Communication-Efficient Model Aggregation with Layer Divergence Feedback in Federated Learning
CoRR(2024)
摘要
Federated Learning (FL) facilitates collaborative machine learning by
training models on local datasets, and subsequently aggregating these local
models at a central server. However, the frequent exchange of model parameters
between clients and the central server can result in significant communication
overhead during the FL training process. To solve this problem, this paper
proposes a novel FL framework, the Model Aggregation with Layer Divergence
Feedback mechanism (FedLDF). Specifically, we calculate model divergence
between the local model and the global model from the previous round. Then
through model layer divergence feedback, the distinct layers of each client are
uploaded and the amount of data transferred is reduced effectively. Moreover,
the convergence bound reveals that the access ratio of clients has a positive
correlation with model performance. Simulation results show that our algorithm
uploads local models with reduced communication overhead while upholding a
superior global model performance.
更多查看译文
AI 理解论文
溯源树
样例
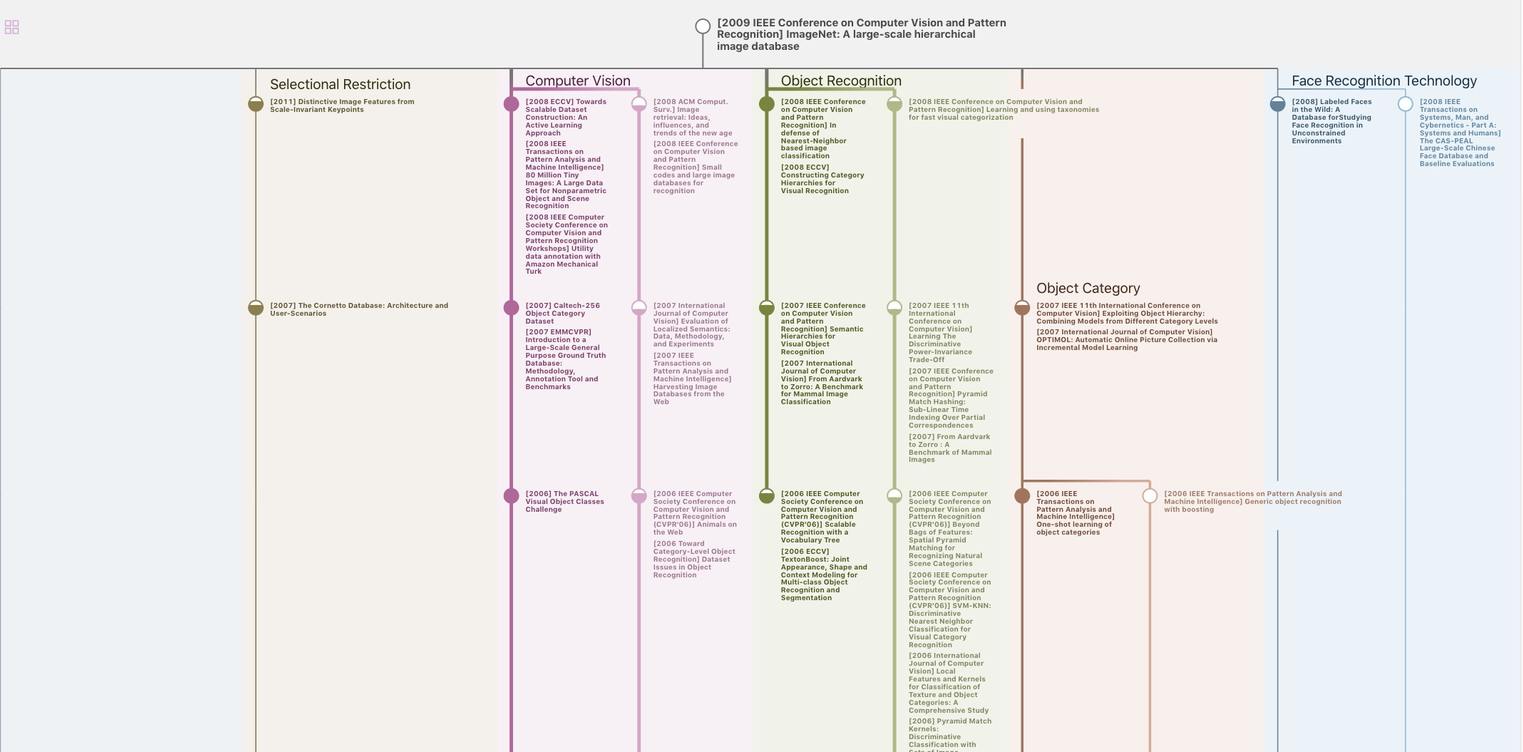
生成溯源树,研究论文发展脉络
Chat Paper
正在生成论文摘要