An exact penalty approach for optimization with nonnegative orthogonality constraints
Math Program(2022)
摘要
Optimization with nonnegative orthogonality constraints has wide applications in machine learning and data sciences. It is NP-hard due to some combinatorial properties of the constraints. We first propose an equivalent optimization formulation with nonnegative and multiple spherical constraints and an additional single nonlinear constraint. Various constraint qualifications, the first- and second-order optimality conditions of the equivalent formulation are discussed. By establishing a local error bound of the feasible set, we design a class of (smooth) exact penalty models via keeping the nonnegative and multiple spherical constraints. The penalty models are exact if the penalty parameter is sufficiently large but finite. A practical penalty algorithm with postprocessing is then developed to approximately solve a series of subproblems with nonnegative and multiple spherical constraints. We study the asymptotic convergence and establish that any limit point is a weakly stationary point of the original problem and becomes a stationary point under some additional mild conditions. Extensive numerical results on the problem of computing the orthogonal projection onto nonnegative orthogonality constraints, the orthogonal nonnegative matrix factorization problems and the K-indicators model show the effectiveness of our proposed approach.
更多查看译文
关键词
Exact penalty, Nonnegative orthogonality constraint, Second-order method, Constraint qualification, Optimality condition
AI 理解论文
溯源树
样例
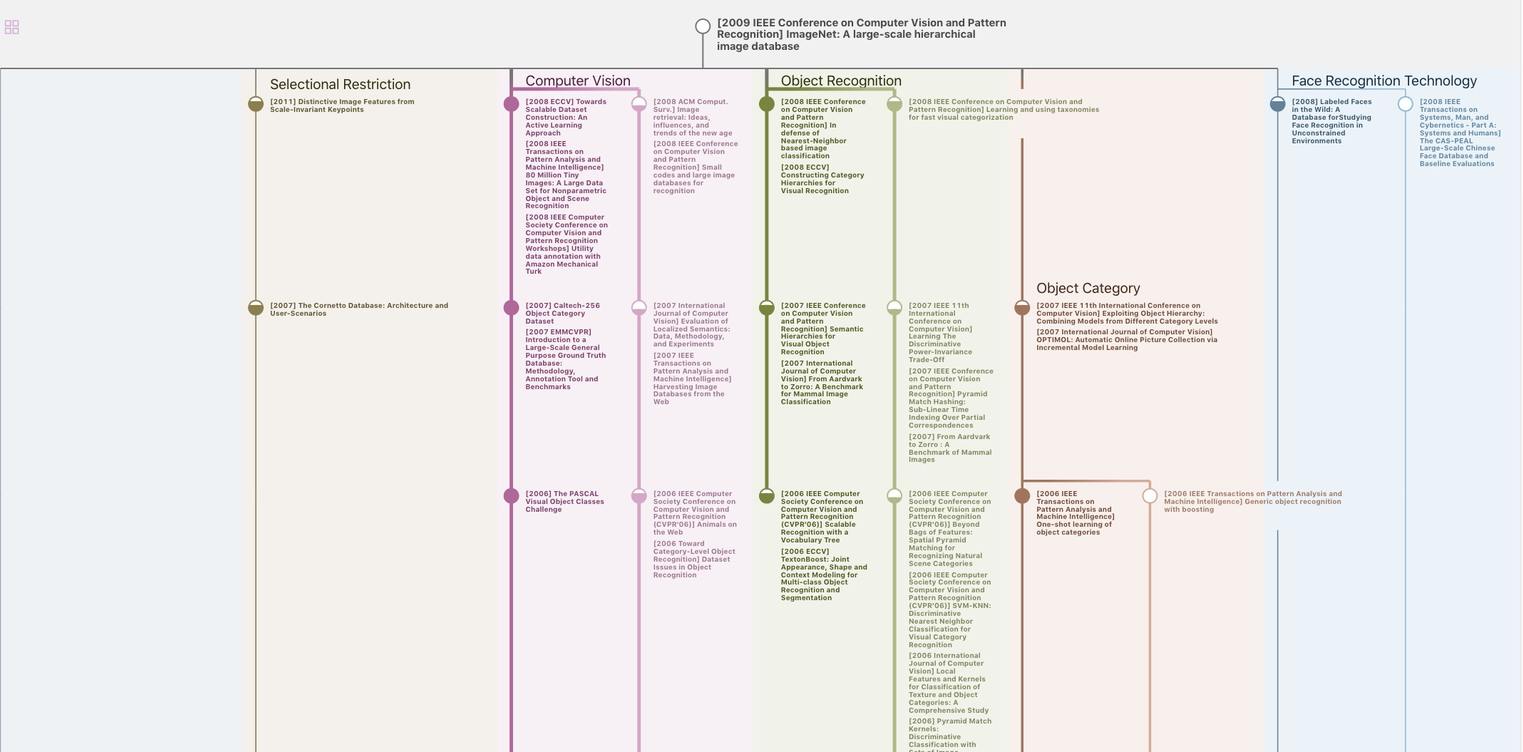
生成溯源树,研究论文发展脉络
Chat Paper
正在生成论文摘要