Nonlinear Variable Selection Algorithms for Surrogate Modeling
AIChE journal(2019)
摘要
Having the ability to analyze, simulate, and optimize complex systems is becoming more important in all engineering disciplines. Decision-making using complex systems usually leads to nonlinear optimization problems, which rely on computationally expensive simulations. Therefore, it is often challenging to detect the actual structure of the optimization problem and formulate these problems with closed-form analytical expressions. Surrogate-based optimization of complex systems is a promising approach that is based on the concept of adaptively fitting and optimizing approximations of the input-output data. Standard surrogate-based optimization assumes the degrees of freedom are known a priori; however, in real applications the sparsity and the actual structure of the black-box formulation may not be known. In this work, we propose to select the correct variables contributing to each objective function and constraints of the black-box problem, by formulating the identification of the true sparsity of the formulation as a nonlinear feature selection problem. We compare three variable selection criteria based on Support Vector Regression and develop efficient algorithms to detect the sparsity of black-box formulations when only a limited amount of deterministic or noisy data is available.
更多查看译文
关键词
nonlinear variable selection,surrogate modeling,support vector regression
AI 理解论文
溯源树
样例
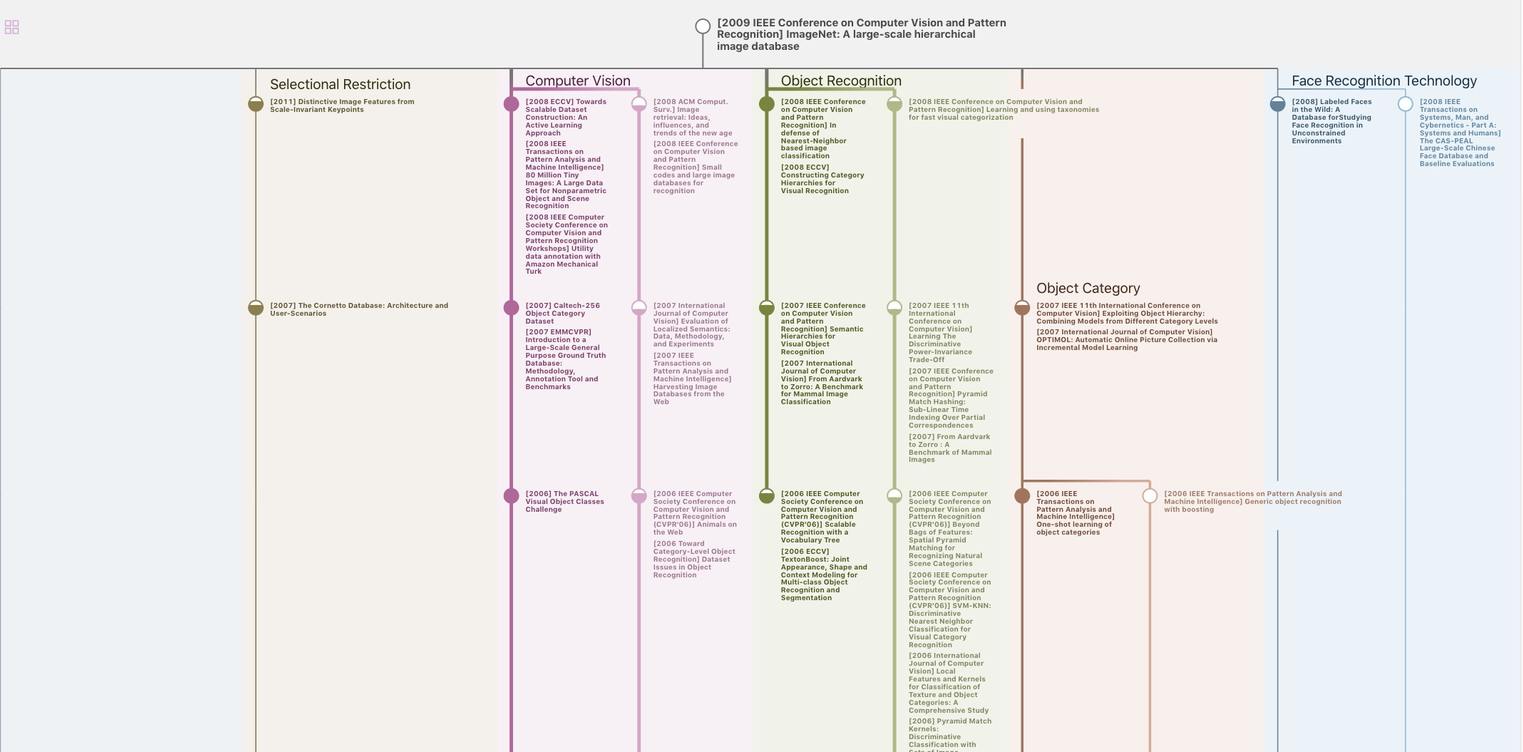
生成溯源树,研究论文发展脉络
Chat Paper
正在生成论文摘要