RL-Cache: Learning-Based Cache Admission for Content Delivery
IEEE journal on selected areas in communications(2020)
摘要
Content delivery networks (CDNs) distribute much of the Internet content by caching and serving the objects requested by users. A major goal of a CDN is to maximize the hit rates of its caches, thereby enabling faster content downloads to the users. Content caching involves two components: an admission algorithm to decide whether to cache an object and an eviction algorithm to determine which object to evict from the cache when it is full. In this paper, we focus on cache admission and propose a novel algorithm called RL-Cache that uses model-free reinforcement learning (RL) to decide whether or not to admit a requested object into the CDN's cache. Unlike prior approaches that use a small set of criteria for decision making, RL-Cache weights a large set of features that include the object size, recency, and frequency of access. We develop a publicly available implementation of RL-Cache and perform an evaluation using production traces for the image, video, and web traffic classes from Akamai's CDN. The evaluation shows that RL-Cache improves the hit rate in comparison with the state of the art and imposes only a modest resource overhead on the CDN servers. Further, RL-Cache is robust enough that it can be trained in one location and executed on request traces of the same or different traffic classes in other locations of the same geographic region. The paper also reports extensive analyses of the RL-Cache sensitivity to its features and hyperparameter values. The analyses validate the made design choices and reveal interesting insights into the RL-Cache behavior.
更多查看译文
关键词
Servers,Production,Sensitivity,Neural networks,Stochastic processes,Optimization,Machine learning algorithms,Content delivery network,caching,cache admission,hit rate,object feature,neural network,direct policy search,Monte Carlo sampling,stochastic optimization,traffic class,image,video,web,production trace
AI 理解论文
溯源树
样例
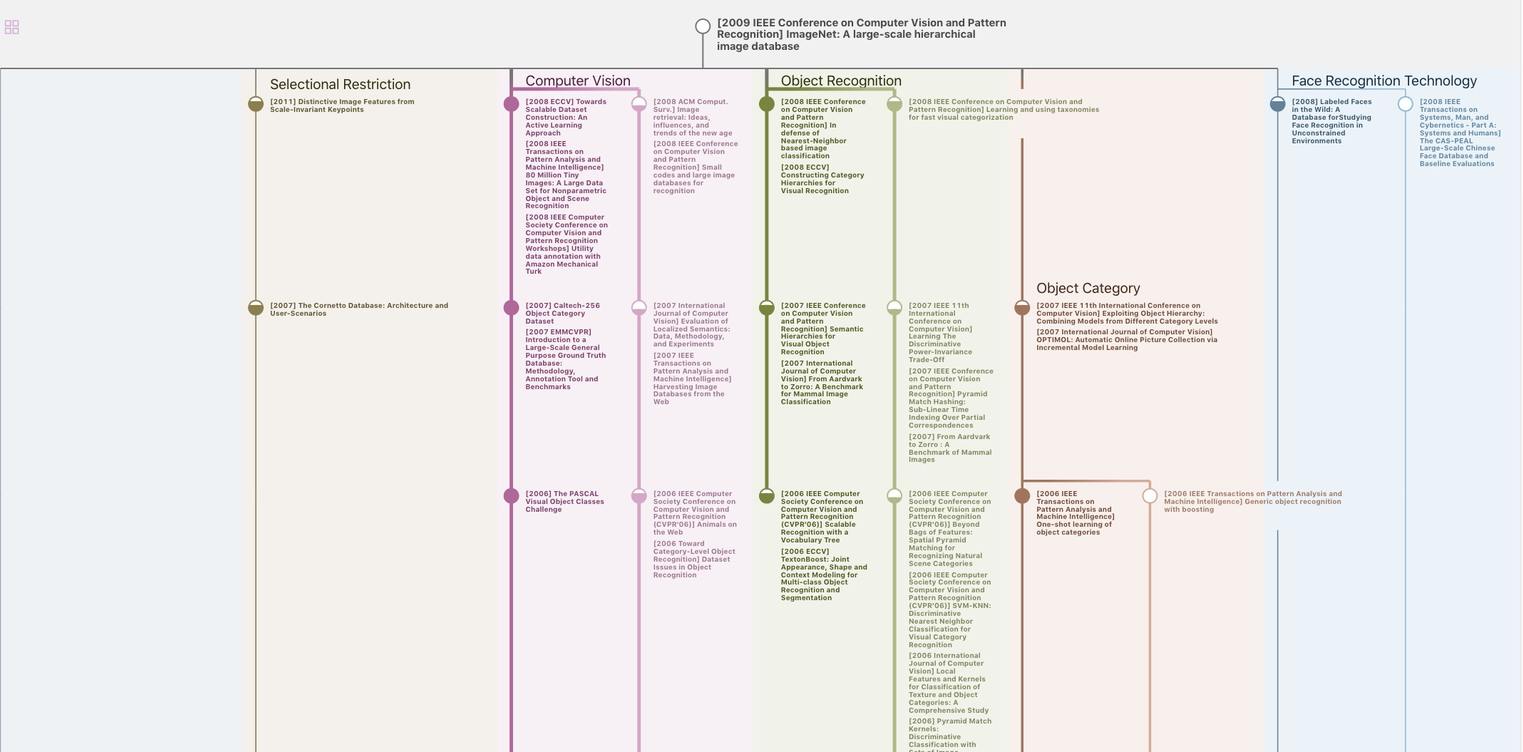
生成溯源树,研究论文发展脉络
Chat Paper
正在生成论文摘要