Multi-sense Based Neural Machine Translation
IJCNN(2017)
摘要
Attention mechanism advances the neural machine translation (NMT) by reducing the confusion introduced by irrelevant words in long sentences. However, the confusion caused by ambiguous words hasn't been handled yet and it may be a bottleneck for the NMT model. This paper validates the hypothesis and proposes a simple and flexible framework, which enables the NMT model to only focus on the relevant sense type of the input word in current context. Experiments show that the proposed model achieves substantial improvements on every test set over competitive baselines. Our contributions come from twofold. Firstly, to the best of our knowledge, this is the first effort to introduce the multi-sense representation, which represents each sense type of the word with a sense-specific embedding, into NMT. Secondly, We propose a sense search module which can detect the sense type of the word automatically. Flexibility and versatility are the most attractive characteristic of the proposed sense search module. It can be applied to any other semantic related NLP tasks with little modification.
更多查看译文
关键词
multisense based neural machine translation,attention mechanism,NMT model,irrelevant words,confusion reduction,ambiguous words,hypothesis validation,multisense representation,sense-specific embedding,sense search module,automatic word sense type detection,semantic related NLP tasks
AI 理解论文
溯源树
样例
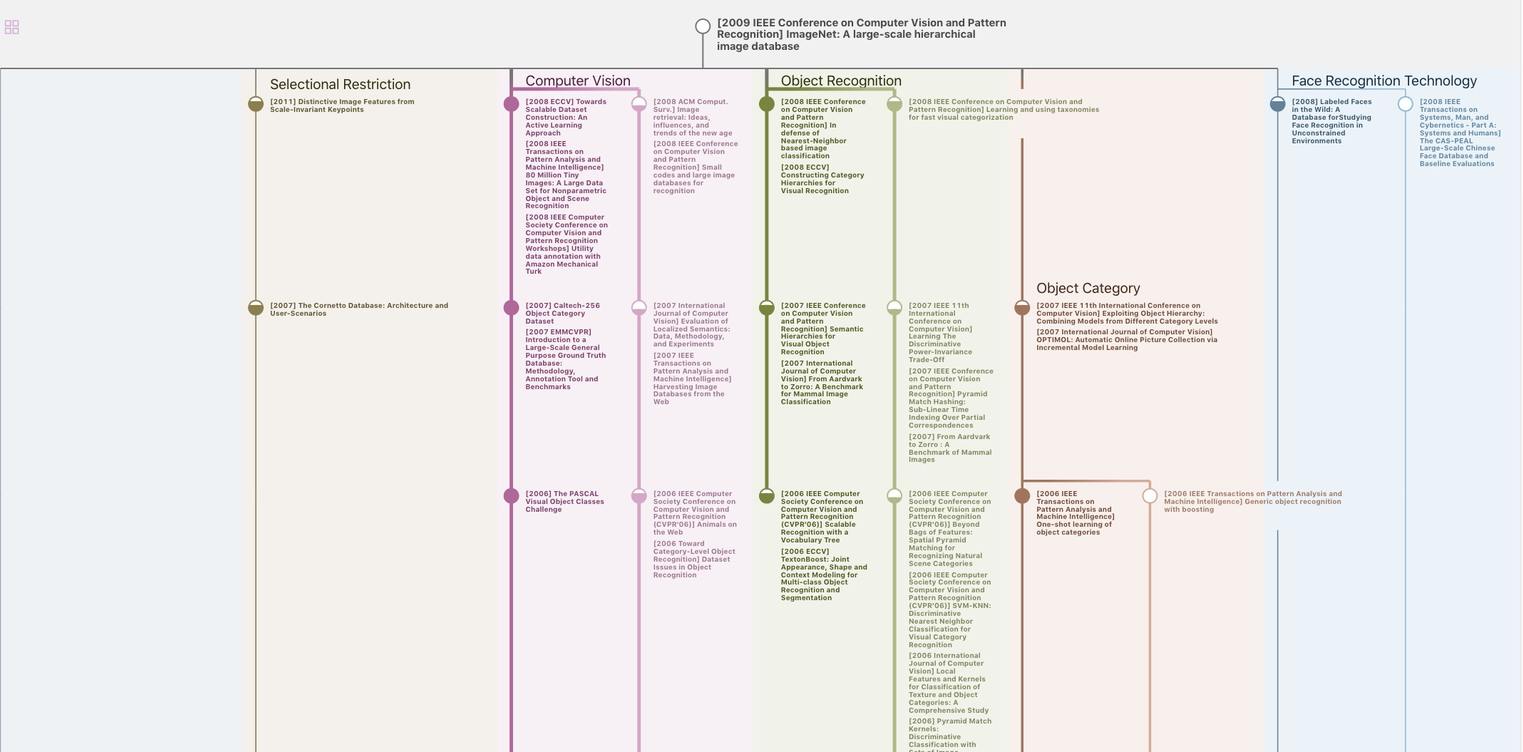
生成溯源树,研究论文发展脉络
Chat Paper
正在生成论文摘要