Attentionanatomy: a unified framework for whole-body organs at risk segmentation using multiple partially annotated datasets
2020 IEEE 17TH INTERNATIONAL SYMPOSIUM ON BIOMEDICAL IMAGING (ISBI 2020)(2020)
摘要
Organs-at-risk (OAR) delineation in computed tomography (CT) is an important step in Radiation Therapy (RT) planning. Recently, deep learning based methods for OAR delineation have been proposed and applied in clinical practice for separate regions of the human body (head and neck, thorax, and abdomen). However, there are few researches regarding the end-to-end whole-body OARs delineation because the existing datasets are mostly partially or incompletely annotated for such task. In this paper, our proposed end-to-end convolutional neural network model, called AttentionAnatomy, can be jointly trained with three partially annotated datasets, segmenting OARs from whole body. Our main contributions are: 1) an attention module implicitly guided by body region label to modulate the segmentation branch output; 2) a prediction re-calibration operation, exploiting prior information of the input images, to handle partial-annotation(HPA) problem; 3) a new hybrid loss function combining batch Dice loss and spatially balanced focal loss to alleviate the organ size imbalance problem. Experimental results of our proposed framework presented significant improvements in both Sorensen-Dice coefficient (DSC) and 95% Hausdorff distance compared to the baseline model.
更多查看译文
关键词
whole body,automated anatomy segmentation,partial annotations,deep learning
AI 理解论文
溯源树
样例
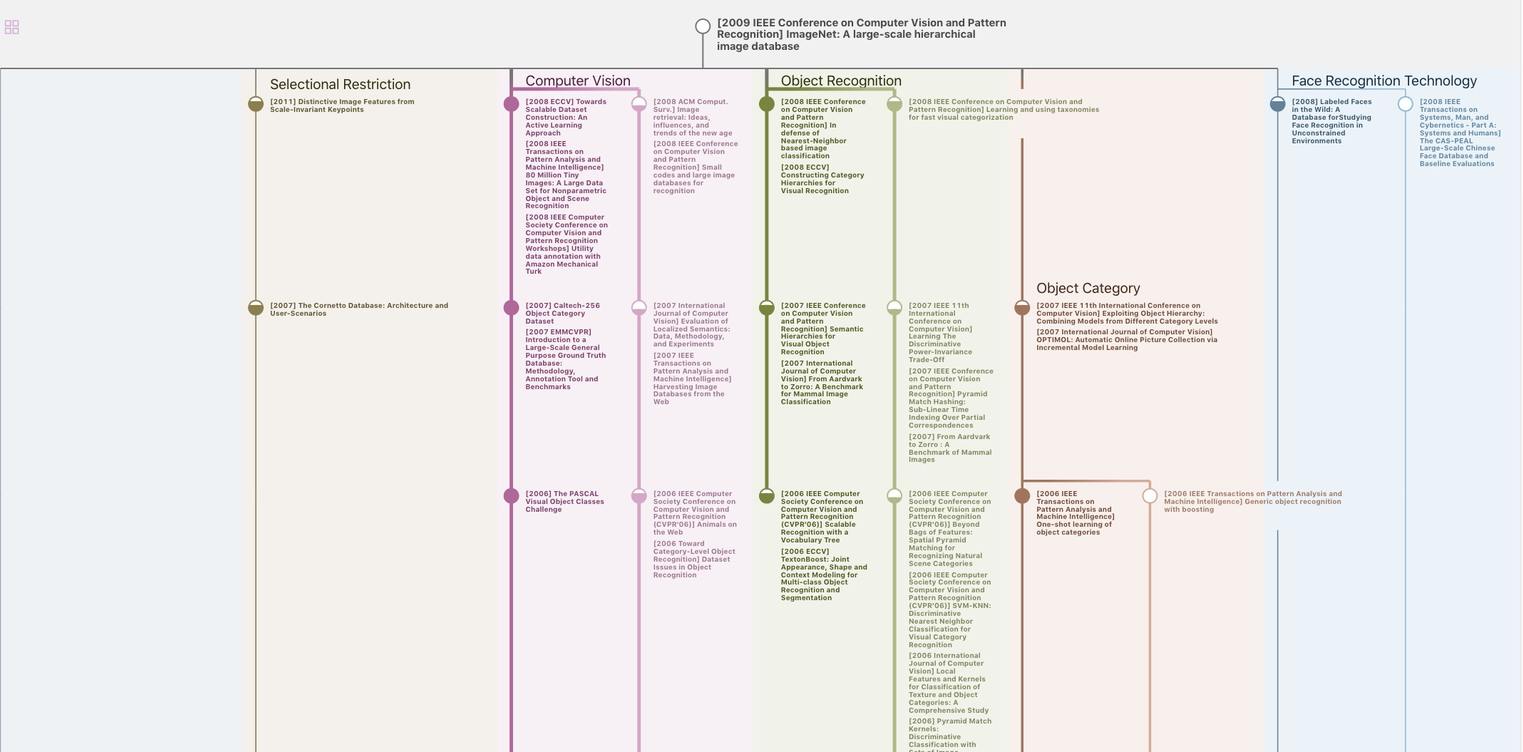
生成溯源树,研究论文发展脉络
Chat Paper
正在生成论文摘要