Fence GAN: Towards Better Anomaly Detection
2019 IEEE 31st International Conference on Tools with Artificial Intelligence (ICTAI)(2019)
摘要
Anomaly detection is a classical problem where the aim is to detect anomalous data that do not belong to the normal data distribution. Current state-of-the-art methods for anomaly detection on complex high-dimensional data are based on the generative adversarial network (GAN). However, the traditional GAN loss is not directly aligned with the anomaly detection objective: it encourages the distribution of the generated samples to overlap with the real data and so the resulting discriminator is ineffective as an anomaly detector. In this paper, we propose modifications to the GAN loss such that the generated samples lie at the boundaries of the real data distribution. With our modified GAN loss, our anomaly detection method, called Fence GAN (FGAN), directly uses the discriminator score as an anomaly threshold. Our experimental results on the MNIST, CIFAR10 and KDD99 datasets show that FGAN yields the best anomaly classification accuracy compared to state-of-the-art methods.
更多查看译文
关键词
Generative Adversarial Network, Anomaly Detection
AI 理解论文
溯源树
样例
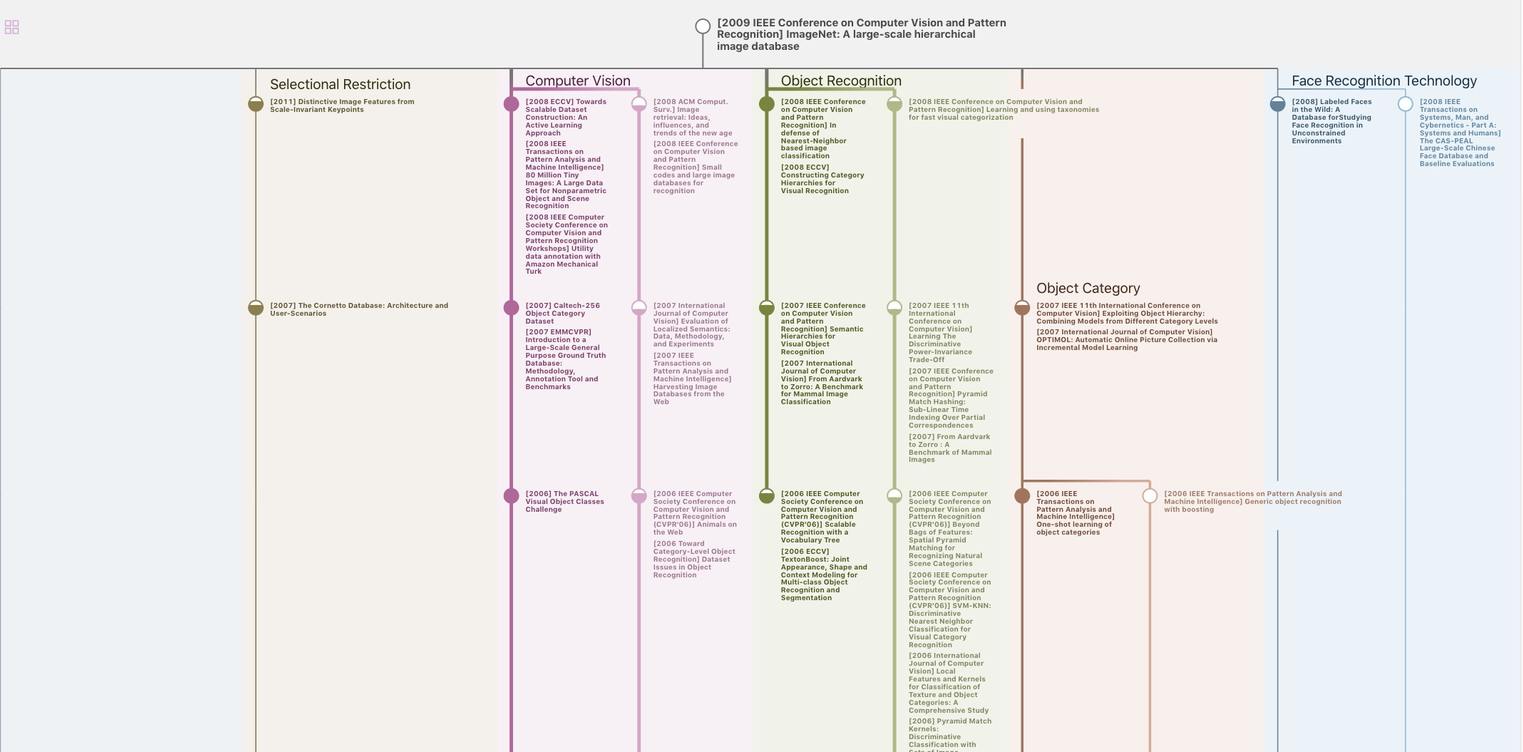
生成溯源树,研究论文发展脉络
Chat Paper
正在生成论文摘要