Multiple Kernel Learning Applied To The Prediction Of Prostate Cancer Recurrence From Mri Radiomic Features
2019 IEEE 19TH INTERNATIONAL CONFERENCE ON BIOINFORMATICS AND BIOENGINEERING (BIBE)(2019)
Abstract
Radiomics refers to the quantification of images by the extraction and analysis of a large number of features from different modalities, aiming to establish potential links between them and disease phenotypes. It can potentially predict the free-disease survival or allow the selection of patients at risk, thereby leading to the development of more personalized treatments. The development of robust prediction models is cumbersome as we deal with a high multidimensional problem, where a high number of features can be available but with a low number of individuals. To cope with this problem, we propose in this paper the use of Multiple Kernel Learning (MKL), which allows a selection of more relevant features and its optimal combination in a classification model. The method was evaluated on a dataset of patients of prostate cancer treated with radiotherapy, which is the second most prevalent cancer in men worldwide, for whom we predicted the risk of recurrence. MKL allowed the selection of 7 features out of 98 to build a reliable model with an accuracy of 94.7%, Sensitivity of 75%, and specificity of 97.78%. Compared to other classification methods, MKL achieved significantly higher performance, emerging like a suited methodology within radiomic studies.
MoreTranslated text
Key words
Prostate cancer, Recurrence prediction, Radiomics, MKL, MRI
AI Read Science
Must-Reading Tree
Example
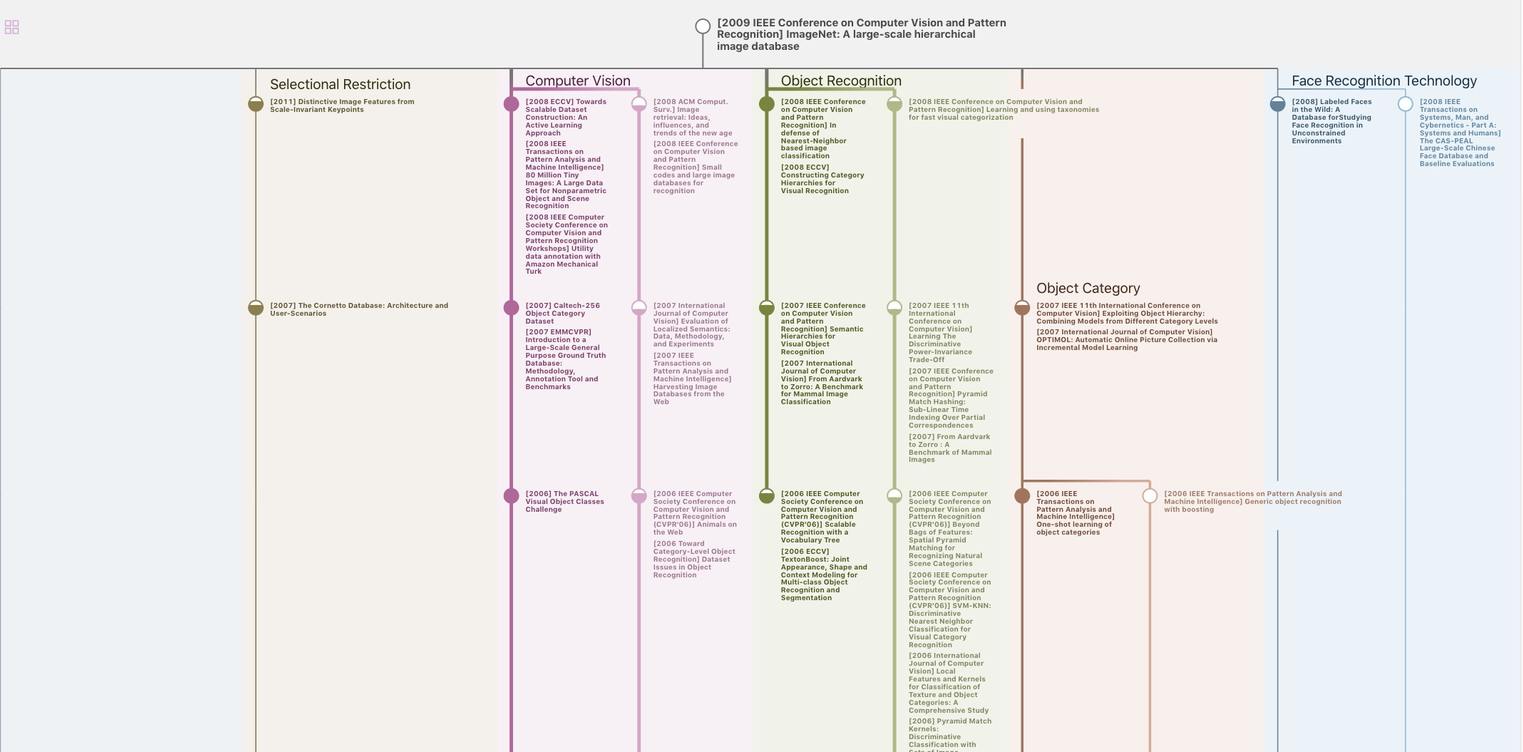
Generate MRT to find the research sequence of this paper
Chat Paper
Summary is being generated by the instructions you defined