A Novel Hyperspectral Image Classification Pattern Using Random Patches Convolution And Local Covariance
REMOTE SENSING(2019)
Abstract
Today, more and more deep learning frameworks are being applied to hyperspectral image classification tasks and have achieved great results. However, such approaches are still hampered by long training times. Traditional spectral-spatial hyperspectral image classification only utilizes spectral features at the pixel level, without considering the correlation between local spectral signatures. Our article has tested a novel hyperspectral image classification pattern, using random-patches convolution and local covariance (RPCC). The RPCC is an effective two-branch method that, on the one hand, obtains a specified number of convolution kernels from the image space through a random strategy and, on the other hand, constructs a covariance matrix between different spectral bands by clustering local neighboring pixels. In our method, the spatial features come from multi-scale and multi-level convolutional layers. The spectral features represent the correlations between different bands. We use the support vector machine as well as spectral and spatial fusion matrices to obtain classification results. Through experiments, RPCC is tested with five excellent methods on three public data-sets. Quantitative and qualitative evaluation indicators indicate that the accuracy of our RPCC method can match or exceed the current state-of-the-art methods.
MoreTranslated text
Key words
random patches convolution,local covariance,feature extraction,hyperspectral image classification
AI Read Science
Must-Reading Tree
Example
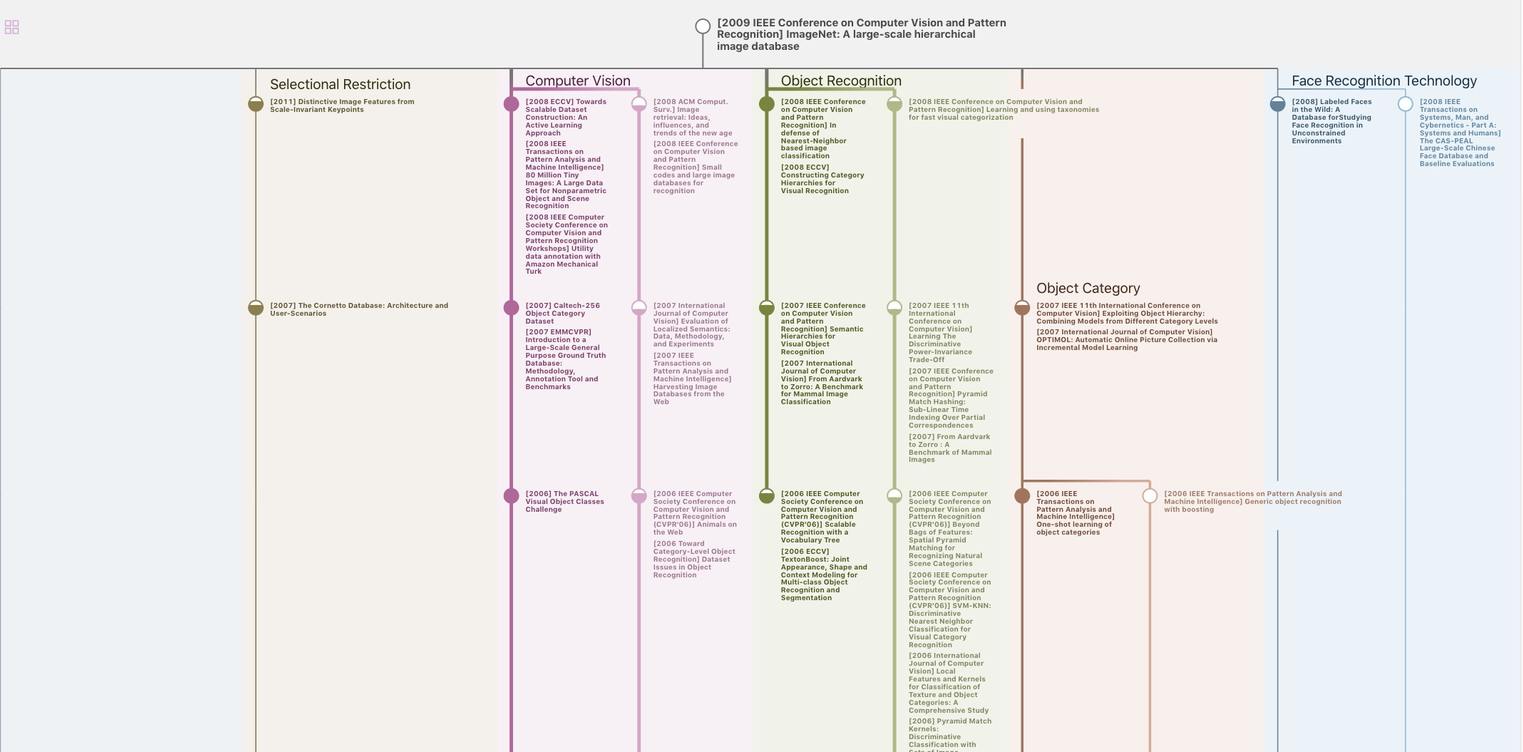
Generate MRT to find the research sequence of this paper
Chat Paper
Summary is being generated by the instructions you defined