On Context-Aware DDoS Attacks Using Deep Generative Networks
2018 27th International Conference on Computer Communication and Networks (ICCCN)(2018)
关键词
context-aware DDoS attacks,legitimate flash crowds,traffic characteristics,DDoS detection techniques,deep generative network models,distributed denial of service attacks,Internet,legitimate traffic features
AI 理解论文
溯源树
样例
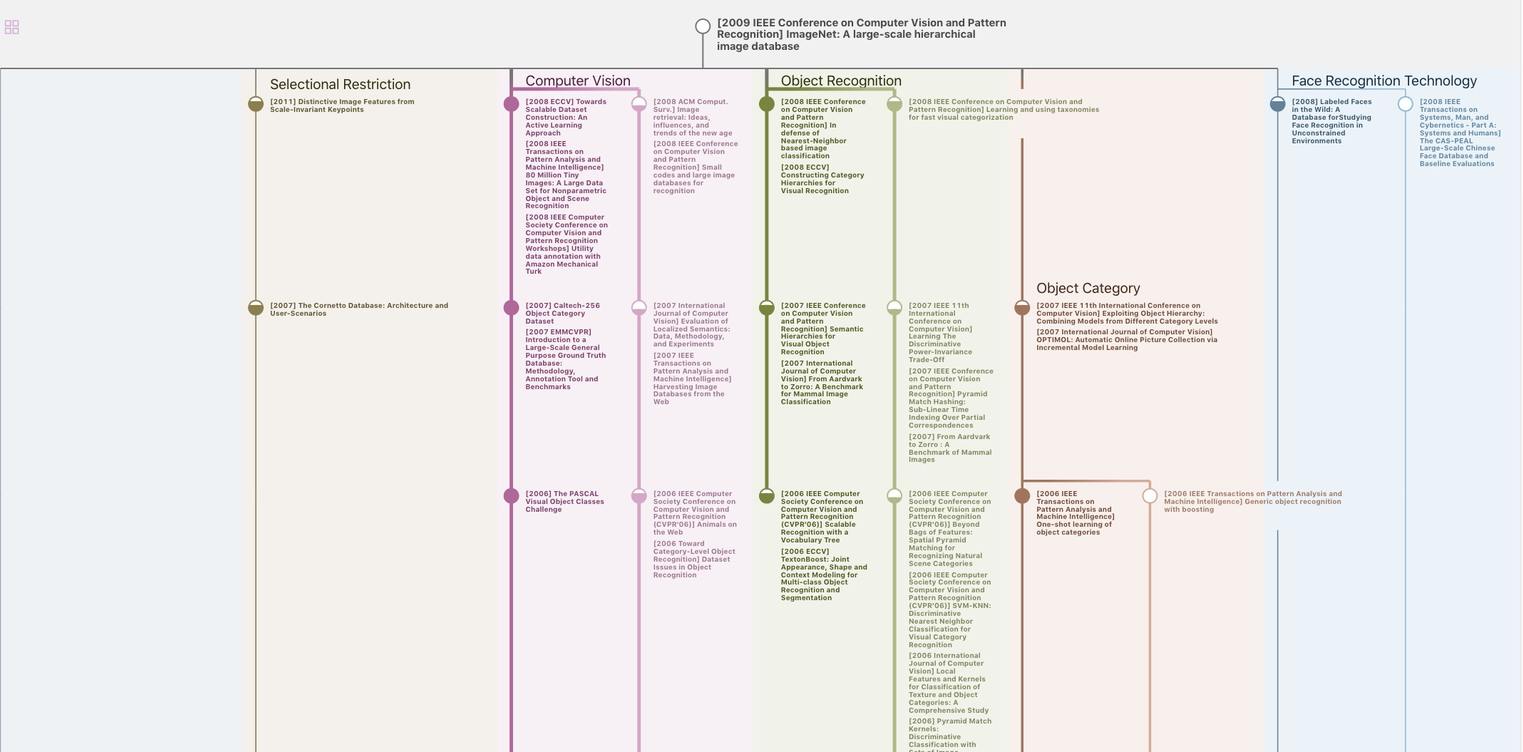
生成溯源树,研究论文发展脉络
Chat Paper
正在生成论文摘要