Sample-Free Learning of Input Grammars for Comprehensive Software Fuzzing
arXiv: Software Engineering(2018)
摘要
Generating valid test inputs for a program is much easier if one knows the input language. We present first successes for a technique that, given a program P without any input samples or models, learns an input grammar that represents the syntactically valid inputs for P – a grammar which can then be used for highly effective test generation for P . To this end, we introduce a test generator targeted at input parsers that systematically explores parsing alternatives based on dynamic tracking of constraints; the resulting inputs go into a grammar learner producing a grammar that can then be used for fuzzing. In our evaluation on subjects such as JSON, URL, or Mathexpr, our PYGMALION prototype took only a few minutes to infer grammars and generate thousands of valid high-quality inputs.
更多查看译文
AI 理解论文
溯源树
样例
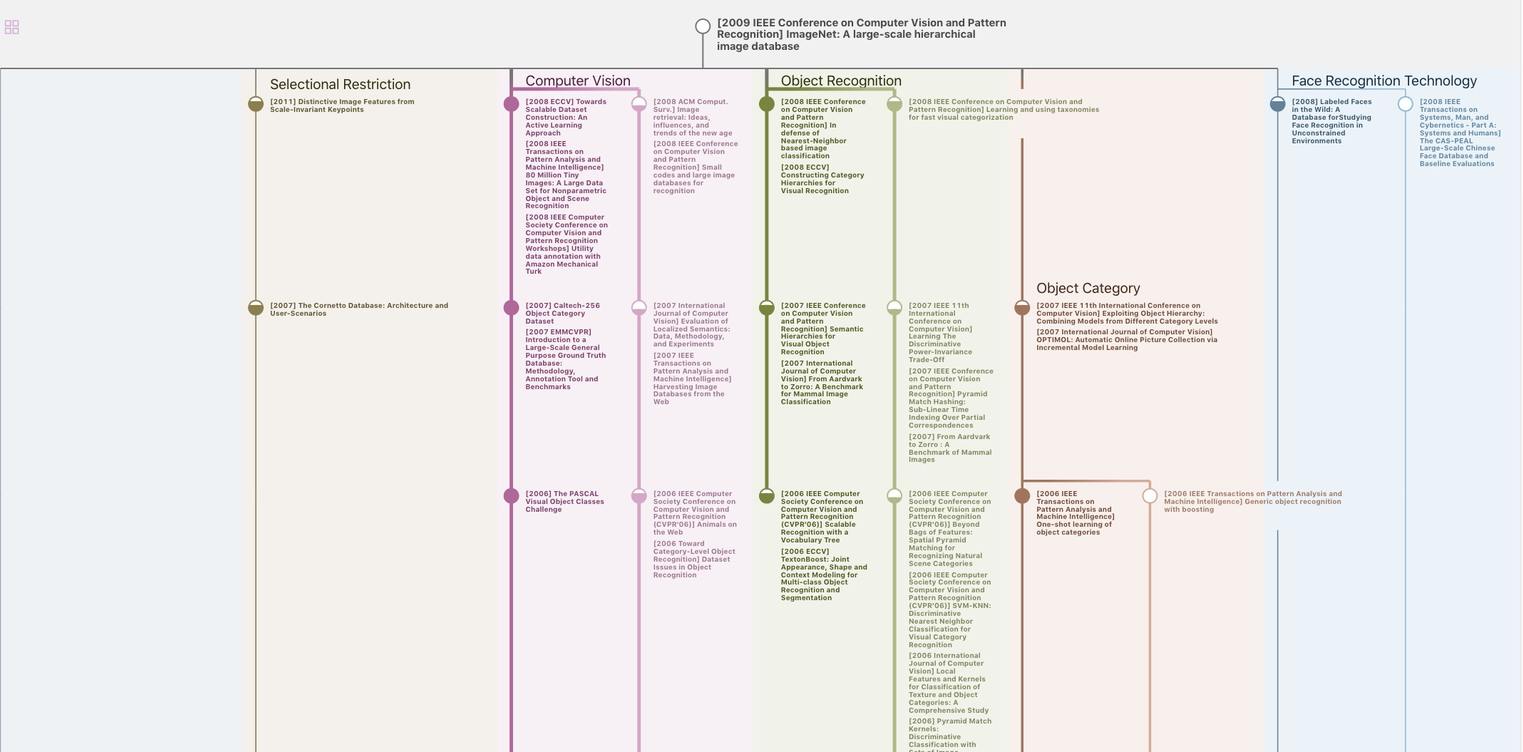
生成溯源树,研究论文发展脉络
Chat Paper
正在生成论文摘要