Human-Like Highway Trajectory Modeling Based On Inverse Reinforcement Learning
2019 IEEE INTELLIGENT TRANSPORTATION SYSTEMS CONFERENCE (ITSC)(2019)
Abstract
Autonomous driving is one of the current cutting edge technologies. For autonomous cars, their driving actions and trajectories should not only achieve autonomy and safety, but also obey human drivers' behavior patterns, when sharing the roads with other human drivers on the highway. Traditional methods, though robust and interpretable, demands much human labor in engineering the complex mapping from current driving situation to vehicle's future control. For newly developed deep-learning methods, though they can automatically learn such complex mapping from data and demands fewer humans' engineering, they mostly act like black-box, and are less interpretable. We proposed a new combined method based on inverse reinforcement learning to harness the advantages of both. Experimental validations on lane-change prediction and human-like trajectory planning show that the proposed method approximates the state-of-the-art performance in modeling human trajectories, and is both interpretable and data-driven.
MoreTranslated text
Key words
human-like highway trajectory planning,safety,autonomous cars,autonomous driving,highway trajectory modeling,lane-change prediction,inverse reinforcement learning,complex mapping,deep-learning methods
AI Read Science
Must-Reading Tree
Example
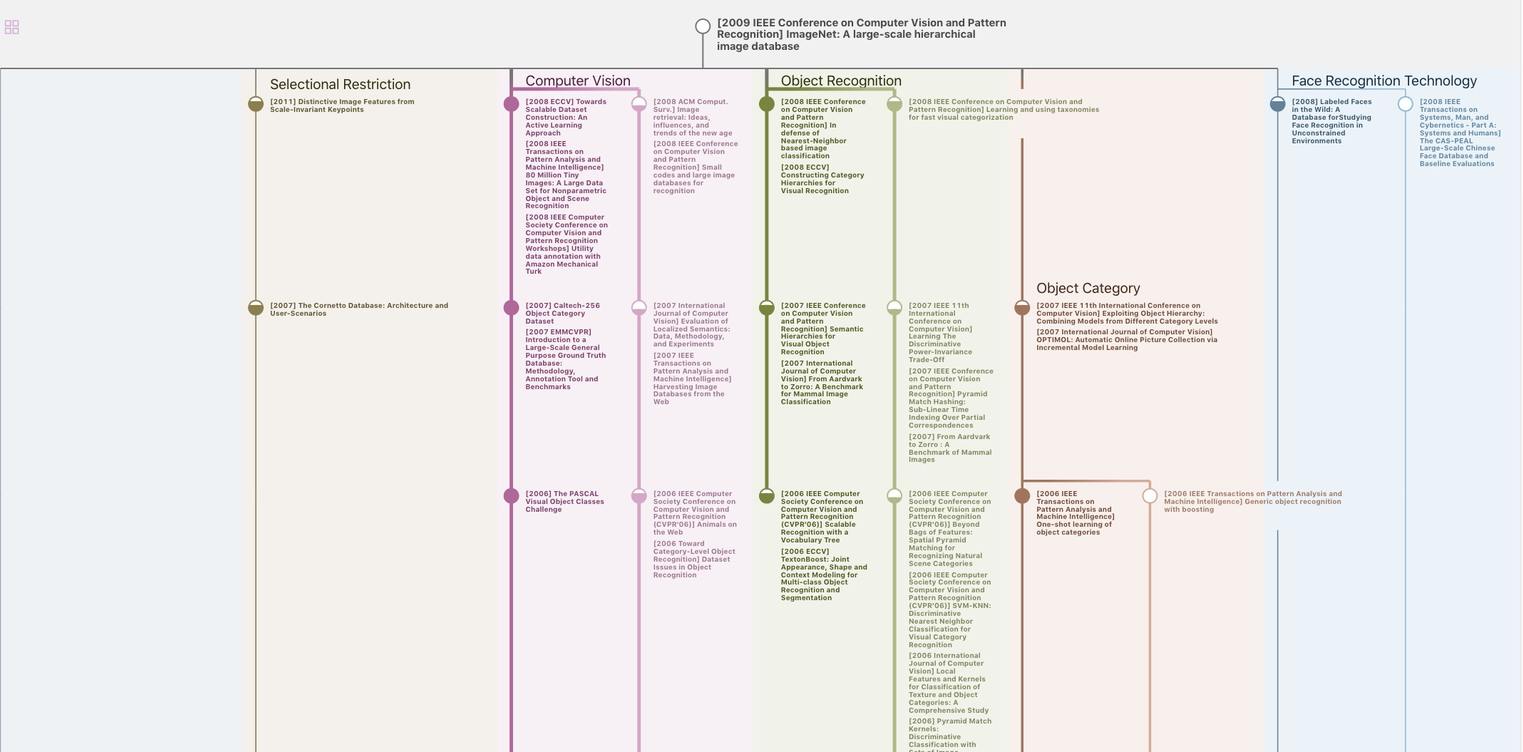
Generate MRT to find the research sequence of this paper
Chat Paper
Summary is being generated by the instructions you defined