Music Visualization Based On Spherical Projection With Adjustable Metrics
IEEE ACCESS(2019)
摘要
Development of techniques for music visualization is important and still open problem in analysis and creation of the quantitative profiles of single or multiple compositions, which could be used as required constraints in music generation or music classification processes. When generating creative data with no objective function, it is hard to select or to find appropriate measurable features. This paper proposes a method to normalize data in MIDI files by 12 dimensional vector descriptors extracted from tonality as well as a novel technique for dimensionality reduction and visualization of extracted music data by 3D projections is discussed. Employing a non-overlapping sliding window through the composition, the harmonic features are found in a music piece. Then a self-similarity matrix is computed using distance metrics to analyze and project the resulting 3D feature vectors. Three dimensional projection creates a quantitative profile of a composition, which correlates the tone similarities along the music piece. The dimensionality reduction is compared with well-known autoencoder. Conducted tests show that our method preserves up to 90% of original data in the projection of reduced dimension. The advantages of the proposed method consist in a novel technique that provides interactive visualization and dynamically adjusts different metrics to observe the behavior of data during music information retrieval and recognition.
更多查看译文
关键词
High dimensional visualization,music composition profile,music data analysis,spherical projection
AI 理解论文
溯源树
样例
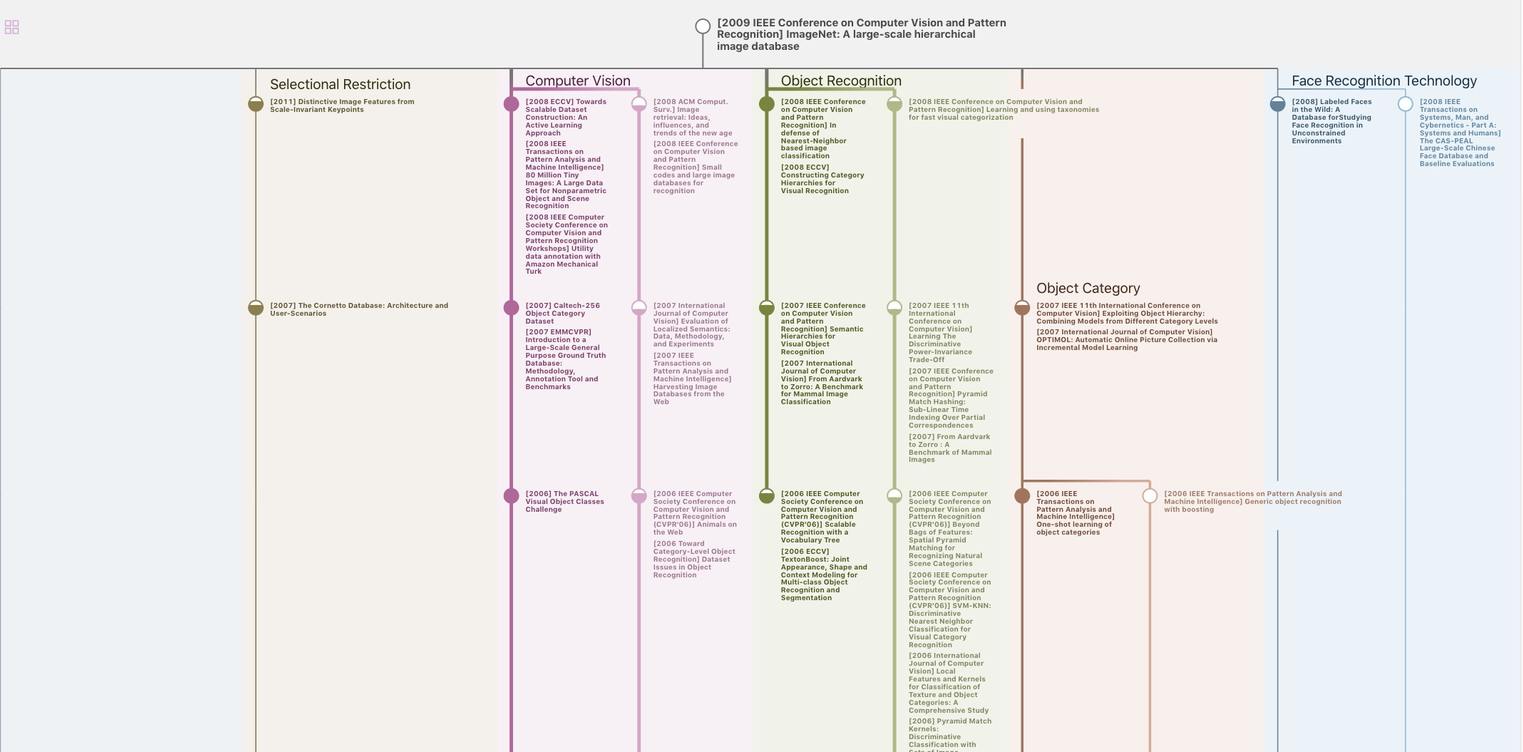
生成溯源树,研究论文发展脉络
Chat Paper
正在生成论文摘要