Neural Network Generalization: the Impact of Camera Parameters
IEEE access(2020)
摘要
We quantify the generalization of a convolutional neural network (CNN) trained to identify cars. First, we perform a series of experiments to train the network using one image dataset - either synthetic or from a camera - and then test on a different image dataset. We show that generalization between images obtained with different cameras is roughly the same as generalization between images from a camera and ray-traced multispectral synthetic images. Second, we use ISETAuto, a soft prototyping tool that creates ray-traced multispectral simulations of camera images, to simulate sensor images with a range of pixel sizes, color filters, acquisition and post-acquisition processing. These experiments reveal how variations in specific camera parameters and image processing operations impact CNN generalization. We find that (a) pixel size impacts generalization, (b) demosaicking substantially impacts performance and generalization for shallow (8-bit) bit-depths but not deeper ones (10-bit), (c) the network performs well using raw (not demosaicked) sensor data for 10-bit pixels.
更多查看译文
关键词
Imaging systems,camera design,autonomous driving,network generalization,convolutional neural network,physically based ray tracing
AI 理解论文
溯源树
样例
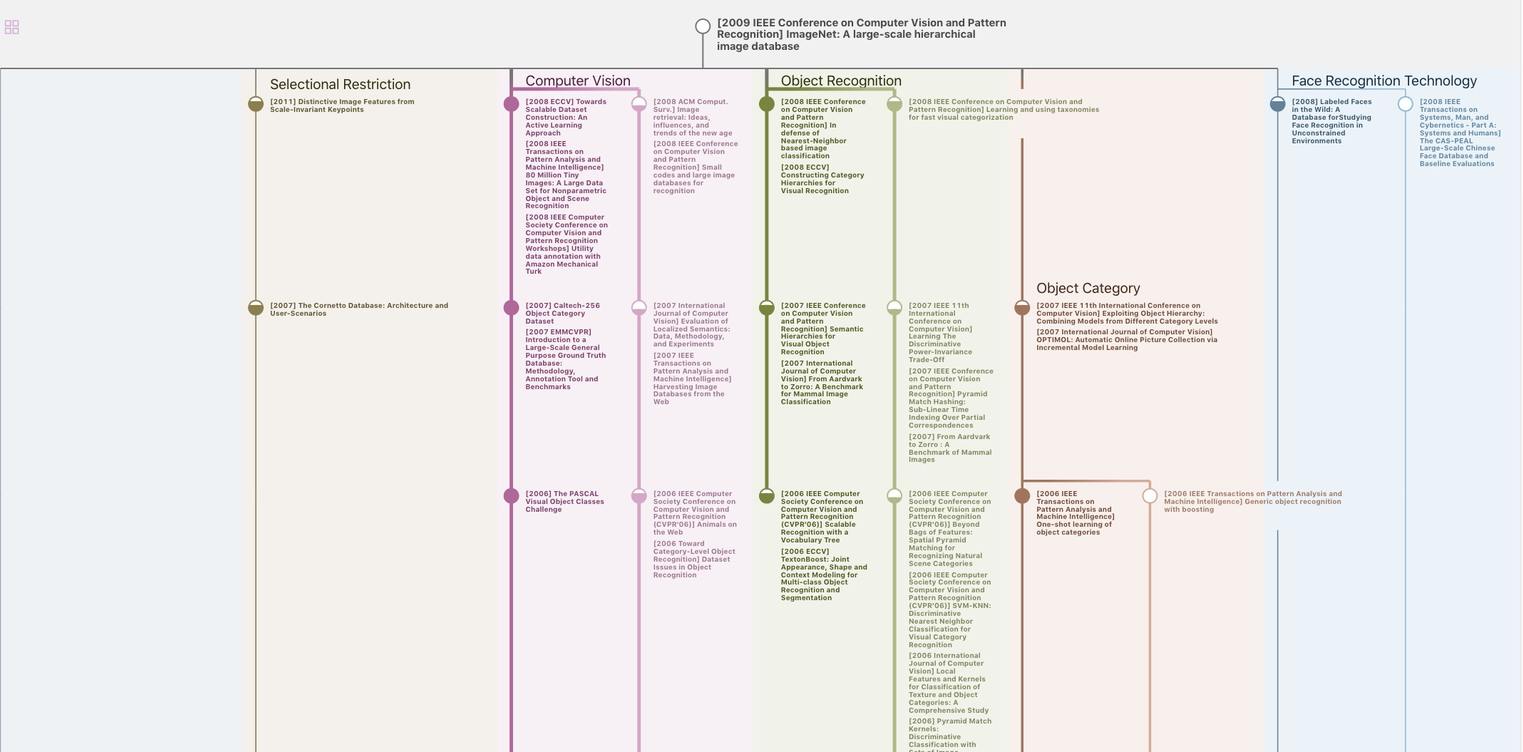
生成溯源树,研究论文发展脉络
Chat Paper
正在生成论文摘要