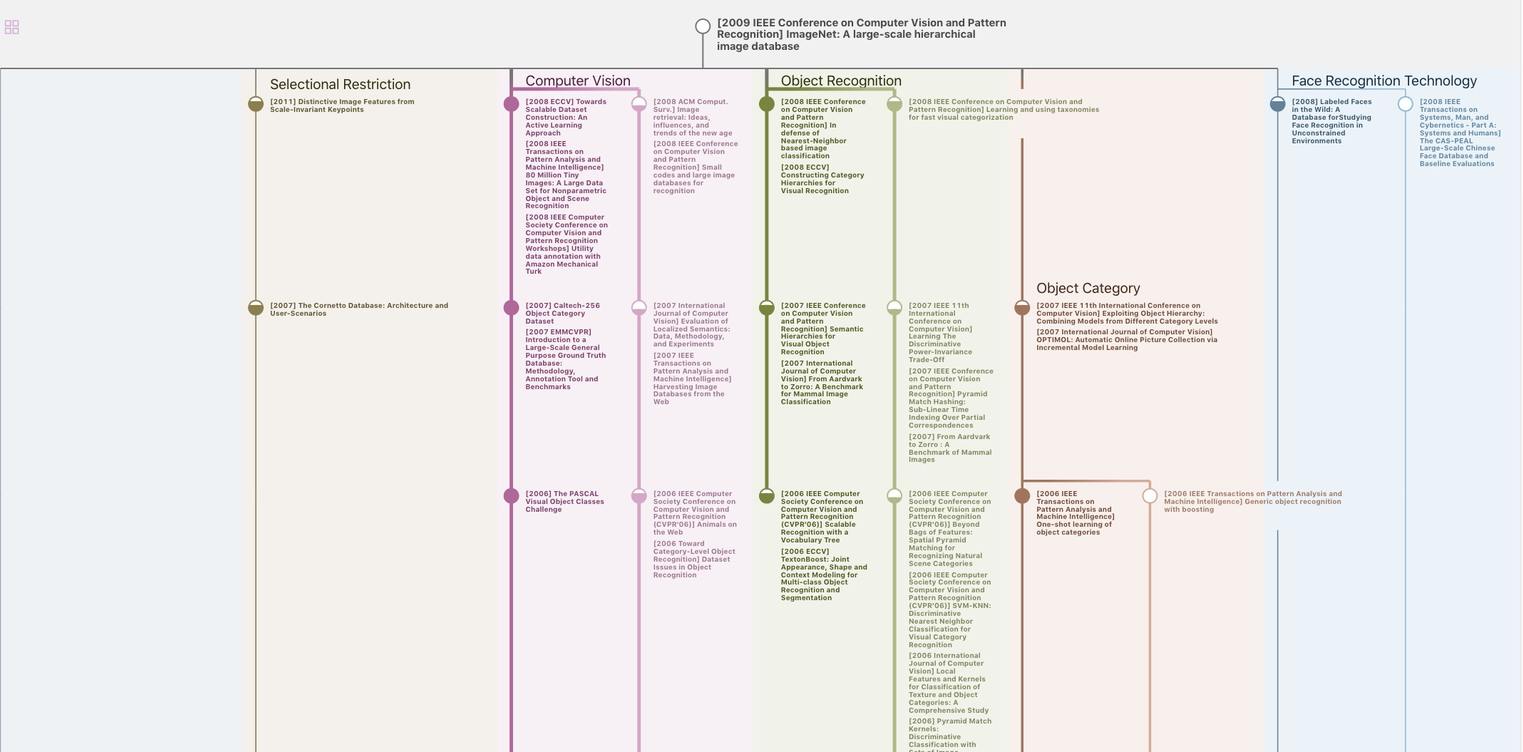
Generate MRT to find the research sequence of this paper
Chat Paper
Summary is being generated by the instructions you defined