First-Stage Prostate Cancer Identification On Histopathological Images: Hand-Driven Versus Automatic Learning
ENTROPY(2019)
摘要
Analysis of histopathological image supposes the most reliable procedure to identify prostate cancer. Most studies try to develop computer aid-systems to face the Gleason grading problem. On the contrary, we delve into the discrimination between healthy and cancerous tissues in its earliest stage, only focusing on the information contained in the automatically segmented gland candidates. We propose a hand-driven learning approach, in which we perform an exhaustive hand-crafted feature extraction stage combining in a novel way descriptors of morphology, texture, fractals and contextual information of the candidates under study. Then, we carry out an in-depth statistical analysis to select the most relevant features that constitute the inputs to the optimised machine-learning classifiers. Additionally, we apply for the first time on prostate segmented glands, deep-learning algorithms modifying the popular VGG19 neural network. We fine-tuned the last convolutional block of the architecture to provide the model specific knowledge about the gland images. The hand-driven learning approach, using a nonlinear Support Vector Machine, reports a slight outperforming over the rest of experiments with a final multi-class accuracy of 0.876 +/- 0.026 in the discrimination between false glands (artefacts), benign glands and Gleason grade 3 glands.
更多查看译文
关键词
gland classification,hand-crafted feature extraction,feature selection,hand-driven learning,deep learning,prostate cancer,histological image
AI 理解论文
溯源树
样例
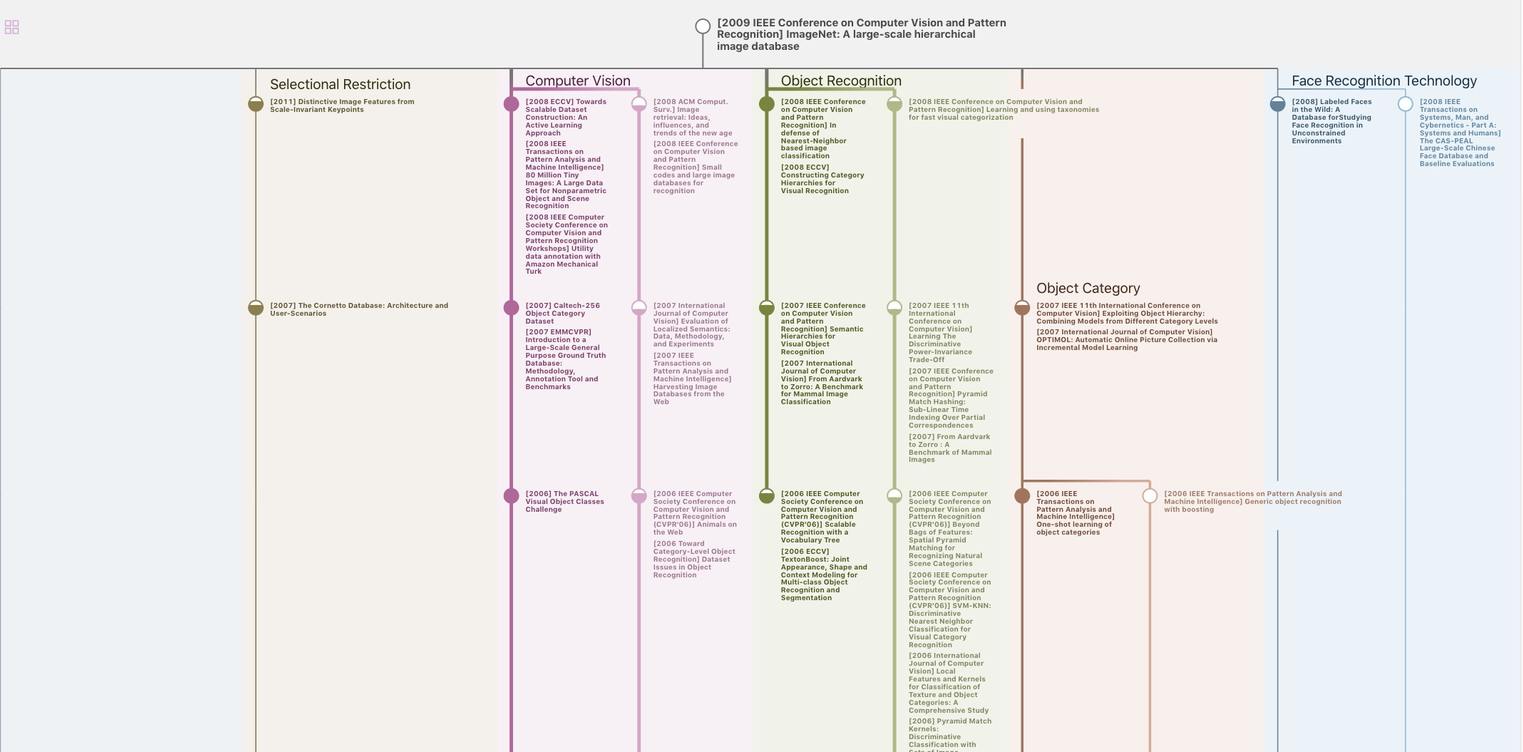
生成溯源树,研究论文发展脉络
Chat Paper
正在生成论文摘要