Modelling complex perception-action choices
Journal of Vision(2018)
摘要
In many contexts, such as reading and sandwich making, the function of gaze is readily interpretable. However, in other contexts (e.g., game playing) the underlying task structure has no ready interpretation. The development of Convolutional Neural Nets (CNNs) has led to breakthroughs in models of human pattern recognition. Here we show their potential for predicting the linkage between gaze and action choices as a way of revealing the underlying task structure in complex behavior. We collected records of actions and gaze using an EyeLink 1000 eye tracker, while participants played eight Atari games in the Arcade Learning Environment. This dataset was used to train two deep CNNs, which we refer to as the Gaze Network and the Policy Network. The former is trained on human gaze data and learns to accurately predict observed gaze positions given the image. The Policy network learns to predict actions (such as firing or evasion) given the image and gaze. The result is that incorporating the output of the Gaze Network into the Policy Network significantly improves the accuracy of human action prediction. By incorporating an attention model that extracts the features that are most important for the task, the learning agent is able to better imitate the human demonstrator's behaviors. The visual attention model also enables the agent to learn better policies, resulting in higher game scores than previous networks. Those networks were trained to predict behaviors demonstrated by human experts using image data, but had no overt attention cues. Our results demonstrate that adding the gaze data greatly improves predictions accuracy (up to 16%). Consequently, the approach explored here has potential for modeling complex visually guided behavior and discovering the underlying task structure. Meeting abstract presented at VSS 2018
更多查看译文
关键词
modelling,complex,perception-action
AI 理解论文
溯源树
样例
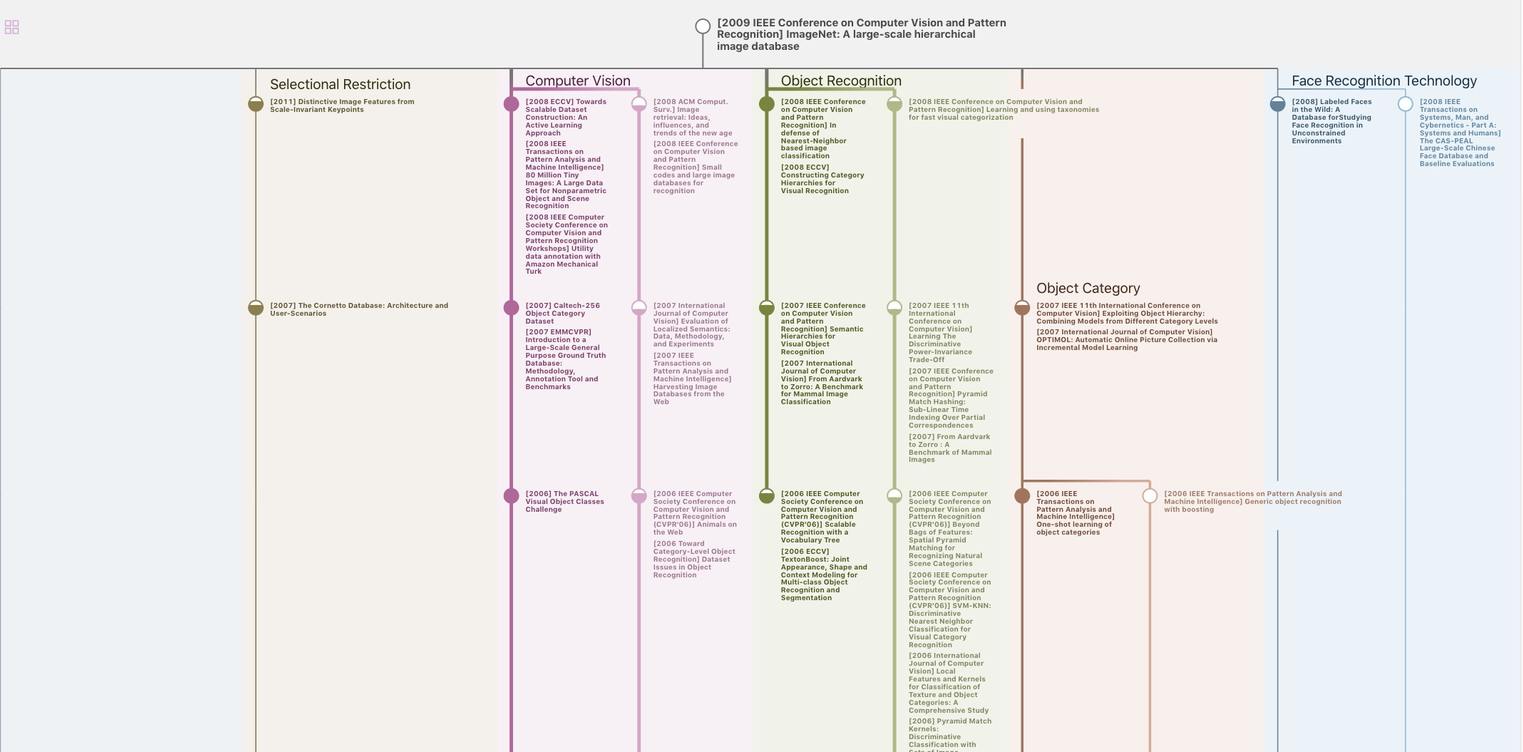
生成溯源树,研究论文发展脉络
Chat Paper
正在生成论文摘要