E-Embed: A time series visualization framework based on earth mover's distance.
Journal of Visual Languages & Computing(2018)
摘要
Time series analysis is an important topic in machine learning and a suitable visualization method can be used to facilitate the work of data mining. In this paper, we propose E-Embed: a novel framework to visualize time series data by projecting them into a low-dimensional space while capturing the underlying data structure. In the E-Embed framework, we use discrete distributions to model time series and measure the distances between them by using earth mover’s distance (EMD). After the distances between time series are calculated, we can visualize the data by dimensionality reduction algorithms. To combine different dimensionality reduction methods (such as Isomap) that depend on K-nearest neighbor (KNN) graph effectively, we propose an algorithm for constructing a KNN graph based on the earth mover’s distance. We evaluate our visualization framework on both univariate time series data and multivariate time series data. Experimental results demonstrate that E-Embed can provide high quality visualization with low computational cost.
更多查看译文
关键词
Time series,Visualization,EMD,Dimensionality reduction,Similarity measures
AI 理解论文
溯源树
样例
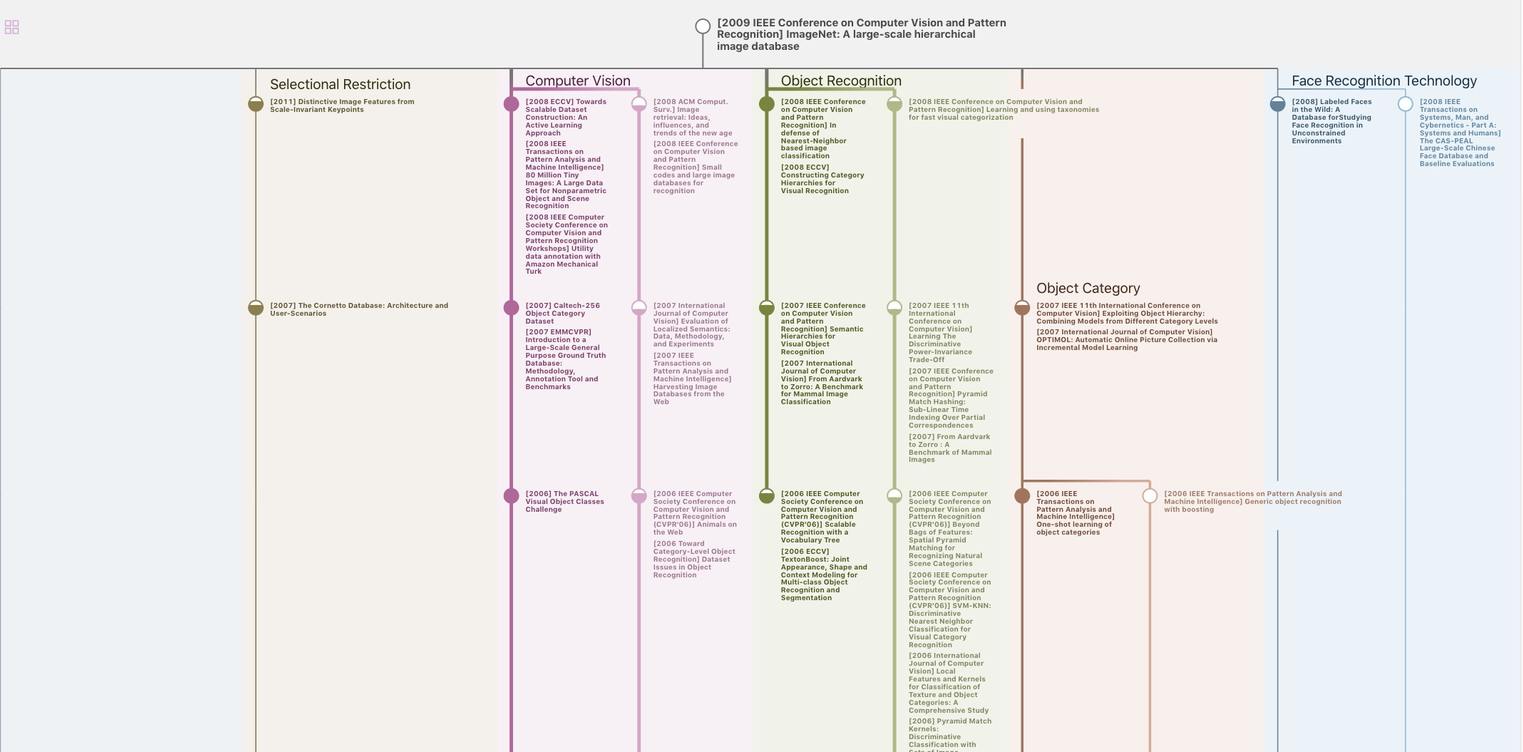
生成溯源树,研究论文发展脉络
Chat Paper
正在生成论文摘要