Unsupervised Language Model Adaptation By Data Selection For Speech Recognition
INTELLIGENT INFORMATION AND DATABASE SYSTEMS, ACIIDS 2017, PT I(2017)
摘要
In this paper, we present a language model (LM) adaptation framework based on data selection to improve the recognition accuracy of automatic speech recognition systems. Previous approaches of LM adaptation usually require additional data to adapt the existing background LM. In this work, we propose a novel two-pass decoding approach that uses no additional data, but instead, selects relevant data from the existing background corpus that is used to train the background LM. The motivation is that the background corpus consists of data from the different domains and as such, the LM trained from it is generic and not discriminative. To make the LM more discriminative, we will select sentences from the background corpus that are similar in some linguistic characteristics to the utterances recognized in the first-pass and use them to train a new LM which is employed during the second-pass decoding. In this work, we examine the use of n-gram and bag-of-words features as linguistic characteristics of selection criteria. Evaluated on the 11 talks in the test-set of TED-LIUM corpus, the proposed adaptation framework produced a LM that reduced the word error rate by up to 10% relatively and the perplexity by up to 47% relatively. When the LM was adapted for each talk individually, further word error rate reduction was achieved.
更多查看译文
关键词
Language model adaptation,Unsupervised adaptation,Data selection,Speech recognition
AI 理解论文
溯源树
样例
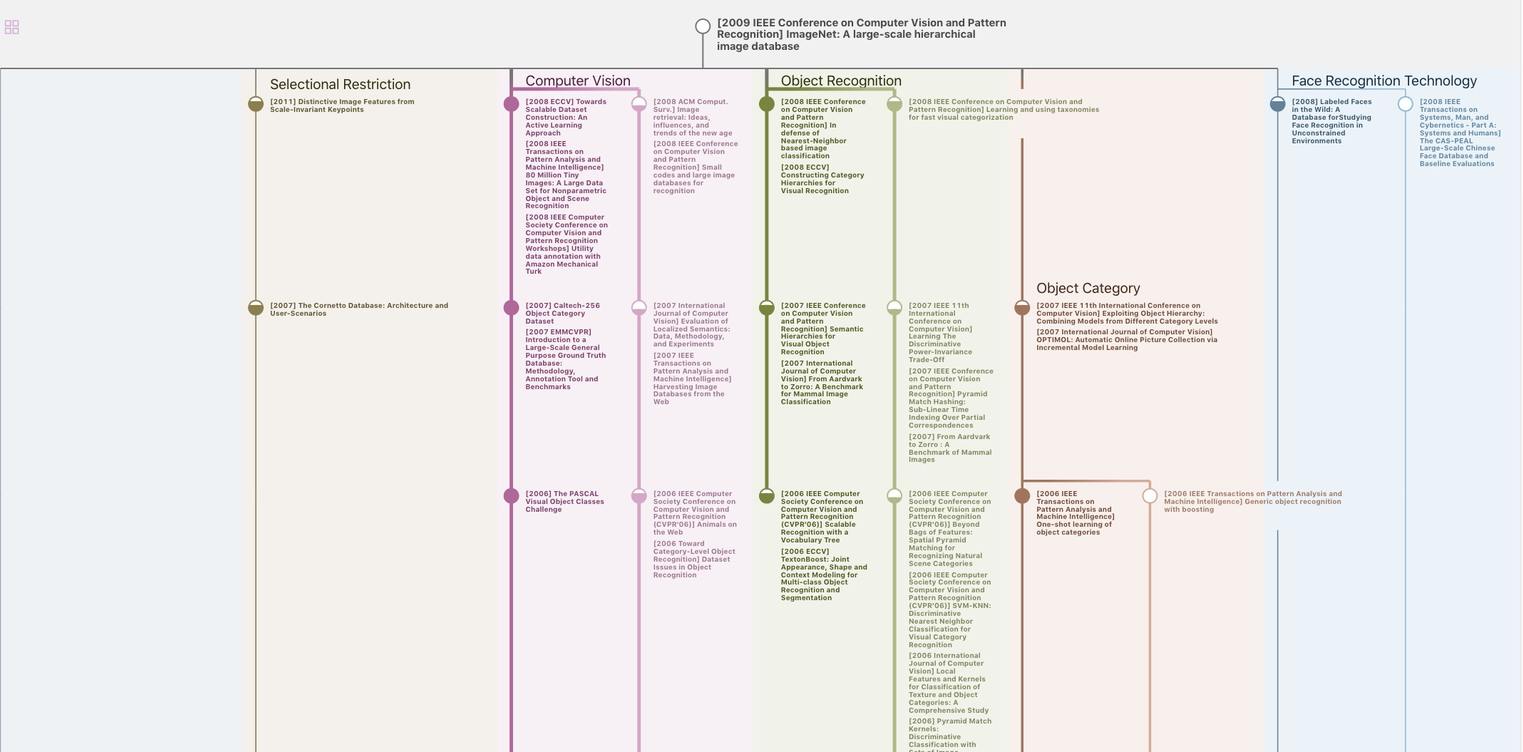
生成溯源树,研究论文发展脉络
Chat Paper
正在生成论文摘要