Guided Feature Selection for Deep Visual Odometry
COMPUTER VISION - ACCV 2018, PT VI(2018)
摘要
We present a novel end-to-end visual odometry architecture with guided feature selection based on deep convolutional recurrent neural networks. Different from current monocular visual odometry methods, our approach is established on the intuition that features contribute discriminately to different motion patterns. Specifically, we propose a dual-branch recurrent network to learn the rotation and translation separately by leveraging current Convolutional Neural Network (CNN) for feature representation and Recurrent Neural Network (RNN) for image sequence reasoning. To enhance the ability of feature selection, we further introduce an effective context-aware guidance mechanism to force each branch to distill related information for specific motion pattern explicitly. Experiments demonstrate that on the prevalent KITTI and ICL_NUIM benchmarks, our method outperforms current state-of-the-art model- and learning-based methods for both decoupled and joint camera pose recovery.
更多查看译文
关键词
Visual odometry, Recurrent neural networks, Feature selection
AI 理解论文
溯源树
样例
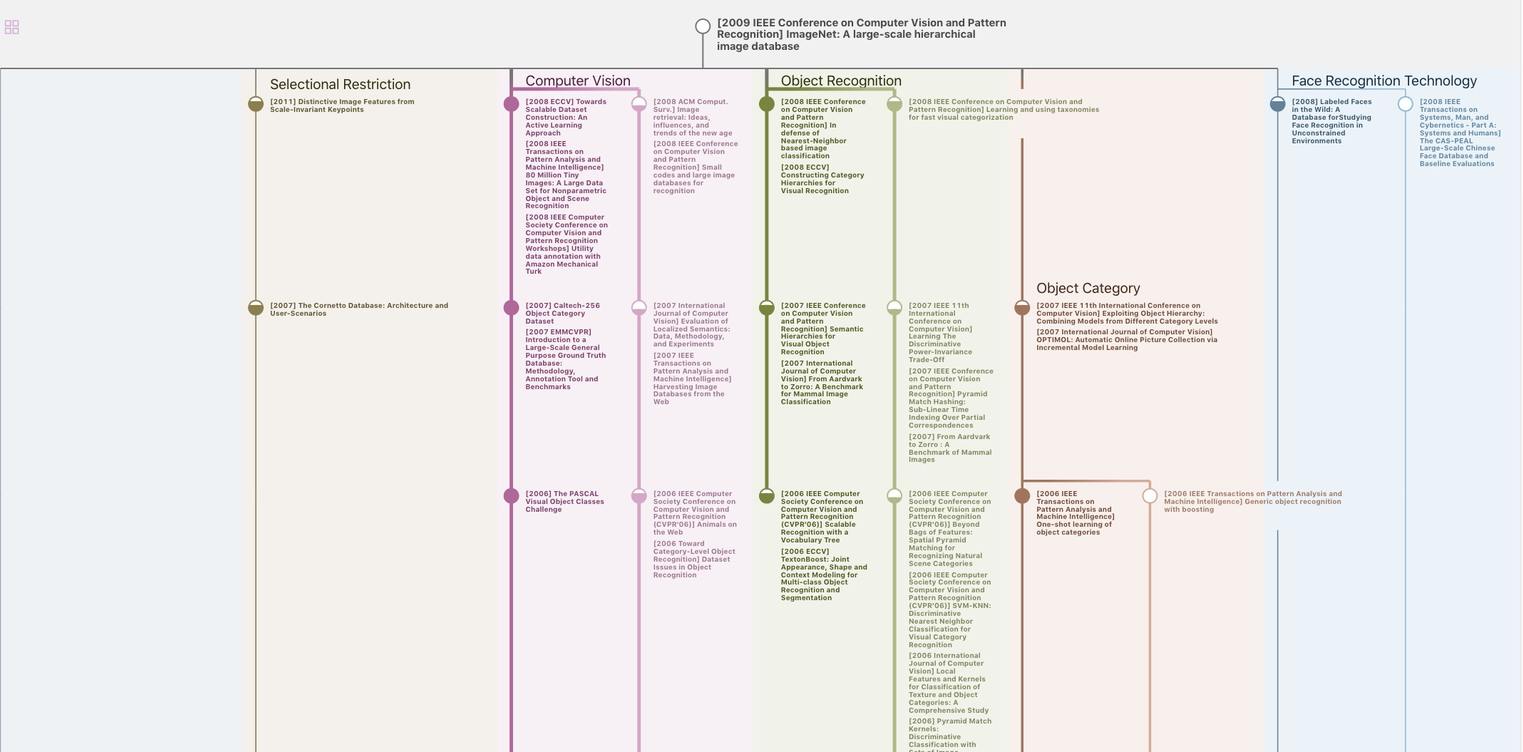
生成溯源树,研究论文发展脉络
Chat Paper
正在生成论文摘要