Autonomous Driving Using Safe Reinforcement Learning By Incorporating A Regret-Based Human Lane-Changing Decision Model
2020 AMERICAN CONTROL CONFERENCE (ACC)(2020)
摘要
It is expected that human-driven vehicles and autonomous vehicles (AVs) will coexist in a mixed traffic for a long time. To enable AVs to safely and efficiently maneuver in this mixed traffic, it is critical that the AVs can understand how humans cope with risks and make driving-related decisions. In this work, we incorporate a human decision-making model in reinforcement learning to control AVs for safe and efficient operations. Specifically, we adapt regret theory to describe a human driver's lane-changing behavior and fit the personalized models to individual drivers for predicting their lane-changing decisions. The predicted decisions are incorporated in the safety regulations for reinforcement learning in training and in implementation. We then use an extended version of double deep Q-network (DDQN) to train our AV controller within the safety set. By doing so, the number of collisions in training and testing is reduced to zero, while the training accuracy is not impinged.
更多查看译文
关键词
Safe Reinforcement Learning, Human Lane-changing Decisions, Regret Theory, DDQN
AI 理解论文
溯源树
样例
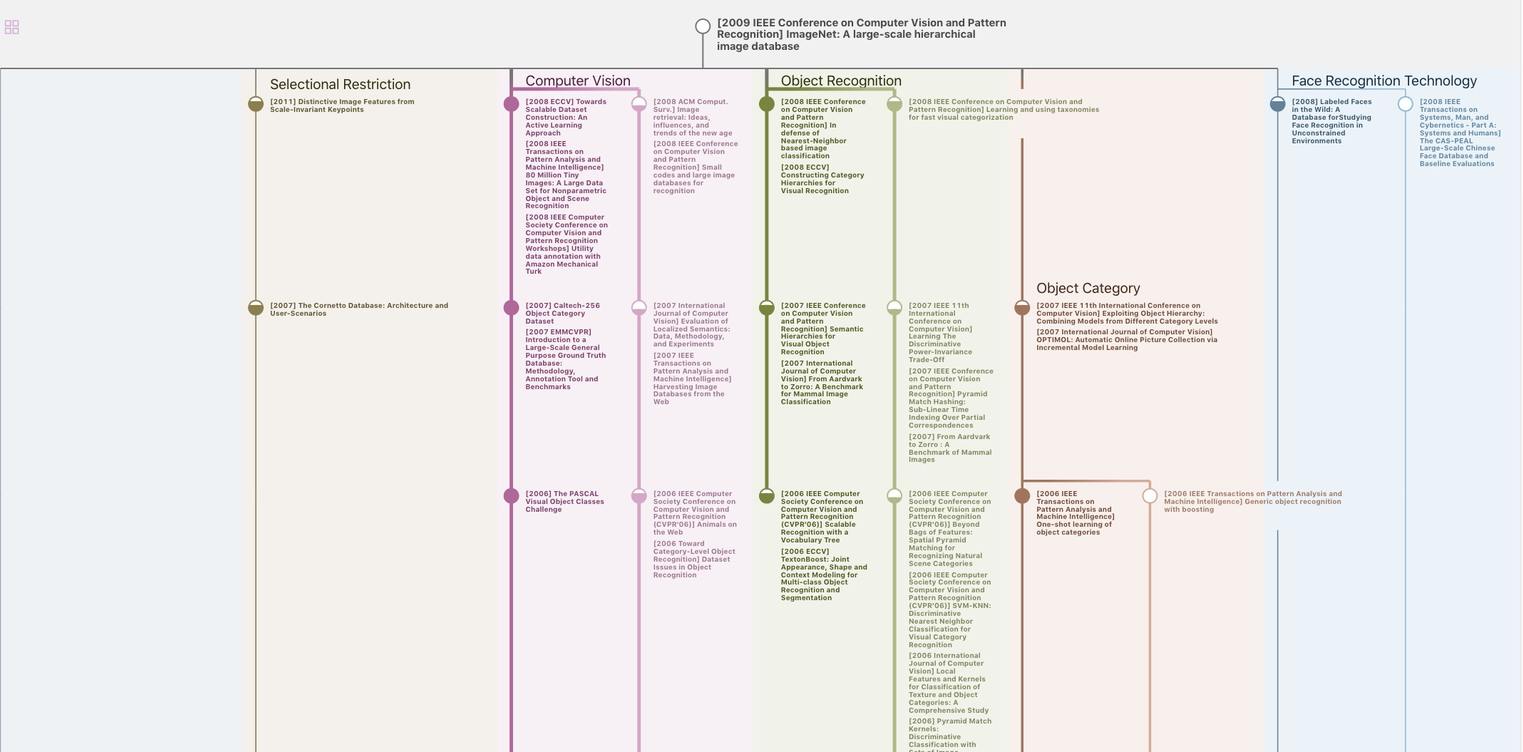
生成溯源树,研究论文发展脉络
Chat Paper
正在生成论文摘要