Sparse Detection For Spatial Modulation In Multiple Access Channels
2018 IEEE SYMPOSIUM ON COMPUTERS AND COMMUNICATIONS (ISCC)(2018)
Abstract
In this paper, a low-complexity detector based on the multi-user sparse Bayesian learning (MSBL) method is proposed for the multi-user spatial modulation (SM) multiple-input-multiple-output (MIMO) system. Firstly, we formulate the multiple access channel SM (MAC-SM) detection as a sparse recovery problem with fixed sparsity constraint. Then, by exploiting the characteristic of the SM transmit signal, we coarsely detect all the potential positions of active antennas. Finally, we select the maximum likely set of the index of active antennas from all user and utilize the zeros-forcing (ZF) estimate to recover the modulation signals. In addition, we theoretically analyze the complexity of proposed algorithm. Experiment and simulation results demonstrate that the proposed detector achieves a good tradeoff between performance and computational complexity.
MoreTranslated text
Key words
Sparse Bayesian Learning, MIMO, Spatial modulation, Multiple access channel
AI Read Science
Must-Reading Tree
Example
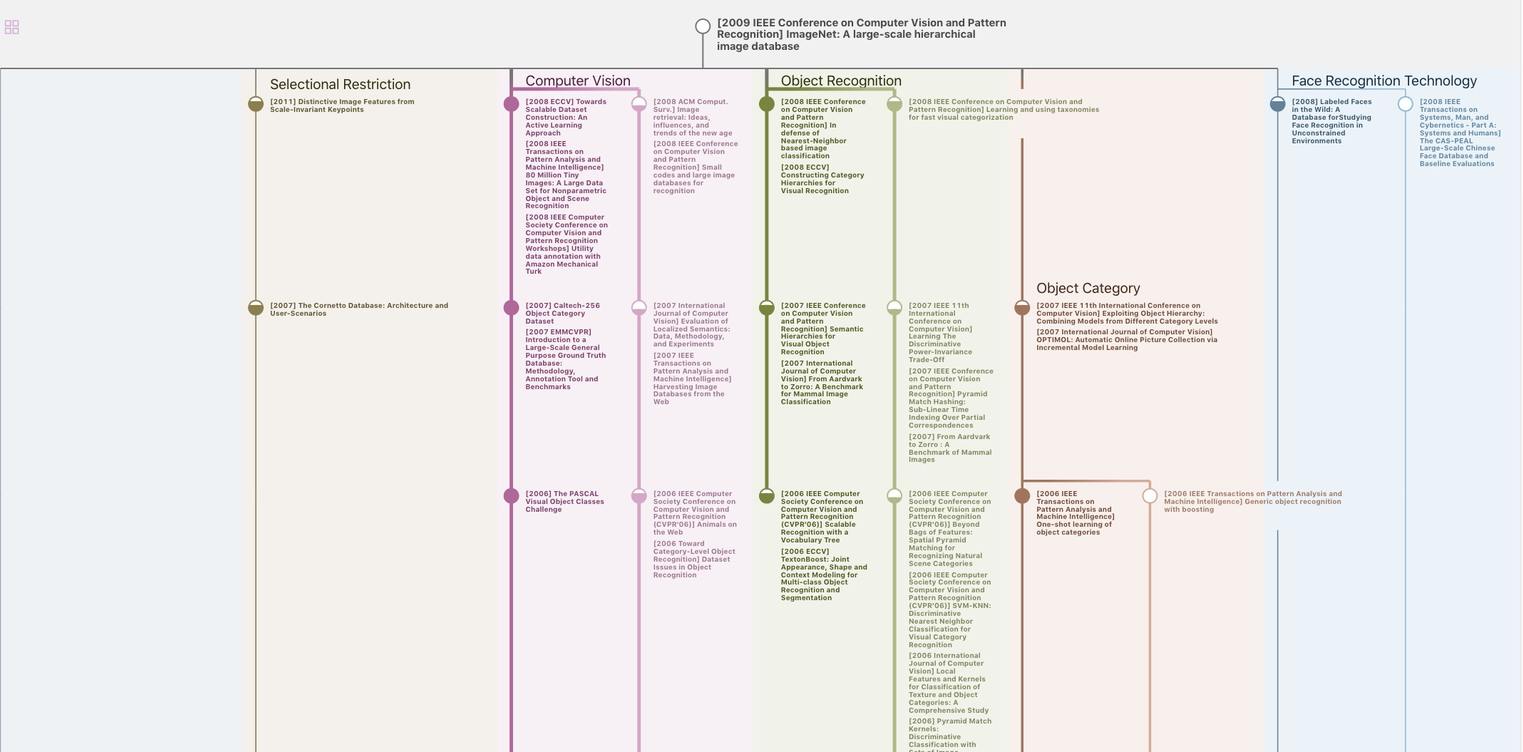
Generate MRT to find the research sequence of this paper
Chat Paper
Summary is being generated by the instructions you defined