Making \emph{ordinary least squares} linear classfiers more robust.
arXiv: Data Analysis, Statistics and Probability(2018)
摘要
In the field of statistics and machine learning, the sums-of-squares, commonly referred to as emph{ordinary least squares}, can be used as a convenient choice of cost function because of its many nice analytical properties, though not always the best choice. However, it has been long known that emph{ordinary least squares} is not robust to outliers. Several attempts to resolve this problem led to the creation of alternative methods that, either did not fully resolved the emph{outlier problem} or were computationally difficult. In this paper, we provide a very simple solution that can make emph{ordinary least squares} less sensitive to outliers in data classification, by emph{scaling the augmented input vector by its length}. We show some mathematical expositions of the emph{outlier problem} using some approximations and geometrical techniques. We present numerical results to support the efficacy of our method.
更多查看译文
AI 理解论文
溯源树
样例
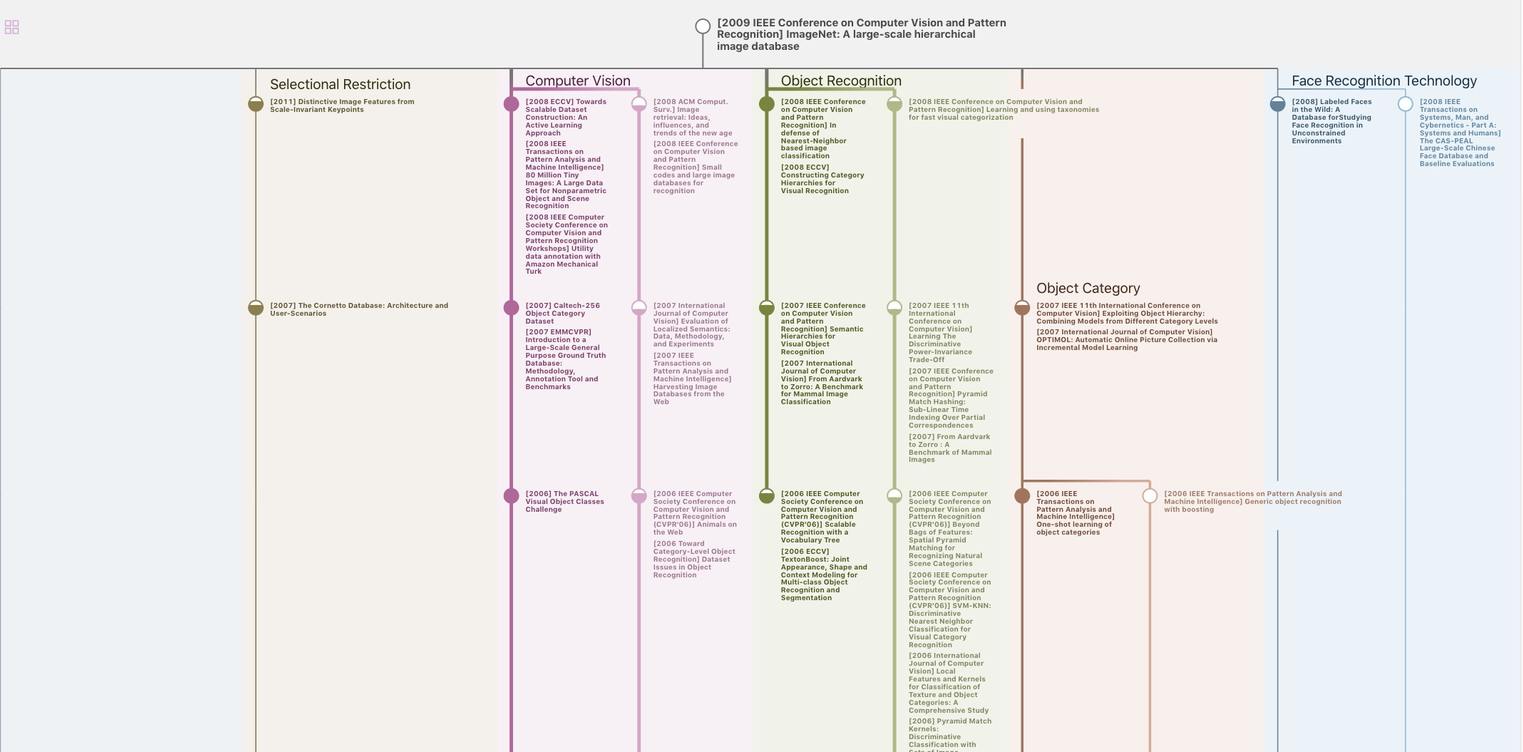
生成溯源树,研究论文发展脉络
Chat Paper
正在生成论文摘要