Two Level Disambiguation Model for Query Translation
International Journal of Electrical and Computer Engineering(2018)
Abstract
Easy availability of machine readable dictionaries have made them first choice for cross language query translation. But selecting the most suitable translation among all translation candidates returned by bilingual dictionary has always been quiet challenging. Researchers have frequently tried to use word co-occurrence statistics to determine the most probable translation for user query. Algorithms using such statistics have certain shortcomings, which are focused in this paper. We propose a novel method for ambiguity resolution, named ‘two level disambiguation model’. At first level disambiguation, the model properly weighs the importance of translation alternatives of query terms obtained from the dictionary. The importance factor measures the probability of a translation candidate of being selected as the final translation of a query term. This removes the problem of taking binary decision for translation candidates. At second level disambiguation, the model targets the user query as a single concept and deduces the translation of all query terms simultaneously, taking into account the weights of translation alternatives also. This is contrary to previous researches which select translation for each word in source language query independently. The experimental result with English-Hindi cross language information retrieval shows that the proposed two level disambiguation model achieved 79.53% and 83.50% of monolingual translation in terms of mean average precision for short queries and long queries respectively. The model achieves 21.11% and 17.36% improvement for short queries and long queries respectively, compared to greedy disambiguation strategies that also exploit word co-occurrence patterns to find best translation.
MoreTranslated text
Key words
level disambiguation model,translation
AI Read Science
Must-Reading Tree
Example
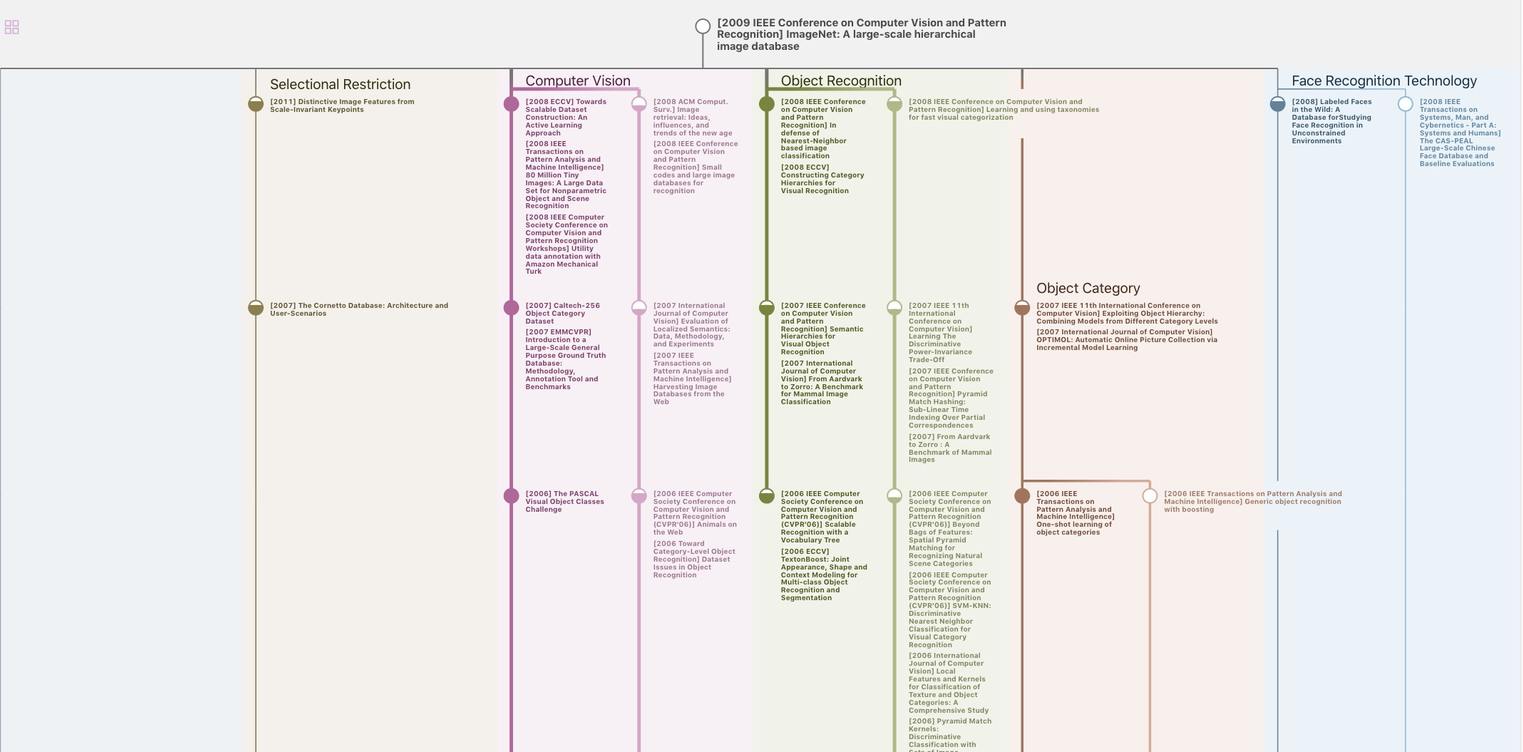
Generate MRT to find the research sequence of this paper
Chat Paper
Summary is being generated by the instructions you defined